Low Rank Optimization for Efficient Deep Learning: Making A Balance between Compact Architecture and Fast Training
CoRR(2023)
Abstract
Deep neural networks have achieved great success in many data processing applications. However, the high computational complexity and storage cost makes deep learning hard to be used on resource-constrained devices, and it is not environmental-friendly with much power cost. In this paper, we focus on low-rank optimization for efficient deep learning techniques. In the space domain, deep neural networks are compressed by low rank approximation of the network parameters, which directly reduces the storage requirement with a smaller number of network parameters. In the time domain, the network parameters can be trained in a few subspaces, which enables efficient training for fast convergence. The model compression in the spatial domain is summarized into three categories as pre-train, pre-set, and compression-aware methods, respectively. With a series of integrable techniques discussed, such as sparse pruning, quantization, and entropy coding, we can ensemble them in an integration framework with lower computational complexity and storage. Besides of summary of recent technical advances, we have two findings for motivating future works: one is that the effective rank outperforms other sparse measures for network compression. The other is a spatial and temporal balance for tensorized neural networks.
MoreTranslated text
Key words
model compression,subspace training,effective rank,low rank tensor optimization,efficient deep learning
AI Read Science
Must-Reading Tree
Example
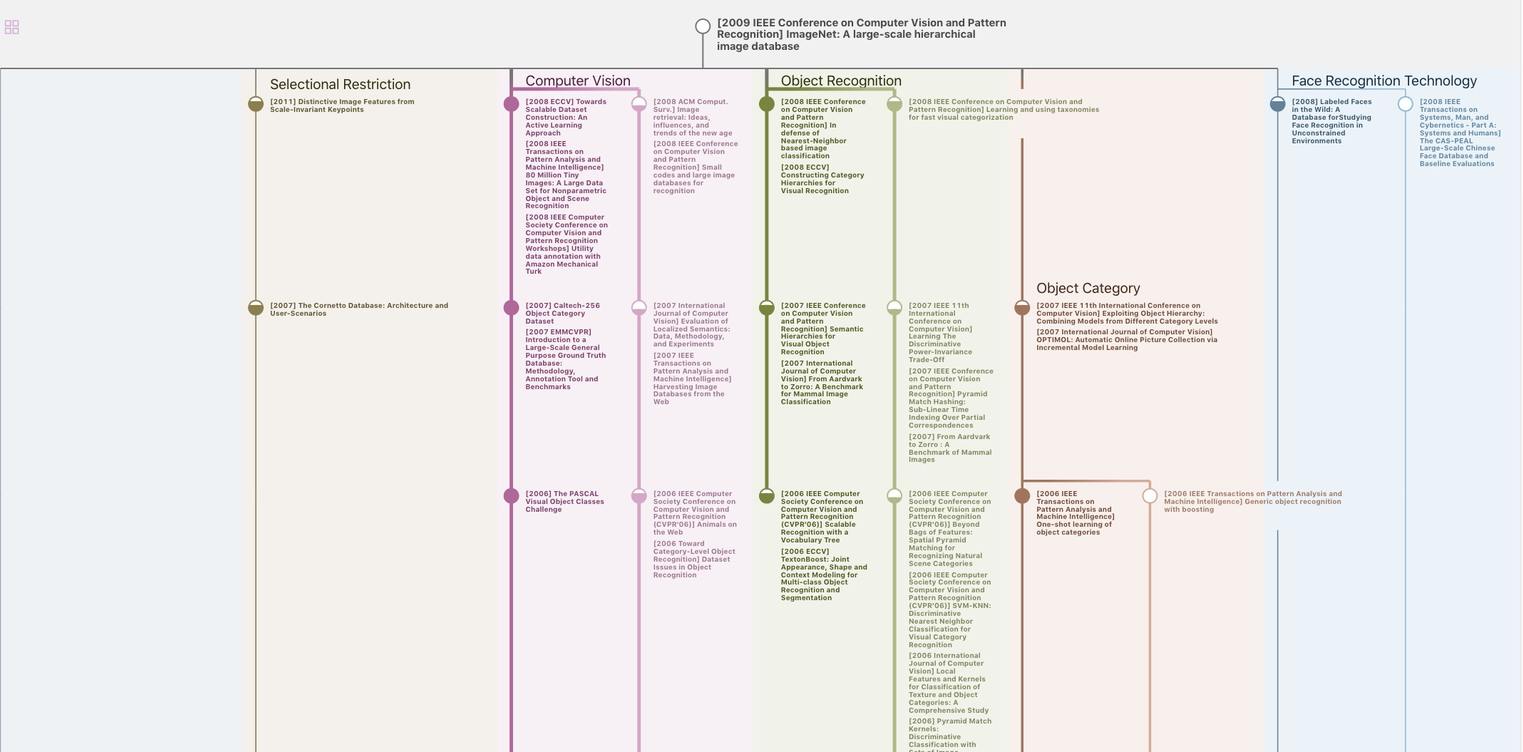
Generate MRT to find the research sequence of this paper
Chat Paper
Summary is being generated by the instructions you defined