Deep Visuo-Tactile Learning: Estimation Of Tactile Properties From Images
2019 INTERNATIONAL CONFERENCE ON ROBOTICS AND AUTOMATION (ICRA)(2019)
摘要
Estimation of tactile properties from vision, such as slipperiness or roughness, is important to effectively interact with the environment. These tactile properties help us decide which actions we should choose and how to perform them. E.g., we can drive slower if we see that we have bad traction or grasp tighter if an item looks slippery. We believe that this ability also helps robots to enhance their understanding of the environment, and thus enables them to tailor their actions to the situation at hand. We therefore propose a model to estimate the degree of tactile properties from visual perception alone (e.g., the level of slipperiness or roughness). Our method extends a encoder-decoder network, in which the latent variables are visual and tactile features. In contrast to previous works, our method does not require manual labeling, but only RGB images and the corresponding tactile sensor data. All our data is collected with a webcam and uSkin tactile sensor mounted on the end-effector of a Sawyer robot, which strokes the surfaces of 25 different materials. We show that our model generalizes to materials not included in the training data by evaluating the feature space, indicating that it has learned to associate important tactile properties with images.
更多查看译文
关键词
deep visuo-tactile learning,tactile sensor data,Webcam,tactile properties,visual perception,encoder-decoder network,latent variables,tactile features,visual features,RGB images,uSkin tactile sensor,end-effector,feature space,Sawyer robot
AI 理解论文
溯源树
样例
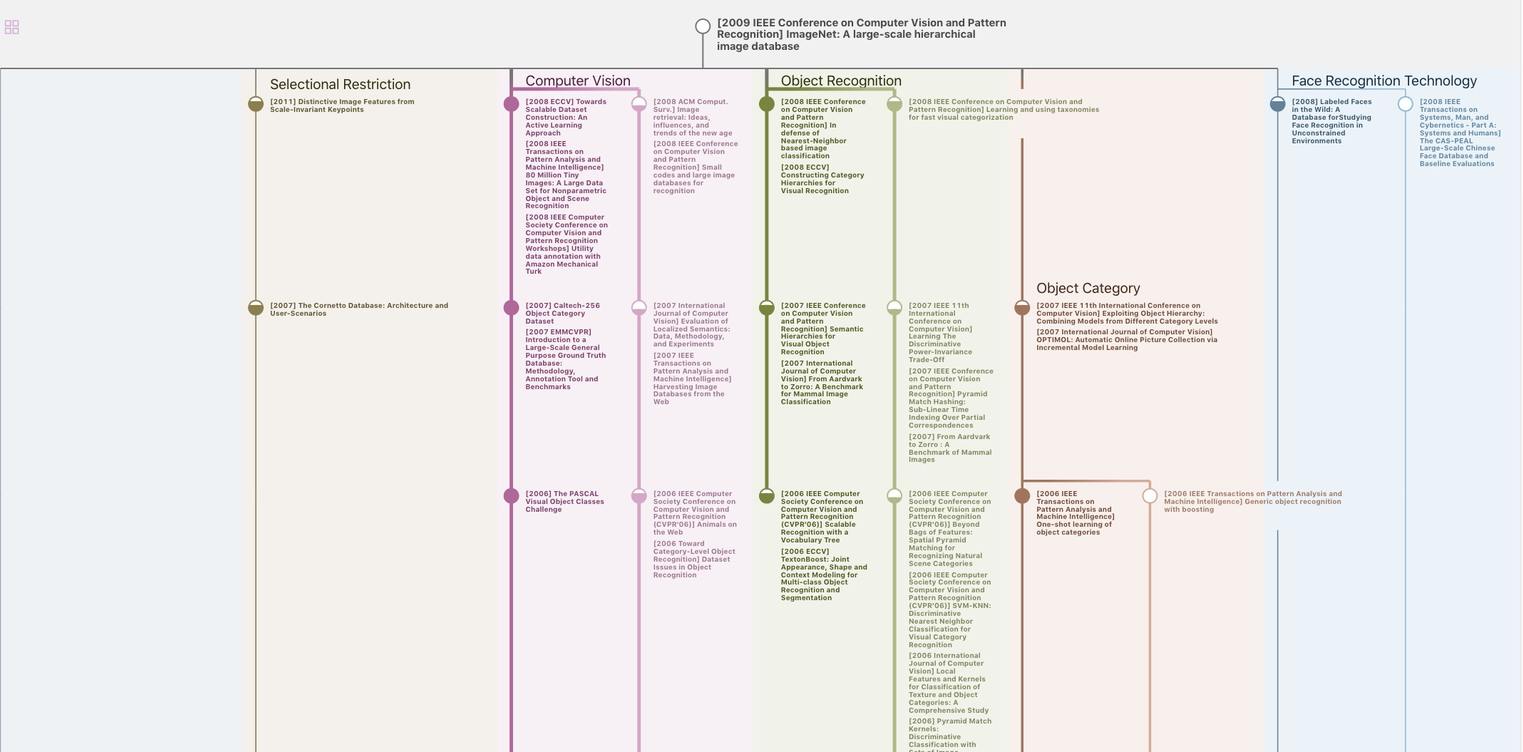
生成溯源树,研究论文发展脉络
Chat Paper
正在生成论文摘要