Robust deep learning method for choroidal vessel segmentation on swept source optical coherence tomography images.
BIOMEDICAL OPTICS EXPRESS(2019)
Abstract
Accurate choroidal vessel segmentation with swept-source optical coherence tomography (SS-OCT) images provide unprecedented quantitative analysis towards the understanding of choroid-related diseases. Motivated by the leading segmentation performance in medical images from the use of deep learning methods, in this study, we proposed the adoption of a deep learning method, RefineNet, to segment the choroidal vessels from SS-OCT images. We quantitatively evaluated the RefineNet on 40 SS-OCT images consisting of similar to 3,900 manually annotated choroidal vessels regions. We achieved a segmentation agreement (SA) of 0.840 +/- 0.035 with clinician 1 (C1) and 0.823 +/- 0.027 with clinician 2 (C2). These results were higher than inter-observer variability measure in SA between C1 and C2 of 0.821 +/- 0.037. Our results demonstrated that the choroidal vessels from SS-OCT can be automatically segmented using a deep learning method and thus provided a new approach towards an objective and reproducible quantitative analysis of vessel regions. (C) 2019 Optical Society of America under the terms of the OSA Open Access Publishing Agreement
MoreTranslated text
Key words
choroidal vessel segmentation,optical coherence tomography images,robust deep learning method
AI Read Science
Must-Reading Tree
Example
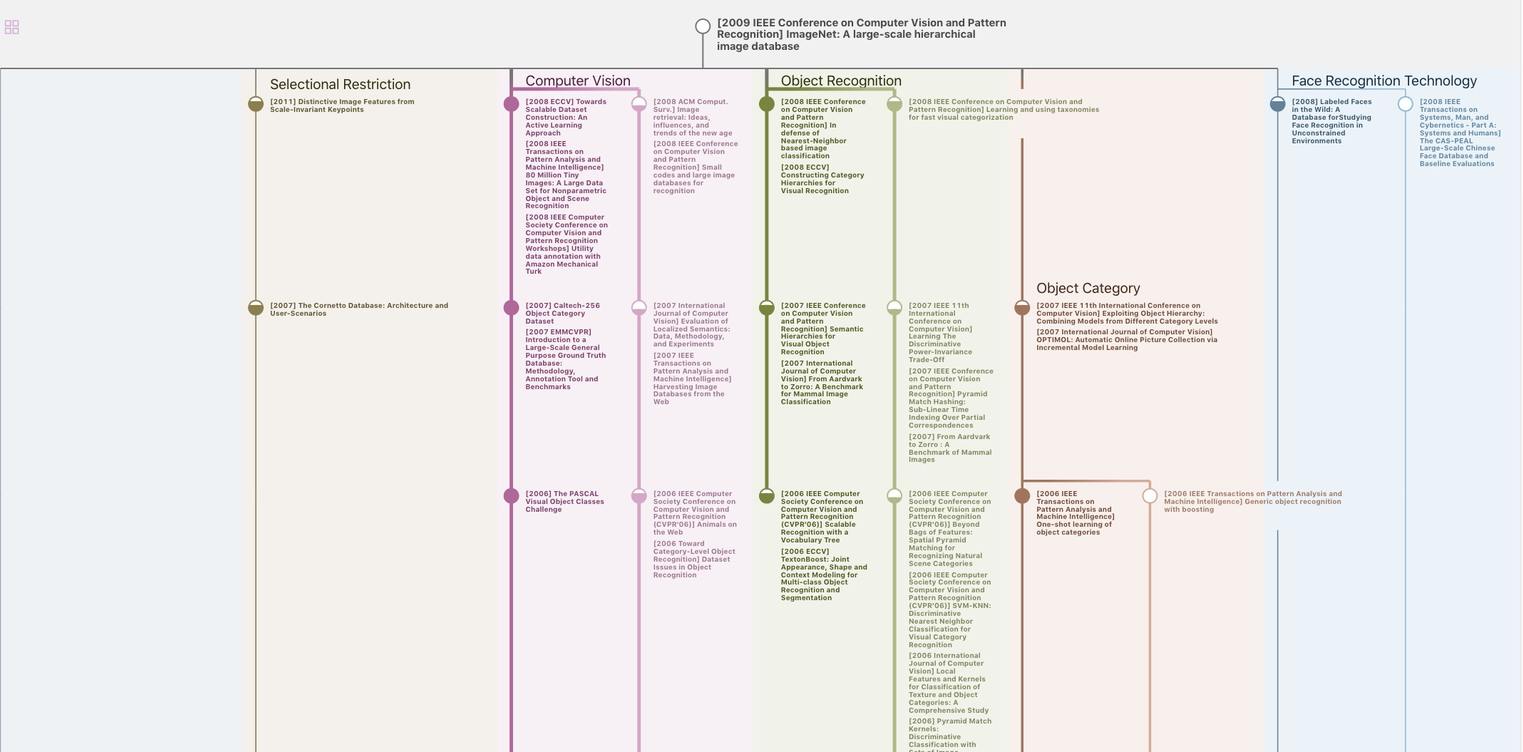
Generate MRT to find the research sequence of this paper
Chat Paper
Summary is being generated by the instructions you defined