Towards Self-Adaptive Metric Learning On the Fly
WWW '19: The Web Conference on The World Wide Web Conference WWW 2019(2019)
摘要
Good quality similarity metrics can significantly facilitate the performance of many large-scale, real-world applications. Existing studies have proposed various solutions to learn a Mahalanobis or bilinear metric in an online fashion by either restricting distances between similar (dissimilar) pairs to be smaller (larger) than a given lower (upper) bound or requiring similar instances to be separated from dissimilar instances with a given margin. However, these linear metrics learned by leveraging fixed bounds or margins may not perform well in real-world applications, especially when data distributions are complex. We aim to address the open challenge of “Online Adaptive Metric Learning” (OAML) for learning adaptive metric functions on-the-fly. Unlike traditional online metric learning methods, OAML is significantly more challenging since the learned metric could be non-linear and the model has to be self-adaptive as more instances are observed. In this paper, we present a new online metric learning framework that attempts to tackle the challenge by learning a ANN-based metric with adaptive model complexity from a stream of constraints. In particular, we propose a novel Adaptive-Bound Triplet Loss (ABTL) to effectively utilize the input constraints, and present a novel Adaptive Hedge Update (AHU) method for online updating the model parameters. We empirically validates the effectiveness and efficacy of our framework on various applications such as real-world image classification, facial verification, and image retrieval.
更多查看译文
关键词
Adaptive Metric Complexity, Adaptive-Bound Triplet Loss, Online Metric Learning
AI 理解论文
溯源树
样例
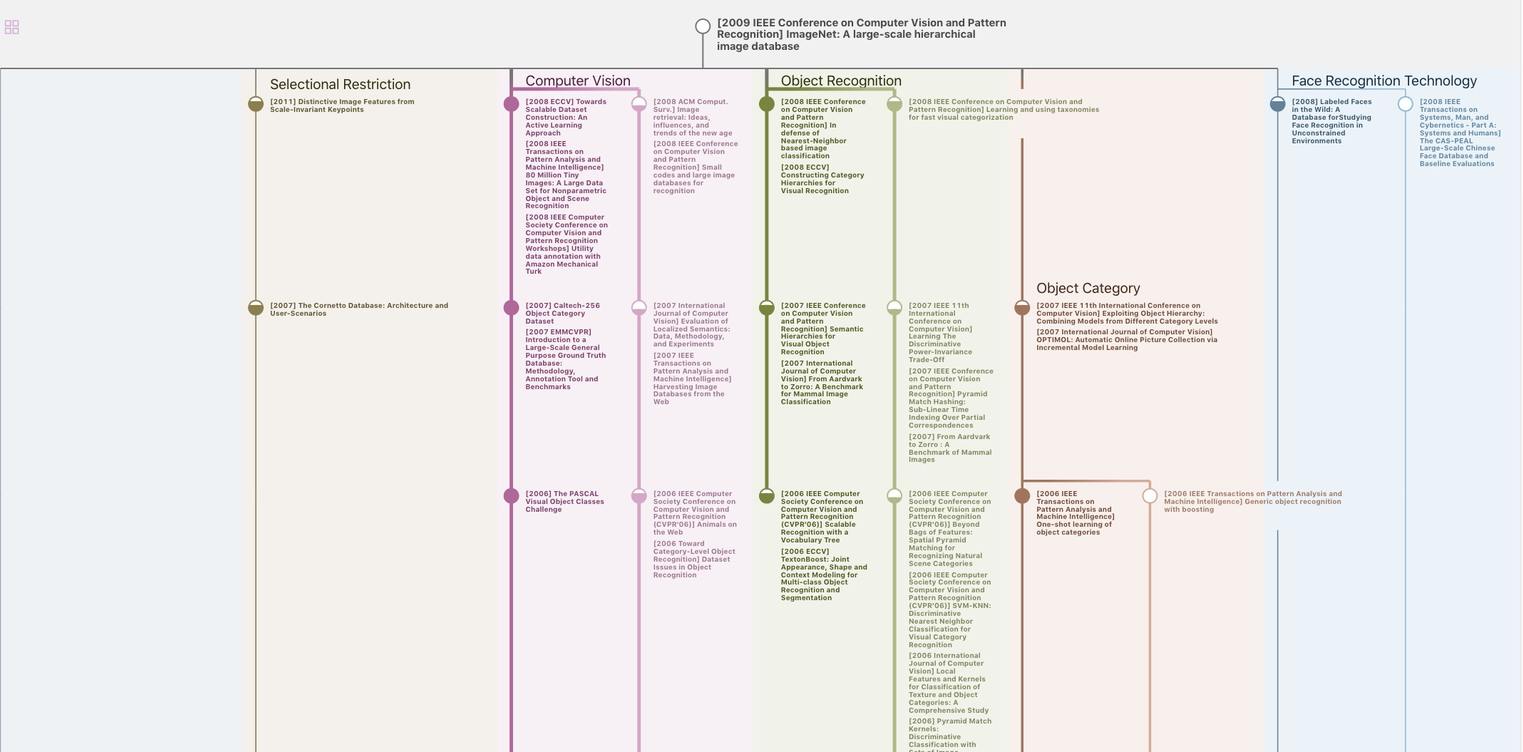
生成溯源树,研究论文发展脉络
Chat Paper
正在生成论文摘要