Research on Texture Defect Detection Based on Faster-RCNN and Feature Fusion
Proceedings of the 2019 11th International Conference on Machine Learning and Computing(2019)
摘要
Product texture defect detection is one of the important quality inspection procedures in industrial production. For the traditional defect detection methods, the detection processes are cumbersome, the accuracies are not high, and the generalizations are not strong. This paper proposes a method based on Faster-RCNN and feature fusion. This method uses the ResNet network model to extract the shared convolution feature, and combines the high-level features of the ROI pooling layer output with the low-level features obtained by the direction gradient histogram (HOG) as full connection layer input. Then, optimizing the model by adjusting the training parameters and convolutional neural network structure. Experiments on the German Pattern Recognition Association (GAPR) texture defect dataset show that the proposed model has improved in the mAP index. Through the migration learning strategy, experiments are carried out on several sets of actually collected data sets. The experimental results show that the model has good adaptability and can be applied to the surface defect detection of workpieces under different conditions.
更多查看译文
关键词
defect detection, faster-RCNN, feature fusion, target location
AI 理解论文
溯源树
样例
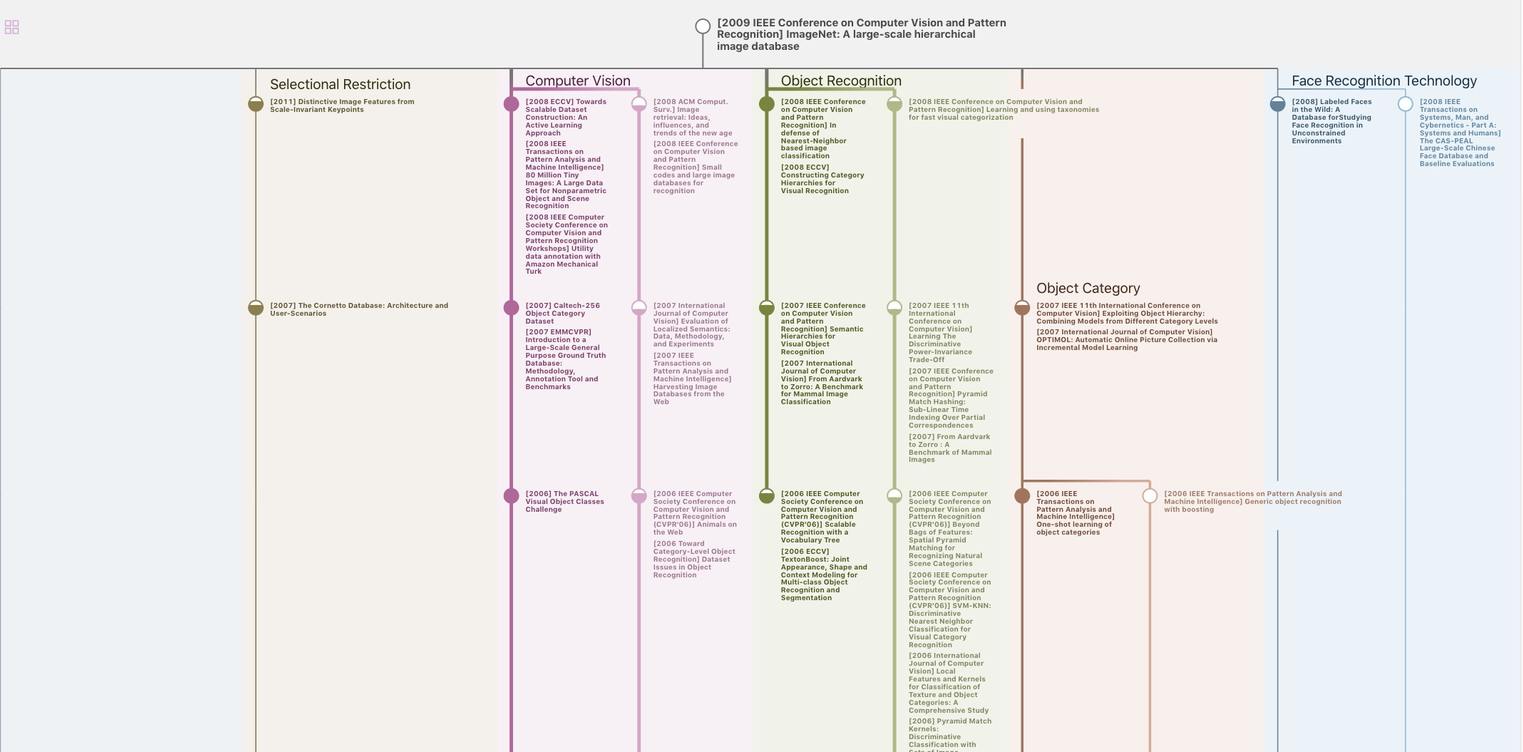
生成溯源树,研究论文发展脉络
Chat Paper
正在生成论文摘要