A Novel K-medoids clustering recommendation algorithm based on probability distribution for collaborative filtering.
Knowledge-Based Systems(2019)
Abstract
Data sparsity is a widespread problem of collaborative filtering (CF) recommendation algorithms. However, some common CF methods cannot adequately utilize all user rating information; they are only able to use a small part of the rating data, depending on the co-rated items, which leads to low prediction accuracy. To alleviate this problem, a novel K-medoids clustering recommendation algorithm based on probability distribution for CF is proposed. The proposed scheme makes full use of all rating information based on Kullback–Leibler (KL) divergence from the perspective of item rating probability distribution, and distinguishes different items efficiently when selecting the cluster centers. Meanwhile, the distance model breaks the symmetric mode of classic geometric distance methods (such as Euclidean distance) and considers the effects of different rating numbers between items to emphasize their asymmetric relationship. Experimental results on different datasets show that the proposed clustering algorithm outperforms other compared methods in various evaluation metrics; this approach enhances the prediction accuracy and effectively deals with the sparsity problem.
MoreTranslated text
Key words
Collaborative filtering,Clustering,K-medoids algorithm,Kullback–Leibler (KL) divergence,Probability distribution
AI Read Science
Must-Reading Tree
Example
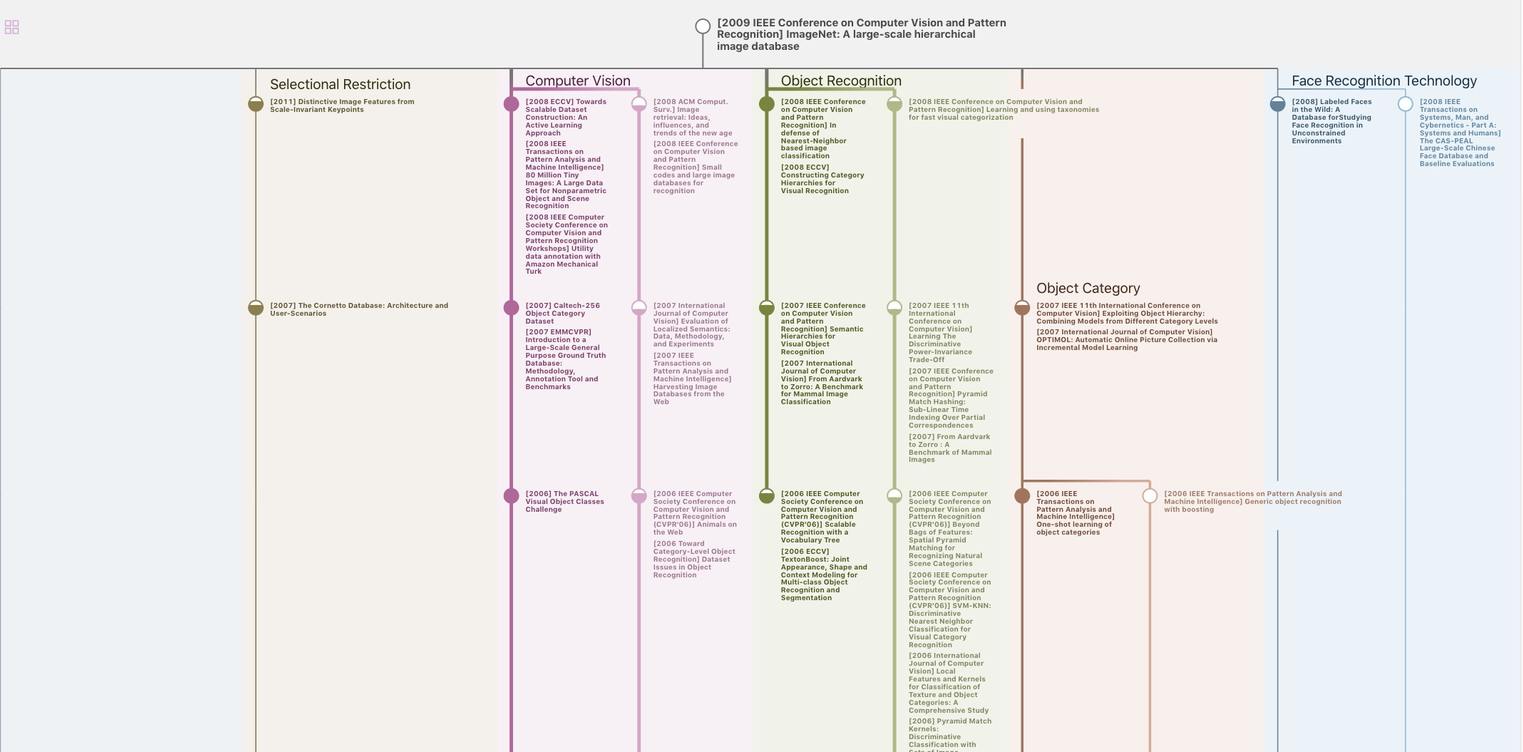
Generate MRT to find the research sequence of this paper
Chat Paper
Summary is being generated by the instructions you defined