Enhanced Fault Detection Based on Ensemble Global–Local Preserving Projections with Quantitative Global–Local Structure Analysis
INDUSTRIAL & ENGINEERING CHEMISTRY RESEARCH(2017)
摘要
A novel data-driven fault detection strategy that fully considers the global local structure is proposed. Inevitably, traditional methods concerning the global local structure are unilateral and the global local information is extracted partially. To extract the information fully, an available idea is to combine these methods. Considering the feasibility, the same type of model is applied in this paper. The global local preserving projections (GLPP) model, which is suitable for extracting the global local structure, is used as the base model of the proposed model. The purpose of this paper is to study the combination of the GLPP models by the use of ensemble learning strategy. This idea requires dealing with two main problems: how to choose the GLPP models diversely and how to combine them. To solve these two issues, first, a global index Gper that could analyze the global and local structure quantitatively is proposed and then the diverse GLPP models can be selected according to Gp. The kernel density estimation (KDE) method is used to get more accurate results and control limits can be acquired. Next, Bayesian inference and weighted sum strategies are adopted to combine the selected GLPP models. The weighted sum strategy implements the motivation of fully analyzing the global local structure in a simple and useful way. In particular, the false alarm rate (FAR) of the ensemble one is a little higher since the false alarm information is accumulated as well. To help reduce the FAR of EGLPP (ensemble GLPP) model, an indicator called WFAR that could represent the reliability of the GLPP model is proposed. It is shown that the proposed EGLPP monitoring method could enhance the performance of monitoring, in other words, be more stable to the selection of the global index and significantly improve the detection performance under a relatively low FAR. This is demonstrated by the widely accepted Tennessee Eastman benchmark process.
更多查看译文
AI 理解论文
溯源树
样例
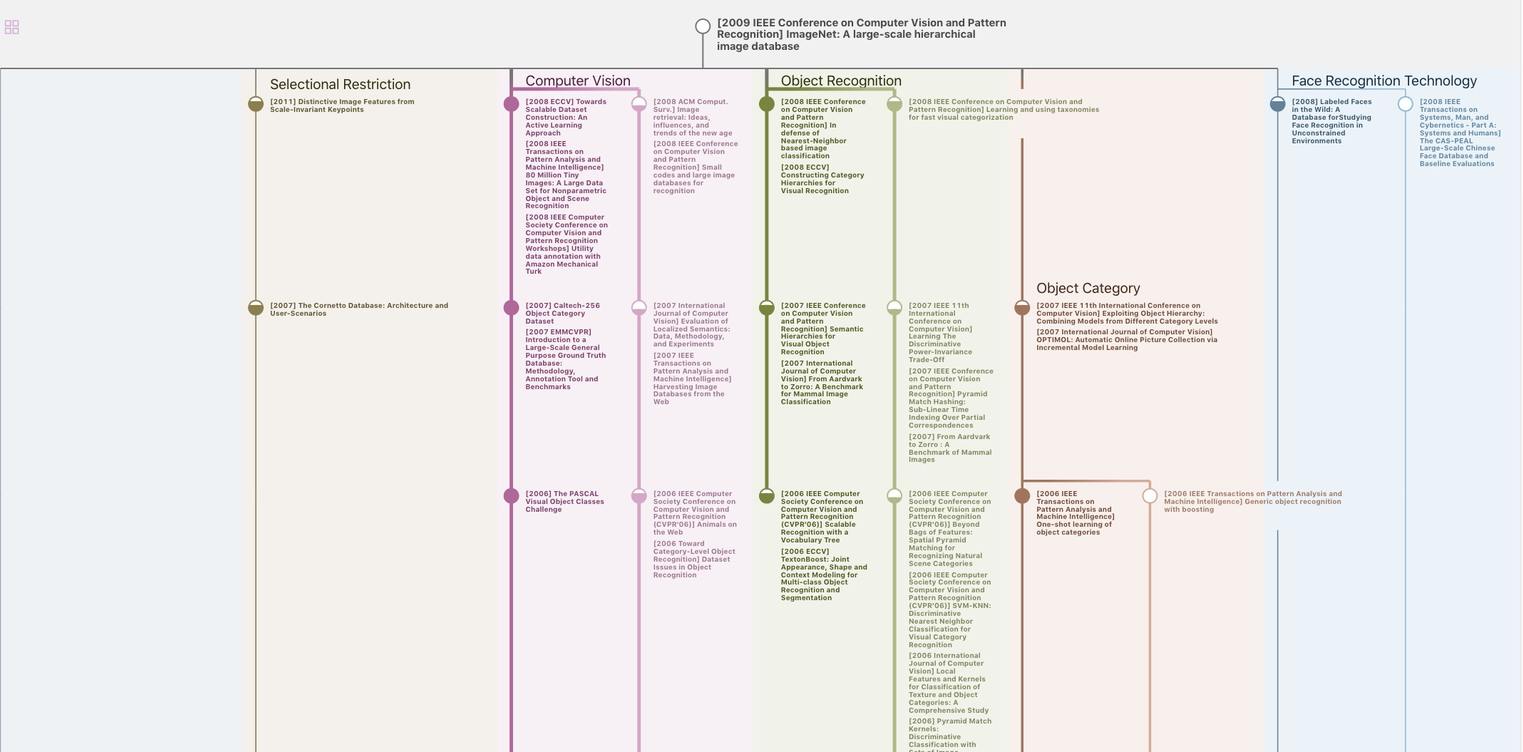
生成溯源树,研究论文发展脉络
Chat Paper
正在生成论文摘要