Exploring the Hyperparameter Landscape of Adversarial Robustness
CoRR(2019)
摘要
Adversarial training shows promise as an approach for training models that are robust towards adversarial perturbation. In this paper, we explore some of the practical challenges of adversarial training. We present a sensitivity analysis that illustrates that the effectiveness of adversarial training hinges on the settings of a few salient hyperparameters. We show that the robustness surface that emerges across these salient parameters can be surprisingly complex and that therefore no effective one-size-fits-all parameter settings exist. We then demonstrate that we can use the same salient hyperparameters as tuning knob to navigate the tension that can arise between robustness and accuracy. Based on these findings, we present a practical approach that leverages hyperparameter optimization techniques for tuning adversarial training to maximize robustness while keeping the loss in accuracy within a defined budget.
更多查看译文
关键词
robustness,hyperparameter landscape
AI 理解论文
溯源树
样例
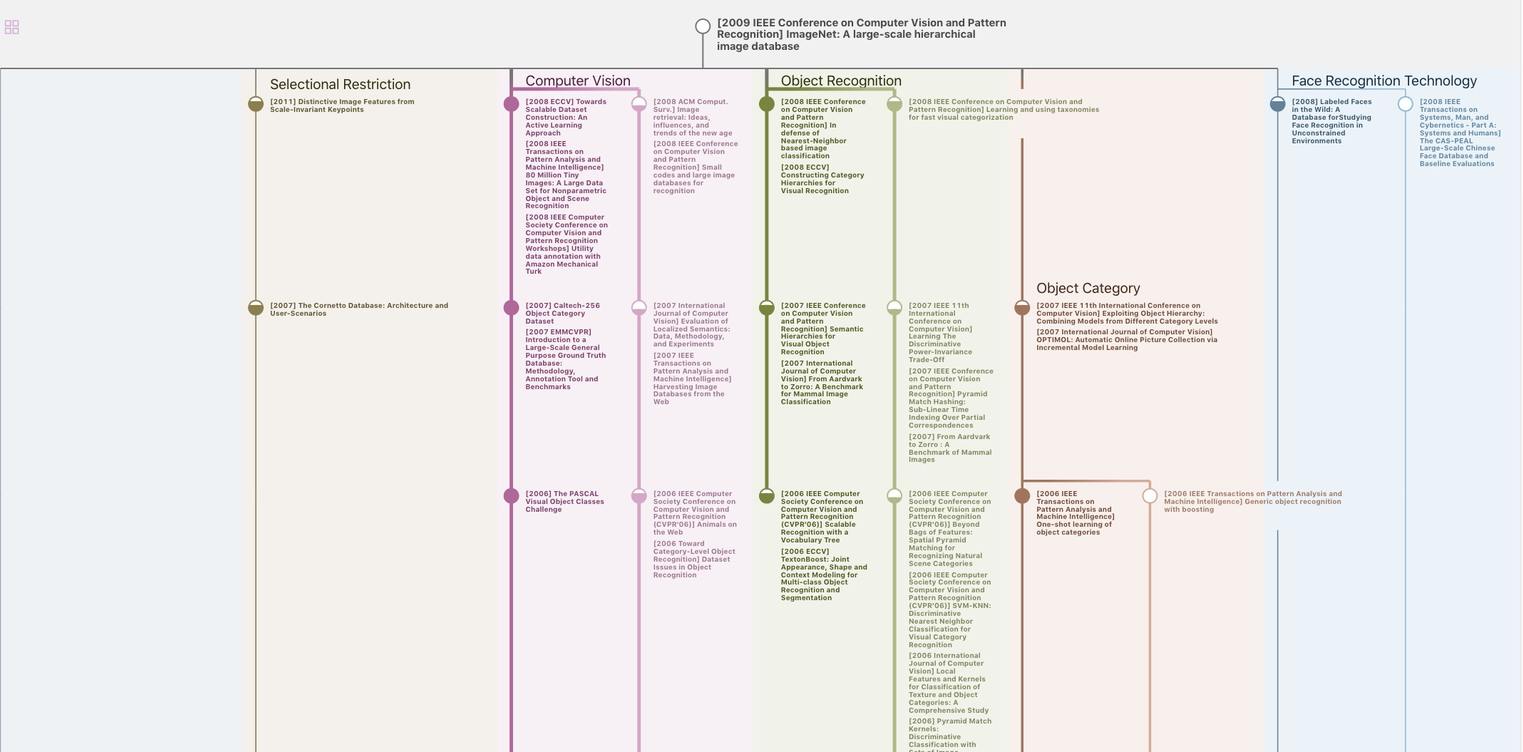
生成溯源树,研究论文发展脉络
Chat Paper
正在生成论文摘要