Convolutional Neural Networks Utilizing Multifunctional Spin-Hall MTJ Neurons.
arXiv: Emerging Technologies(2019)
Abstract
We propose a new network architecture for standard spin-Hall magnetic tunnel junction-based spintronic neurons that allows them to compute multiple critical convolutional neural network functionalities simultaneously and in parallel, saving space and time. An approximation to the Rectified Linear Unit transfer function and the local pooling function are computed simultaneously with the convolution operation itself. A proof-of-concept simulation is performed on the MNIST dataset, achieving up to 98% accuracy at a cost of less than 1 nJ for all convolution, activation and pooling operations combined. The simulations are remarkably robust to thermal noise, performing well even with very small magnetic layers.
MoreTranslated text
AI Read Science
Must-Reading Tree
Example
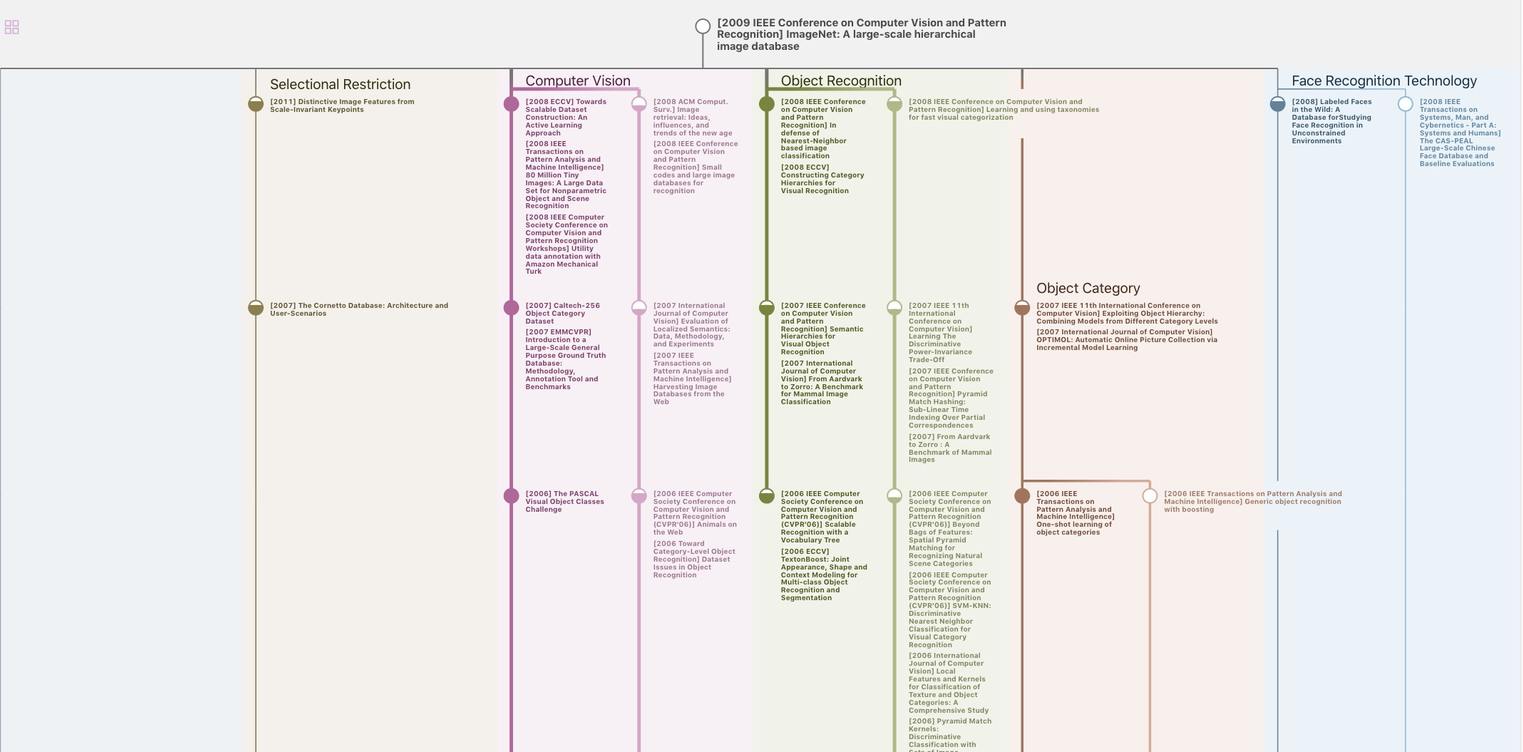
Generate MRT to find the research sequence of this paper
Chat Paper
Summary is being generated by the instructions you defined