A Model of Temporal Scaling Correctly Predicts that Weber′s Law is Speed-dependent
bioRxiv(2017)
摘要
Timing is fundamental to complex motor behaviors: from tying a knot to playing the piano. A general feature of motor timing is temporal scaling: the ability to produce motor patterns at different speeds. Here we report that temporal scaling is not automatic. After learning to produce a Morse code pattern at one speed, subjects did not accurately generalize to novel speeds. Temporal scaling was also not a general property of a recurrent neural network (RNN) model, however after training across different speeds the model produced robust temporal scaling. The model captured a signature of motor timing-Weber′s law-but predicted that temporal precision increases at faster speeds. A human psychophysics study confirmed this prediction: the standard deviation of responses in absolute time were lower at faster speeds. These results establish that RNNs can account for temporal scaling, and suggest a novel psychophysical principle: the Weber-speed effect.
更多查看译文
AI 理解论文
溯源树
样例
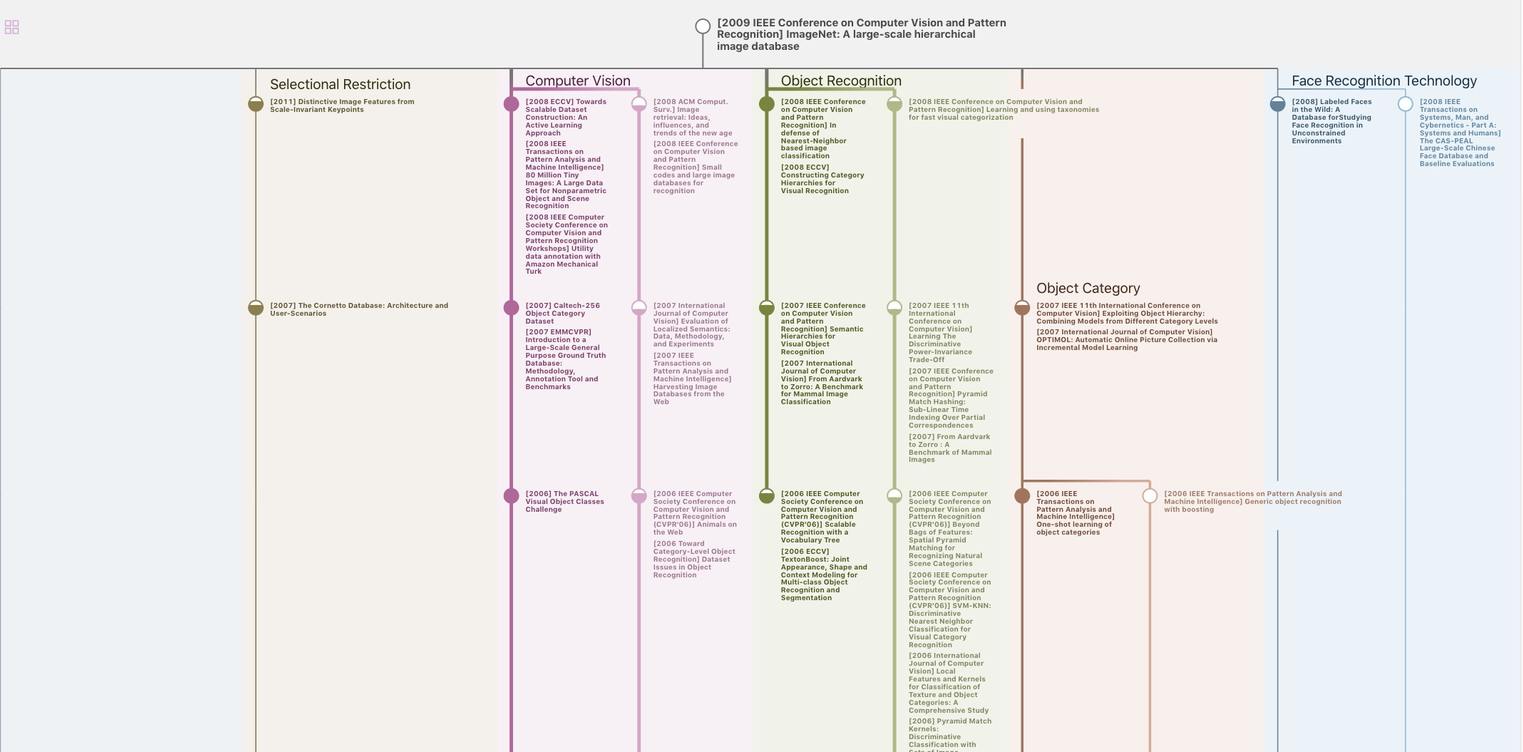
生成溯源树,研究论文发展脉络
Chat Paper
正在生成论文摘要