Caveats in Generating Medical Imaging Labels from Radiology Reports.
arXiv: Computation and Language(2019)
摘要
Acquiring high-quality annotations in medical imaging is usually a costly process. Automatic label extraction with natural language processing (NLP) has emerged as a promising workaround to bypass the need of expert annotation. Despite the convenience, the limitation of such an approximation has not been carefully examined and is not well understood. With a challenging set of 1,000 chest X-ray studies and their corresponding radiology reports, we show that there exists a surprisingly large discrepancy between what radiologists visually perceive and what they clinically report. Furthermore, with inherently flawed report as ground truth, the state-of-the-art medical NLP fails to produce high-fidelity labels.
更多查看译文
关键词
generating medical imaging labels,radiology reports
AI 理解论文
溯源树
样例
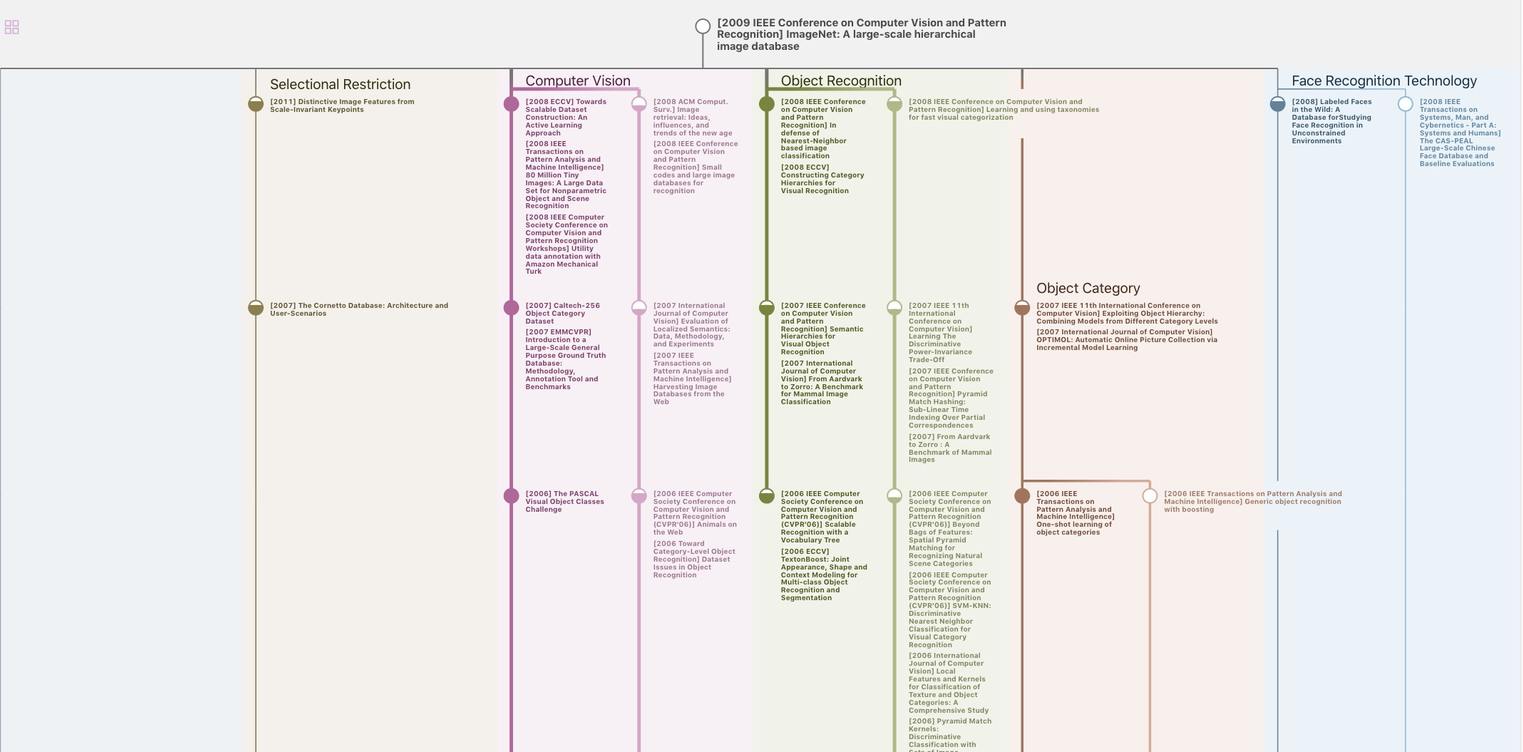
生成溯源树,研究论文发展脉络
Chat Paper
正在生成论文摘要