A bias–variance trade-off governs individual differences in on-line learning in an unpredictable environment
NATURE HUMAN BEHAVIOUR(2018)
摘要
Decisions often benefit from learned expectations about the sequential structure of the evidence. Here we show that individual differences in this learning process can reflect different implicit assumptions about sequence complexity, leading to performance trade-offs. For a task requiring decisions about dynamic evidence streams, human subjects with more flexible, history-dependent choices (low bias) had greater trial-to-trial choice variability (high variance). In contrast, subjects with more history-independent choices (high bias) were more predictable (low variance). We accounted for these behaviours using models in which assumed complexity was encoded by the size of the hypothesis space over the latent rate of change of the source of evidence. The most parsimonious model used an efficient sampling algorithm in which the range of sampled hypotheses represented an information bottleneck that gave rise to a bias–variance trade-off. This trade-off, which is well known in machine learning, may thus also have broad applicability to human decision-making.
更多查看译文
关键词
Decision,Learning algorithms,Life Sciences,general,Behavioral Sciences,Neurosciences,Microeconomics,Personality and Social Psychology,Experimental Psychology
AI 理解论文
溯源树
样例
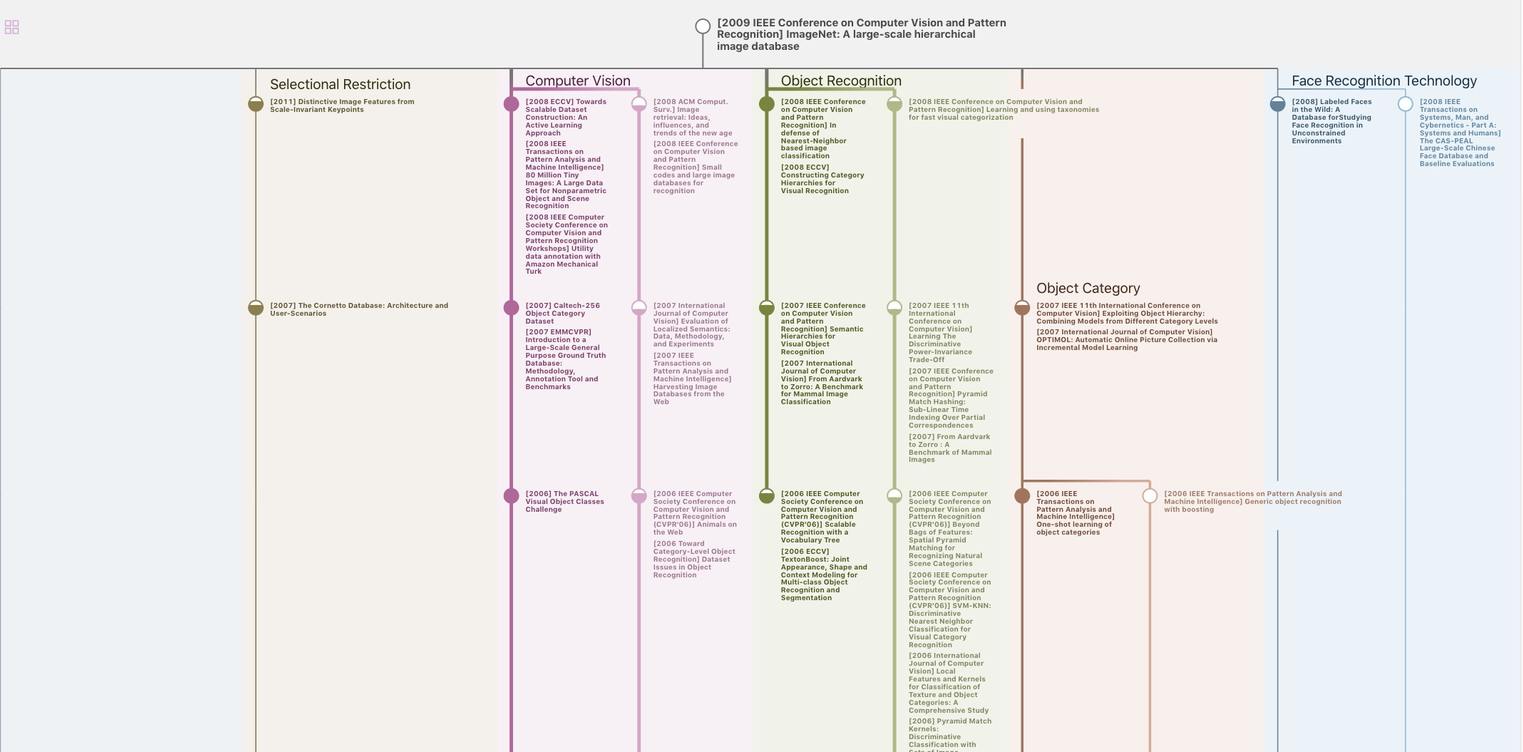
生成溯源树,研究论文发展脉络
Chat Paper
正在生成论文摘要