Diffuse to fuse EEG spectra -- intrinsic geometry of sleep dynamics for classification
arXiv: Signal Processing(2019)
摘要
We propose a novel algorithm for sleep dynamics visualization and automatic annotation by applying diffusion geometry based sensor fusion algorithm to fuse spectral information from two electroencephalograms (EEG). The diffusion geometry approach helps organize the nonlinear dynamical structure hidden in the EEG signal. The visualization is achieved by the nonlinear dimension reduction capability of the chosen diffusion geometry algorithms. For the automatic annotation purpose, the {support vector machine} is trained to predict the sleep stage. The prediction performance is validated on a publicly available benchmark database, Physionet Sleep-EDF [extended] SC$^*$ {(SC = Sleep Cassette)} and ST$^*$ {(ST = Sleep Telemetry)}, with the leave-one-subject-out cross validation. When we have a single EEG channel (Fpz-Cz), the overall accuracy, macro F1 and Cohen's kappa achieve $82.72\%$,$75.91\%$ and $76.1\%$ respectively in Sleep-EDF SC$^*$ and $78.63\%$, $73.58\%$ and $69.48\%$ in Sleep-EDF ST$^*$. This performance is compatible {with} the state-of-the-art results. When we have two EEG channels (Fpz-Cz and Pz-Oz), the overall accuracy, macro F1 and Cohen's kappa achieve $84.44\%$,$78.25\%$ and $78.36\%$ respectively in Sleep-EDF SC$^*$ and $79.05\%$, $74.73\%$ and $70.31\%$ in Sleep-EDF ST$^*$. The results suggest the potential of the proposed algorithm in practical applications.
更多查看译文
关键词
Electroencephalogram,Scattering transform,Sensor fusion,Alternating diffusion map,Multiview diffusion,Sleep stage
AI 理解论文
溯源树
样例
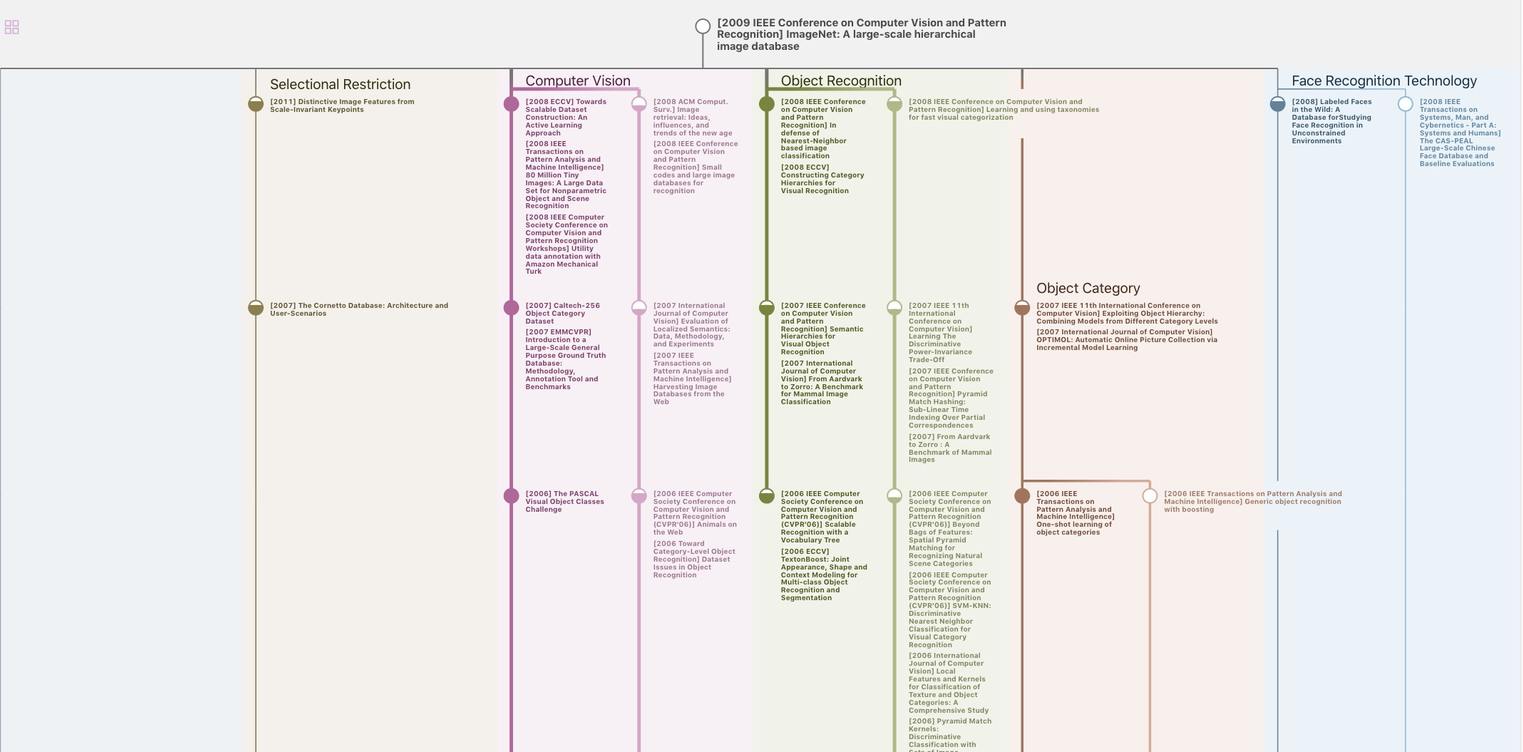
生成溯源树,研究论文发展脉络
Chat Paper
正在生成论文摘要