Partitioning and local matching learning of large biomedical ontologies.
SAC(2019)
摘要
Conventional ontology matching systems are not well-tailored to ensure sufficient quality alignments for large ontology matching tasks. In this paper, we propose a local matching learning strategy to align large and complex biomedical ontologies. We define a novel partitioning approach that breakups large ontology alignment task into a set of local sub-matching tasks. We perform a machine learning approach for each local sub-matching task. We build a local machine learning model for each sub-matching task without any user involvement. Each local matching learning model automatically provides adequate matching settings for each local sub-matching task. Our results show that: (i) partitioning approach outperforms existing techniques, (ii) local matching while using a specific machine learning model for each sub-matching task yields to promising results and (iii) the combination between partitioning and machine learning increases the overall result.
更多查看译文
关键词
machine learning, ontology matching, ontology partitioning, semantic web
AI 理解论文
溯源树
样例
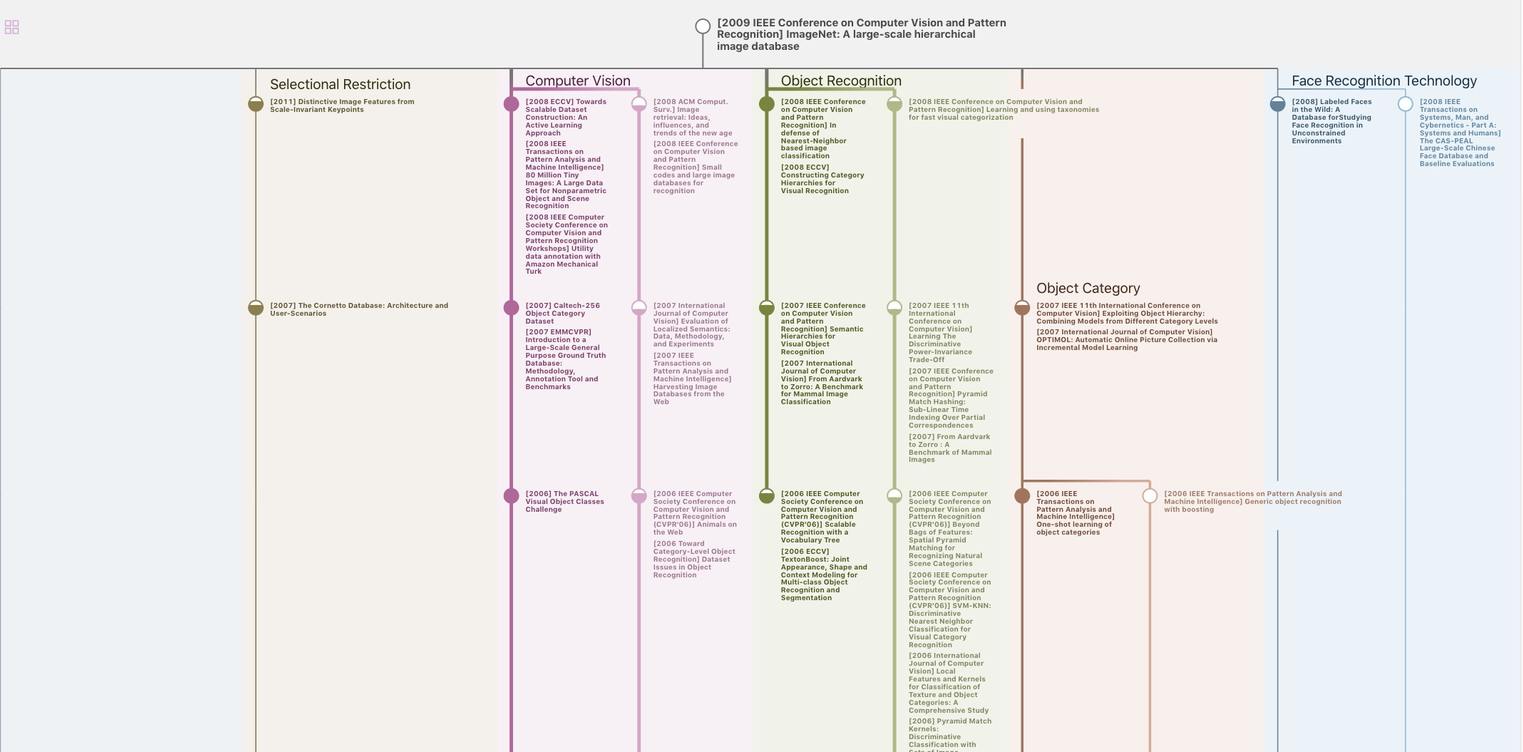
生成溯源树,研究论文发展脉络
Chat Paper
正在生成论文摘要