Challenging 30-day mortality as a site-specific quality metric in non-small cell lung cancer.
The Journal of Thoracic and Cardiovascular Surgery(2019)
摘要
Objective: The objective of this project was to assess the best measure for postoperative outcomes by comparing 30-day and 90-day mortality rates after surgery for non-small cell lung cancer using the National Cancer Database. Secondarily, hospital performance was examined at multiple postoperative intervals to assess changes in ranking based on mortality up to 1 year after surgery. Methods: Patients who had undergone surgery for non-small cell lung cancer between 2004 and 2013 were identified in the National Cancer Database. Mortality rates at 30 days and 90 days were compared after adjusting for several patient characteristics, tumor variables, and hospital procedural volume using generalized logistic mixed models. Subsequently, mixed model logistic regression models were employed to evaluate hospital performance based on calculated mortality at prespecified time points. Results: A total of 303,579 patients with non-small cell lung cancer were included for analysis. The 90-day mortality was almost double the 30-day mortality (3.0% vs 5.7%). Several patient characteristics, tumor features, and hospital volume were significantly associated with mortality at both 30 days and 90 days. Hospital rankings fluctuate appreciably between early mortality time points, which is additional evidence that quality metrics need to be based on later mortality time points. Conclusions: Thirty-day mortality is the commonly accepted quality measure for thoracic surgeons; however, hospital rankings may be inaccurate if based on this variable alone. Mortality after 90 days appears to be a threshold after which there is less variability in hospital ranking and should be considered as an alternative quality metric in lung cancer surgery.
更多查看译文
关键词
30-day mortality,90-day mortality,quality metrics,hospital ranking,lung cancer surgery,National Cancer Database (NCDB)
AI 理解论文
溯源树
样例
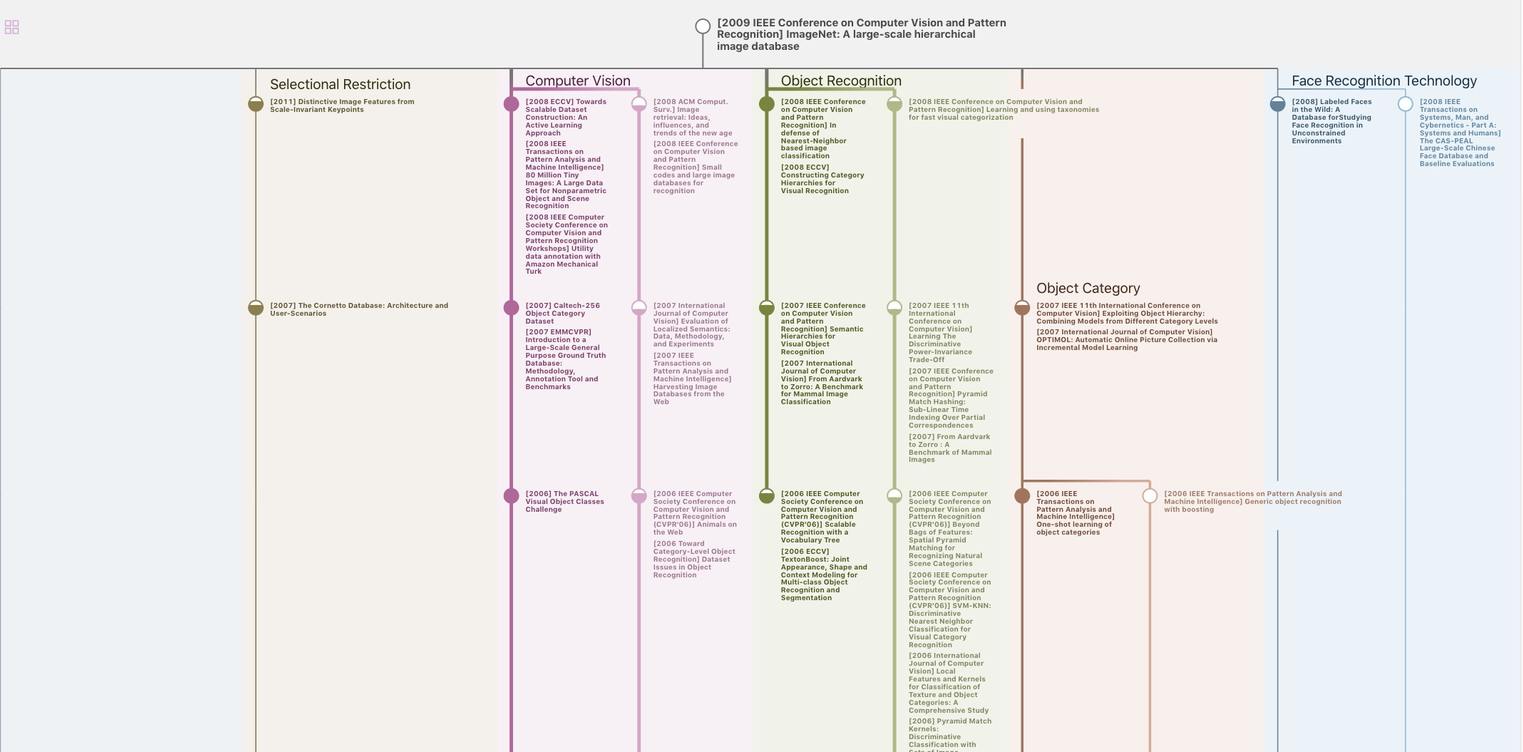
生成溯源树,研究论文发展脉络
Chat Paper
正在生成论文摘要