Scoliosis Screening through a Machine Learning Based Gait Analysis Test
International Journal of Precision Engineering and Manufacturing(2018)
摘要
This study discussed application of a machine learning approach (Support vector machine, SVM) for the automatic cognition of gait changes due to scoliosis using gait measures: kinematic based on gait phase segmentation. The gaits of 18 controls and 24 scoliosis patients were recorded and analyzed using inertial measurement unit (IMU)-based systems during normal walking. Altogether, 72 gait features were extracted for developing gait recognition models. Cross-validation test results indicated that the performance of SVM was 90.5% to recognize scoliosis patients and controls gait patterns. When features were optimally selected, a feature selection algorithm could effectively distinguish the age groups with 95.2% accuracy. Applying the method that the previous test used, the severity of scoliosis was classified after clinician labeled the severity based on the Cobb angle. Test results indicated an accuracy of 81.0% by the SVM to recognize scoliosis severity gait patterns. Optimal selected features could effectively distinguish the scoliosis severity with 85.7% accuracy. When the measured features are ranked in order of high contribution, the abduction and adduction of left hip joint in the single support phase is most important in gait of patients with scoliosis. These results demonstrate considerable potential in applying SVMs in gait classification for medical applications.
更多查看译文
关键词
Scoliosis,Gait analysis,Inertial measurement unit,Machine learning
AI 理解论文
溯源树
样例
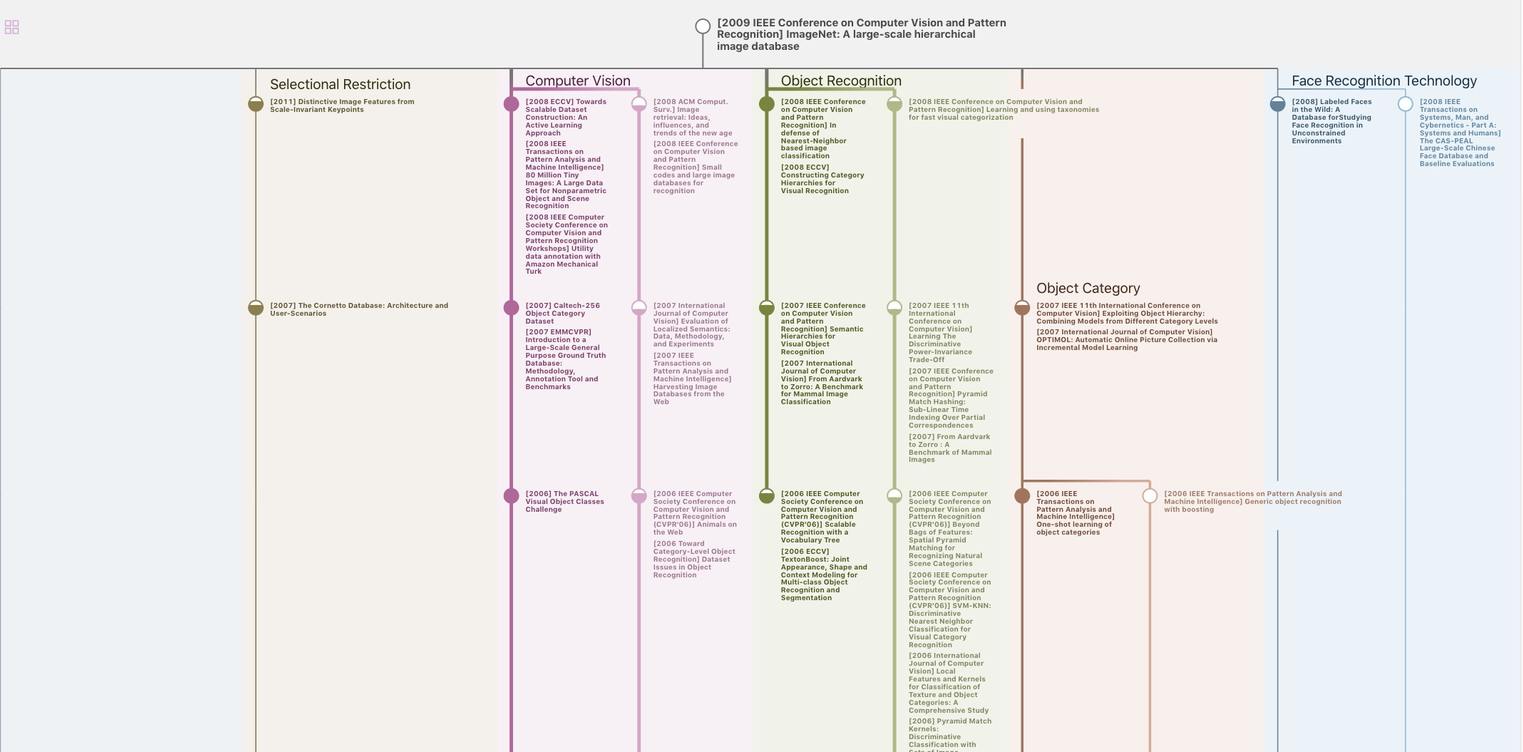
生成溯源树,研究论文发展脉络
Chat Paper
正在生成论文摘要