Indexes to Find the Optimal Number of Clusters in a Hierarchical Clustering.
14TH INTERNATIONAL CONFERENCE ON SOFT COMPUTING MODELS IN INDUSTRIAL AND ENVIRONMENTAL APPLICATIONS (SOCO 2019)(2020)
摘要
Clustering analysis is one of the most commonly used techniques for uncovering patterns in data mining. Most clustering methods require establishing the number of clusters beforehand. However, due to the size of the data currently used, predicting that value is at a high computational cost task in most cases. In this article, we present a clustering technique that avoids this requirement, using hierarchical clustering. There are many examples of this procedure in the literature, most of them focusing on the dissociative or descending subtype, while in this article we cover the agglomerative or ascending subtype. Being more expensive in computational and temporal cost, it nevertheless allows us to obtain very valuable information, regarding elements membership to clusters and their groupings, that is to say, their dendrogram. Finally, several sets of data have been used, varying their dimensionality. For each of them, we provide the calculations of internal validation indexes to test the algorithm developed, studying which of them provides better results to obtain the best possible clustering.
更多查看译文
关键词
Machine Learning,Hierarchical clustering,Internal validation indexes
AI 理解论文
溯源树
样例
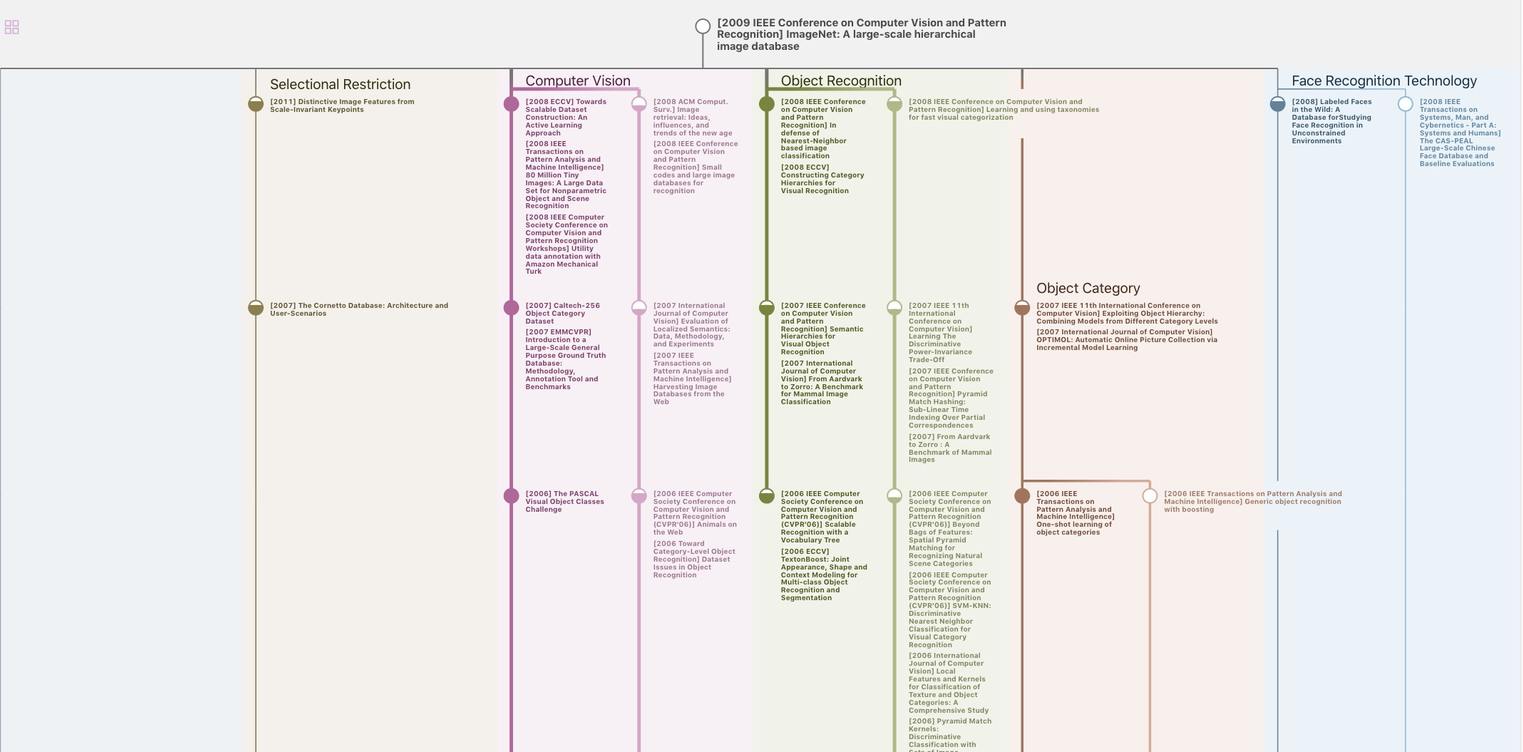
生成溯源树,研究论文发展脉络
Chat Paper
正在生成论文摘要