Scalable Supervised Asymmetric Hashing with Semantic and Latent Factor Embedding.
IEEE transactions on image processing : a publication of the IEEE Signal Processing Society(2019)
摘要
Compact hash code learning has been widely applied to fast similarity search owing to its significantly reduced storage and highly efficient query speed. However, it is still a challenging task to learn discriminative binary codes for perfectly preserving the full pairwise similarities embedded in the high-dimensional real-valued features, such that the promising performance can be guaranteed. To overcome this difficulty, in this paper, we propose a novel scalable supervised asymmetric hashing (SSAH) method, which can skillfully approximate the full-pairwise similarity matrix based on maximum asymmetric inner product of two different non-binary embeddings. In particular, to comprehensively explore the semantic information of data, the supervised label information and the refined latent feature embedding are simultaneously considered to construct the high-quality hashing function and boost the discriminant of the learned binary codes. Specifically, SSAH learns two distinctive hashing functions in conjunction of minimizing the regression loss on the semantic label alignment and the encoding loss on the refined latent features. More importantly, instead of using only part of similarity correlations of data, the full-pairwise similarity matrix is directly utilized to avoid information loss and performance degeneration, and its cumbersome computation complexity on n×n matrix can be dexterously manipulated during the optimization phase. Furthermore, an efficient alternating optimization scheme with guaranteed convergence is designed to address the resulting discrete optimization problem. The encouraging experimental results on diverse benchmark datasets demonstrate the superiority of the proposed SSAH method in comparison with many recently proposed hashing algorithms.
更多查看译文
关键词
Semantics,Binary codes,Optimization,Image coding,Task analysis,Correlation,Image retrieval
AI 理解论文
溯源树
样例
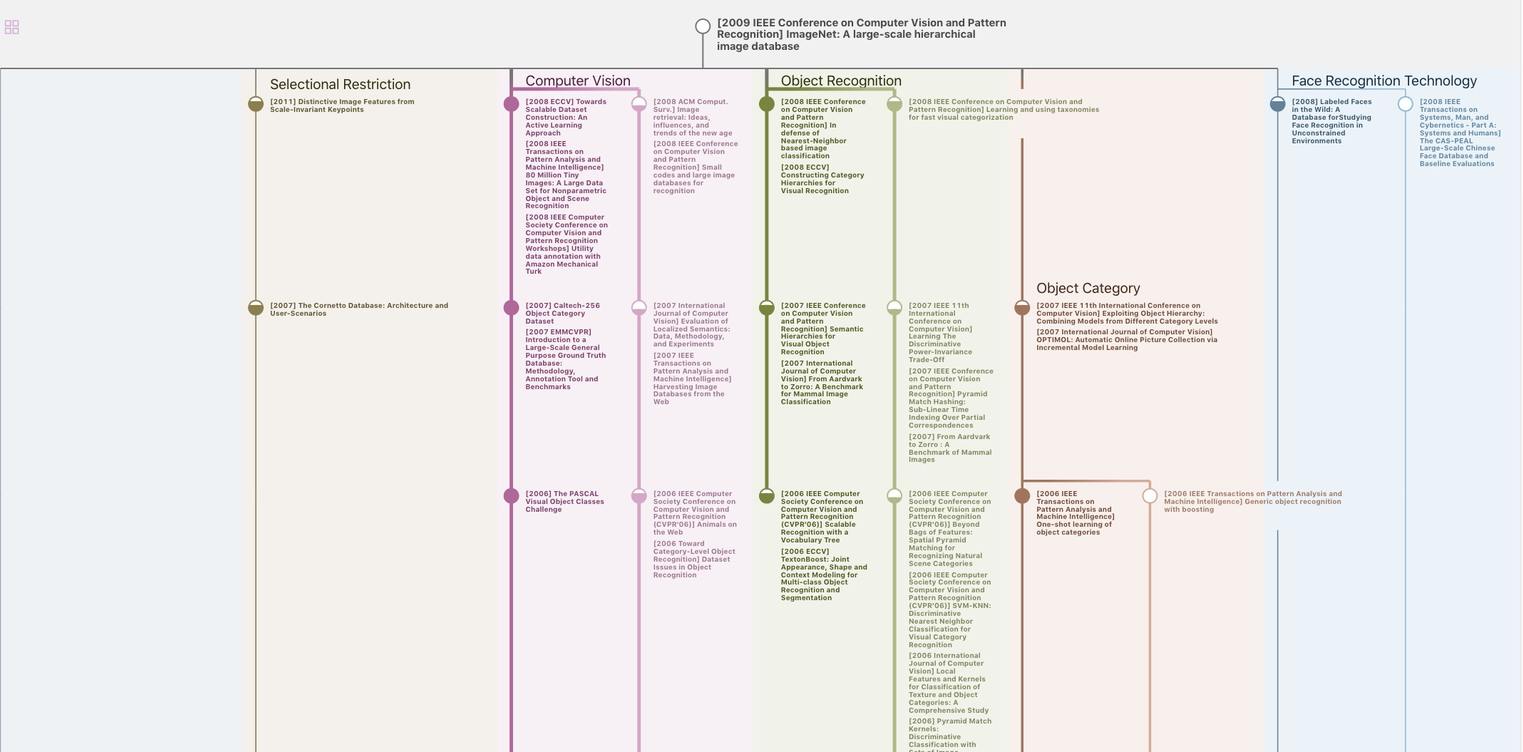
生成溯源树,研究论文发展脉络
Chat Paper
正在生成论文摘要