Central limit theorems in the configuration model
ANNALS OF APPLIED PROBABILITY(2019)
摘要
We prove a general normal approximation theorem for local graph statistics in the configuration model, together with an explicit bound on the error in the approximation with respect to the Wasserstein metric. Such statistics take the form T := Sigma(v is an element of V) H-v, where V is the vertex set, and H-v depends on a neighbourhood in the graph around v of size at most l. The error bound is expressed in terms of l, vertical bar V vertical bar, an almost sure bound on H-v, the maximum vertex degree d(max) and the variance of T. Under suitable assumptions on the convergence of the empirical degree distributions to a limiting distribution, we deduce that the size of the giant component in the configuration model has asymptotically Gaussian fluctuations.
更多查看译文
关键词
Configuration random graph model,giant component,central limit theorem,Stein's method
AI 理解论文
溯源树
样例
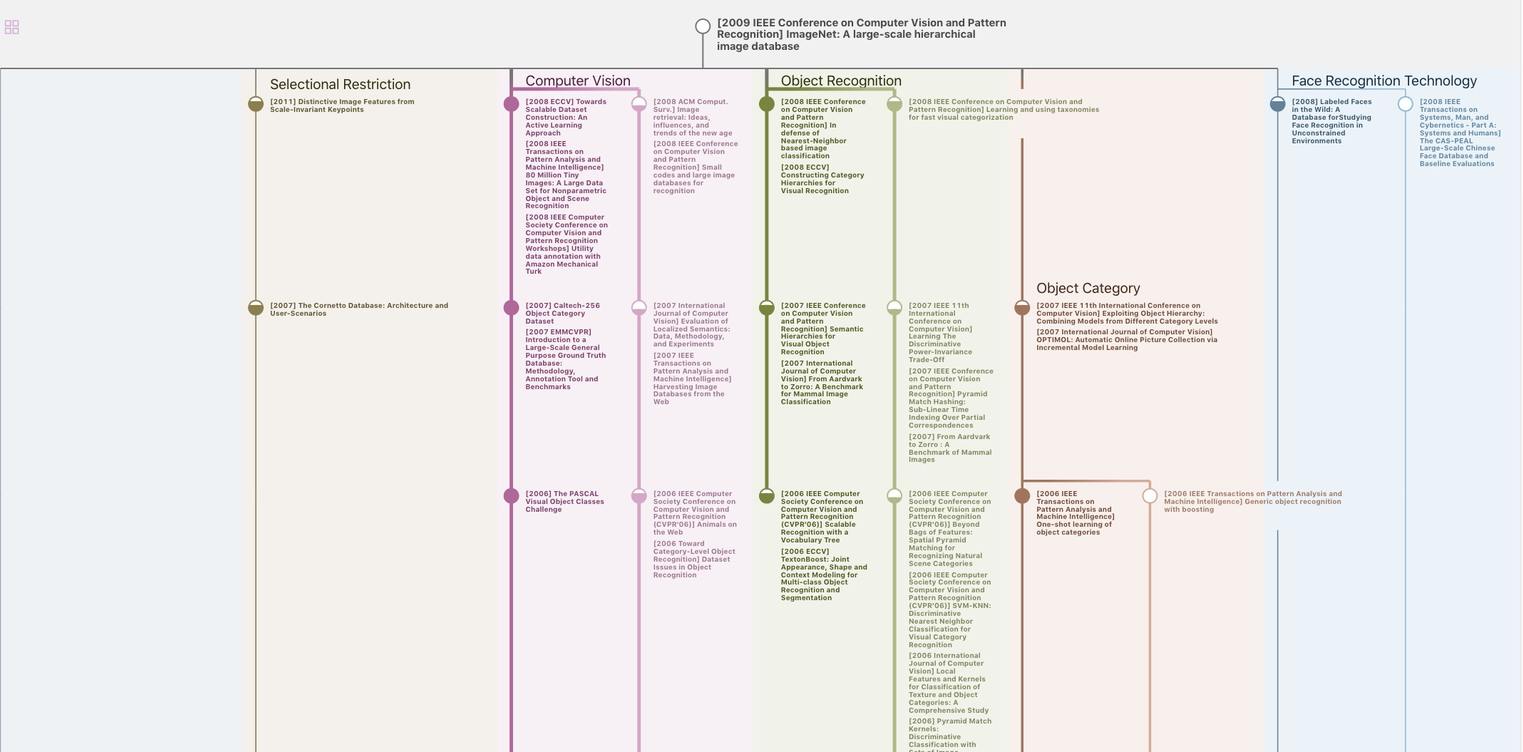
生成溯源树,研究论文发展脉络
Chat Paper
正在生成论文摘要