SU‐F‐J‐138: An Extension of PCA‐Based Respiratory Deformation Modeling Via Multi‐Linear Decomposition
MEDICAL PHYSICS(2016)
Abstract
Purpose:To address and lift the limited degree of freedom (DoF) of globally bilinear motion components such as those based on principal components analysis (PCA), for encoding and modeling volumetric deformation motion.Methods:We provide a systematic approach to obtaining a multi-linear decomposition (MLD) and associated motion model from deformation vector field (DVF) data. We had previously introduced MLD for capturing multi-way relationships between DVF variables, without being restricted by the bilinear component format of PCA-based models. PCA-based modeling is commonly used for encoding patient-specific deformation as per planning 4D-CT images, and aiding on-board motion estimation during radiotherapy. However, the bilinear space-time decomposition inherently limits the DoF of such models by the small number of respiratory phases. While this limit is not reached in model studies using analytical or digital phantoms with low-rank motion, it compromises modeling power in the presence of relative motion, asymmetries and hysteresis, etc, which are often observed in patient data. Specifically, a low-DoF model will spuriously couple incoherent motion components, compromising its adaptability to on-board deformation changes. By the multi-linear format of extracted motion components, MLD-based models can encode higher-DoF deformation structure.Results:We conduct mathematical and experimental comparisons between PCA- and MLD-based models. A set of temporally-sampled analytical trajectories provides a synthetic, high-rank DVF; trajectories correspond to respiratory and cardiac motion factors, including different relative frequencies and spatial variations. Additionally, a digital XCAT phantom is used to simulate a lung lesion deforming incoherently with respect to the body, which adheres to a simple respiratory trend. In both cases, coupling of incoherent motion components due to a low model DoF is clearly demonstrated.Conclusion:Multi-linear decomposition can enable decoupling of distinct motion factors in high-rank DVF measurements. This may improve motion model expressiveness and adaptability to on-board deformation, aiding model-based image reconstruction for target verification.NIH Grant No. R01-184173
MoreTranslated text
Key words
decomposition,deformation,modeling,pca-based,multi-linear
AI Read Science
Must-Reading Tree
Example
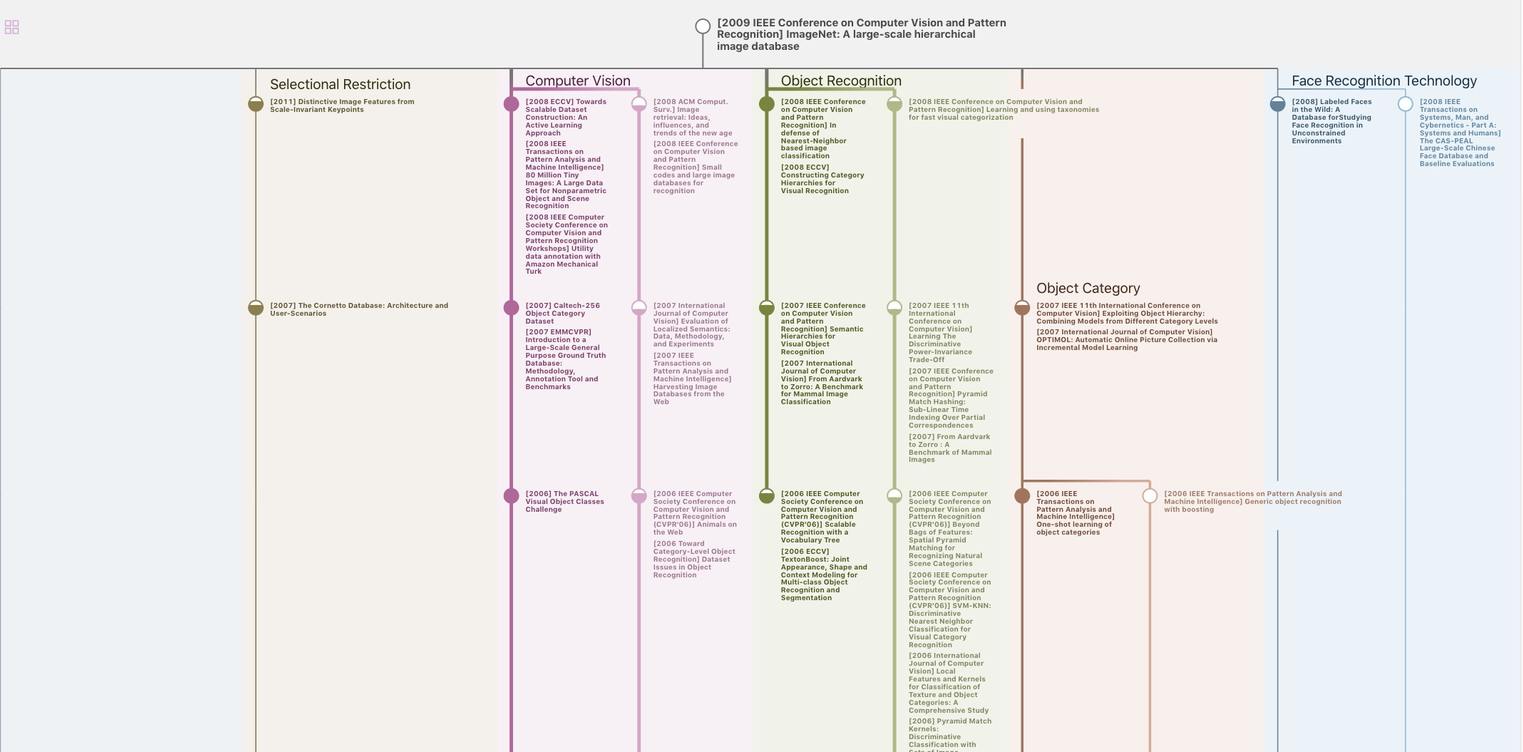
Generate MRT to find the research sequence of this paper
Chat Paper
Summary is being generated by the instructions you defined