Dealing with Label Scarcity in Computational Pathology: A Use Case in Prostate Cancer Classification
CoRR(2019)
摘要
Large amounts of unlabelled data are commonplace for many applications in computational pathology, whereas labelled data is often expensive, both in time and cost, to acquire. We investigate the performance of unsupervised and supervised deep learning methods when few labelled data are available. Three methods are compared: clustering autoencoder latent vectors (unsupervised), a single layer classifier combined with a pre-trained autoencoder (semi-supervised), and a supervised CNN. We apply these methods on hematoxylin and eosin (H E) stained prostatectomy images to classify tumour versus non-tumour tissue. Results show that semi-/unsupervised methods have an advantage over supervised learning when few labels are available. Additionally, we show that incorporating immunohistochemistry (IHC) stained data provides an increase in performance over only using H E.
更多查看译文
关键词
label scarcity,computational pathology,prostate cancer,classification
AI 理解论文
溯源树
样例
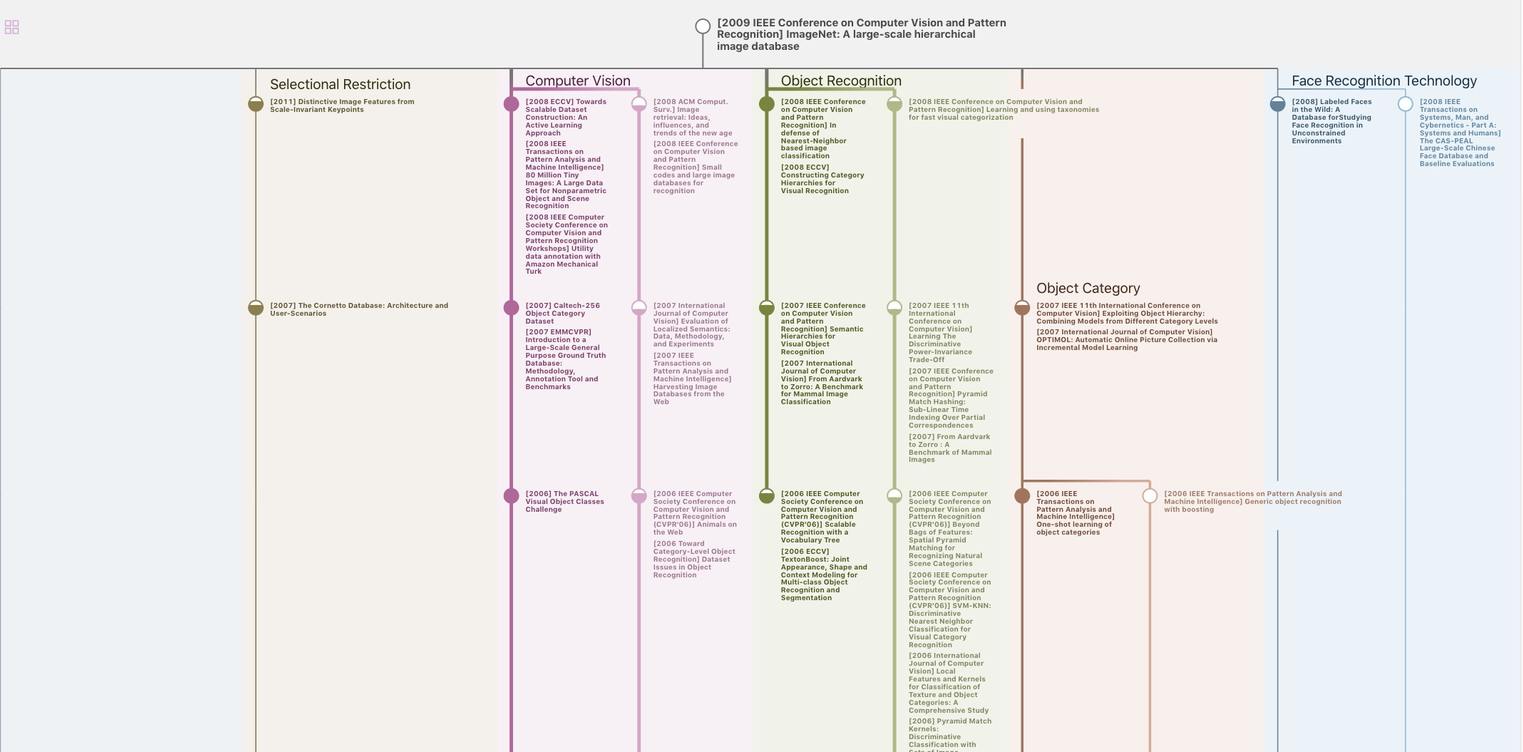
生成溯源树,研究论文发展脉络
Chat Paper
正在生成论文摘要