Anomaly Classification in Distribution Networks Using a Quotient Gradient System
arXiv: Signal Processing(2018)
摘要
The classification of anomalies or sudden changes in power networks versus normal abrupt changes or switching actions is essential to take appropriate maintenance actions that guarantee the quality of power delivery. This issue has increased in importance and has become more complicated with the proliferation of volatile resources that introduce variability, uncertainty, and intermittency in circuit behavior that can be observed as variations in voltage and current phasors. This makes diagnostics applications more challenging. This paper proposes using quotient gradient system (QGS) to train two-stage partially recurrent neural network to improve anomaly classification rate in power distribution networks using high-fidelity data from micro-phasor measurement units (PMUs). QGS is a systematic approach to finding solutions of constraint satisfaction problems. We transform the PMUs data from the power network into a constraint satisfaction problem and use QGS to train a neural network by solving the resulting optimization problem. Simulation results show that the proposed supervised classification method can reliably distinguish between different anomalies in power distribution networks. Comparison with other neural network classifiers shows that QGS trained networks provide significantly better classification. Sensitivity analysis is performed concerning the number of PMUs, reporting rates, noise level and early versus late data stream fusion frameworks.
更多查看译文
AI 理解论文
溯源树
样例
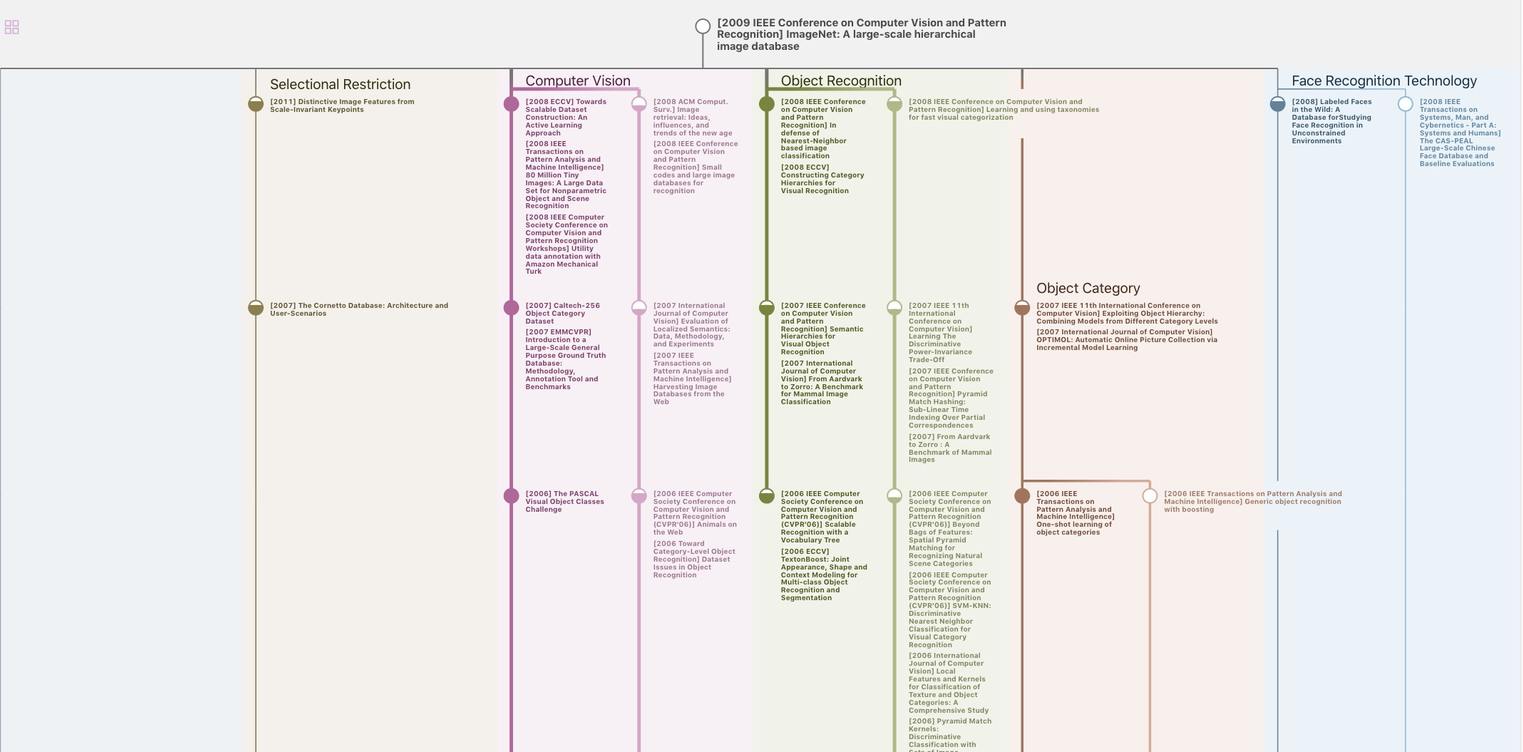
生成溯源树,研究论文发展脉络
Chat Paper
正在生成论文摘要