Drone Net Architecture For Uas Traffic Management Multi-Modal Sensor Networking Experiments
2018 IEEE AEROSPACE CONFERENCE(2018)
Abstract
Drone Net is a conceptual architecture to integrate passive sensor nodes in a local sensor network along with traditional active sensing methods for small Unmanned Aerial System (sUAS) traffic management. The goal of the proposed research architecture is to evaluate the feasibility of the use of multiple passive sensor nodes integrating Electro-Optical/Infrared (EO/IR) and acoustic arrays networked around a UAS Traffic Management (UTM) operating region (Class G uncontrolled airspace for general aviation). The Drone Net approach will be further developed based on the feasibility analysis provided here, to compare to and/or be used in addition to RADAR (Radio Detection and Ranging) and Automatic Dependent Surveillance-Broadcast (ADS-B) tracking and identification in future experiments. We hypothesize that this hybrid passive plus active sensing approach can better manage non-compliant small UAS (without ADS-B transceivers) along with compliant UAS and general aviation in sensitive airspace, urban locations, and geo-fenced regions.Numerous commercial interests are developing UTM instrumentation for compliant and non-compliant drone detection and counter measures, but performance in terms of ability to detect, track, classify (bird, bug, drone, general aviation), identify, and localize aerial objects has not been standardized or well developed to compare multi-sensor solutions. The proposed Drone Net open system reference architecture is designed for passive nodes organized in a network, which can be integrated with RADAR and ADS-B. Here we present preliminary proof of concept results for two primary methods of truth comparison for generation of performance in terms of true and false positives and negatives for detection, classification, and identification. The first ground truth method designed and evaluated uses sUAS Micro Air Vehicle Link (MAVLink) ADS-B data along with EO/IR range detection experiments. The second ground truth method requires human review of triggered detection image capture and allows for truth performance assessment for noncompliant sUAS and other aerial objects (birds and bugs).The networked passive sensors have been designed to meet Class G and geo-fence UTM goals as well as assist with urban UTM operations. The approach can greatly complement NASA UTM collaboration and testing goals for 2020 and the "last fifty foot challenge" for package delivery UAS operations. The EO/IR system has been tested with basic motion detection for general aviation and sUAS in prior work, which is now being extended to include more sensing modalities and more advanced machine vision and machine learning development via the networking of the nodes and ground computing. The paper will detail the hardware, firmware and software architecture, and preliminary efficacy of the two ground truth methods used to compute standard performance metrics.
MoreTranslated text
Key words
Drone net architecture,local sensor network,multiple passive sensor nodes,acoustic arrays,RADAR,Automatic Dependent Surveillance-Broadcast tracking,noncompliant small UAS,ADS-B transceivers,Drone Net open system reference architecture,triggered detection image capture,noncompliant sUAS,networked passive sensors,urban UTM operations,NASA UTM collaboration,package delivery UAS operations,software architecture,UAS Traffic Management,Radio Detection and Ranging,motion detection,machine learning,UAS traffic management multimodal sensor networking,active sensing methods,small Unmanned Aerial System traffic management,EO IR range detection,aerial object detection,aerial object tracking,aerial object classification,aerial object localization,sUAS MicroAir Vehicle Link ADS-B data,machine vision
AI Read Science
Must-Reading Tree
Example
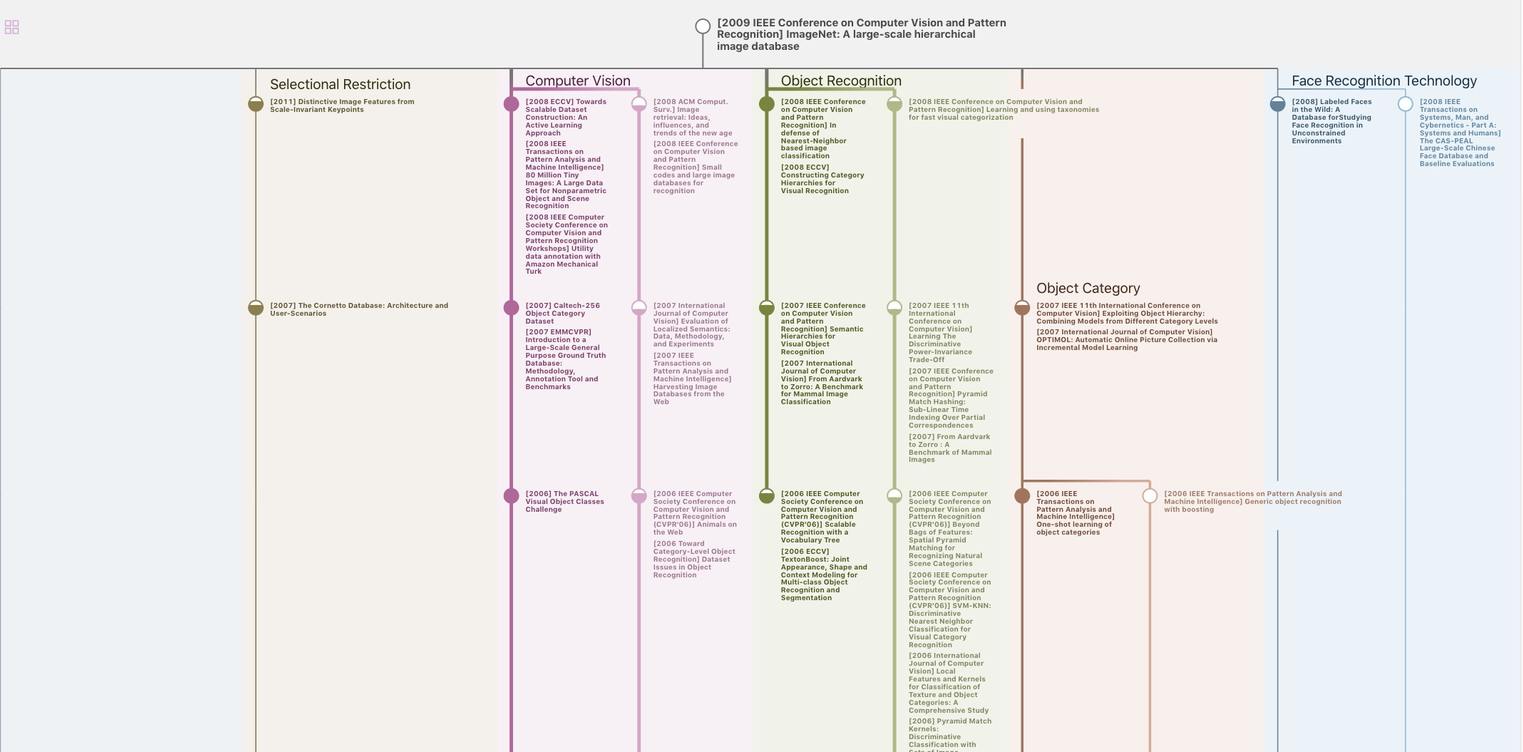
Generate MRT to find the research sequence of this paper
Chat Paper
Summary is being generated by the instructions you defined