Using deep machine learning to detect esophageal lesions in PET-CT scans.
Proceedings of SPIE(2019)
摘要
PET-CT scans using 18F-FDG are increasingly used to detect cancer, but interpretation can be challenging due to non-specific uptake and complex anatomical structures nearby. To aide this process, we investigate the potential of automated detection of lesions in 18F-FDG scans using deep learning tools. A 5-layer convolutional neural network (CNN) with 2x2 kernels, rectified linear unit (ReLU) activations and two dense layers was trained to detect cancerous lesions in 2D axial image segments from PET scans. Pre-contoured scans from a retrospective cohort study of 486 oesophageal cancer patients were split 80:10:10 into training, validation and test sets. These were then used to generate a total of similar to 14000 25x25x25 voxel image segments, where tumor present segments were centred on the marked lesion, and tumor absent segments were randomly located outside the marked lesion. ROC curves generated from the test dataset produced an average AUC of similar to 99%. Ten-fold cross validation on unseen test data was performed which resulted in a sensitivity of 99.5 +/- 0.4% and a specificity of 99.4 +/- 0.3%. A representative model was used to successfully generate volumetric tumor probability maps for the test dataset.
更多查看译文
关键词
Deep Machine Learning,Cancer Detection,PET-CT,CNN
AI 理解论文
溯源树
样例
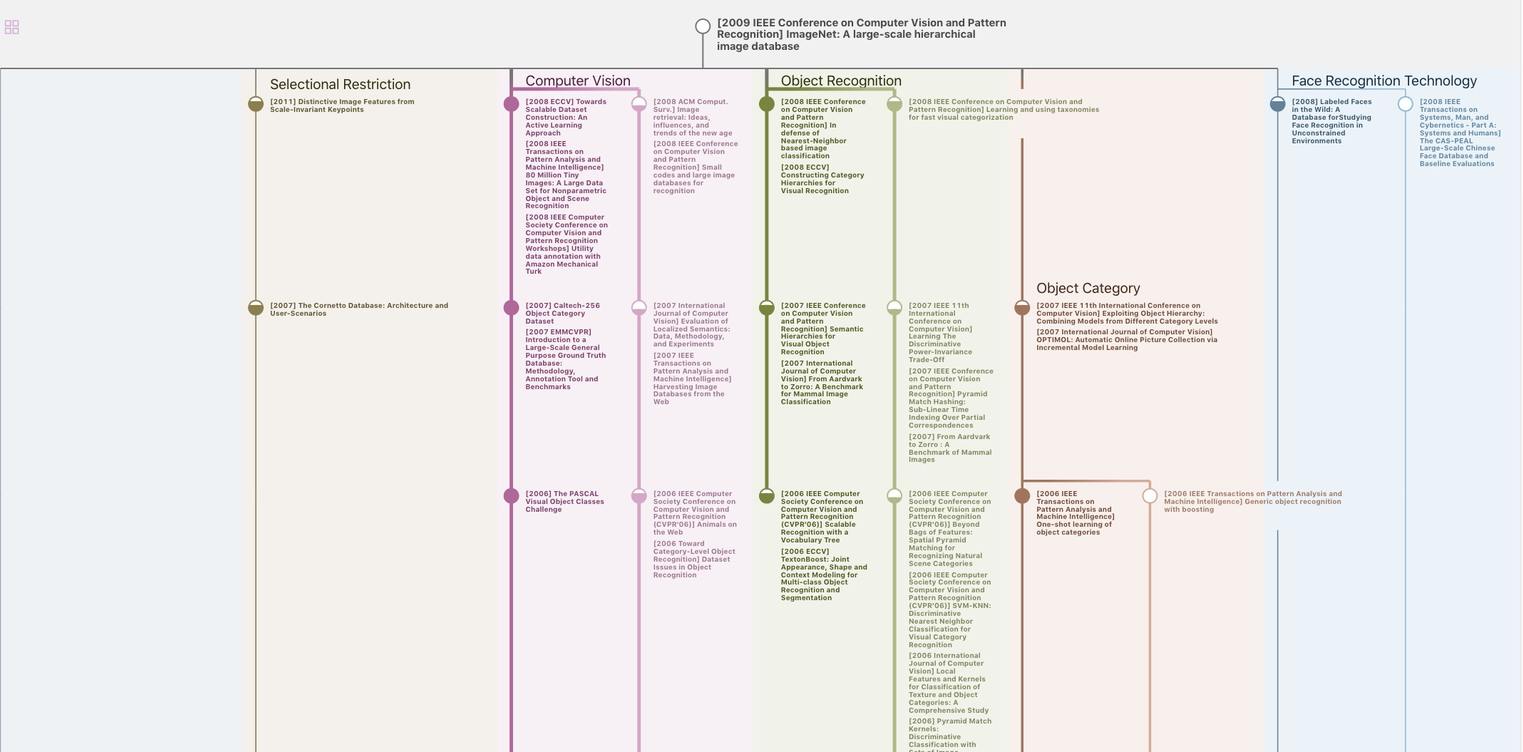
生成溯源树,研究论文发展脉络
Chat Paper
正在生成论文摘要