Use of a medication-based risk adjustment index to predict mortality among veterans dually-enrolled in VA and medicare.
Healthcare (Amsterdam, Netherlands)(2019)
摘要
BACKGROUND:There is systemic undercoding of medical comorbidities within administrative claims in the Department of Veterans Affairs (VA). This leads to bias when applying claims-based risk adjustment indices to compare outcomes between VA and non-VA settings. Our objective was to compare the accuracy of a medication-based risk adjustment index (RxRisk-VM) to diagnostic claims-based indices for predicting mortality.
METHODS:We modified the RxRisk-V index (RxRisk-VM) by incorporating VA and Medicare pharmacy and durable medical equipment claims in Veterans dually-enrolled in VA and Medicare in 2012. Using the concordance (C) statistic, we compared its accuracy in predicting 1 and 3-year all-cause mortality to the following models: demographics only, demographics plus prescription count, or demographics plus a diagnostic claims-based risk index (e.g., Charlson, Elixhauser, or Gagne). We also compared models containing demographics, RxRisk-VM, and a claims-based index.
RESULTS:In our cohort of 271,184 dually-enrolled Veterans (mean age = 70.5 years, 96.1% male, 81.7% non-Hispanic white), RxRisk-VM (C = 0.773) exhibited greater accuracy in predicting 1-year mortality than demographics only (C = 0.716) or prescription counts (C = 0.744), but was less accurate than the Charlson (C = 0.794), Elixhauser (C = 0.80), or Gagne (C = 0.810) indices (all P < 0.001). Combining RxRisk-VM with claims-based indices enhanced its accuracy over each index alone (all models C ≥ 0.81). Relative model performance was similar for 3-year mortality.
CONCLUSIONS:The RxRisk-VM index exhibited a high level of, but slightly less, accuracy in predicting mortality in comparison to claims-based risk indices.
IMPLICATIONS:Its application may enhance the accuracy of studies examining VA and non-VA care and enable risk adjustment when diagnostic claims are not available or biased.
LEVEL OF EVIDENCE:Level 3.
更多查看译文
AI 理解论文
溯源树
样例
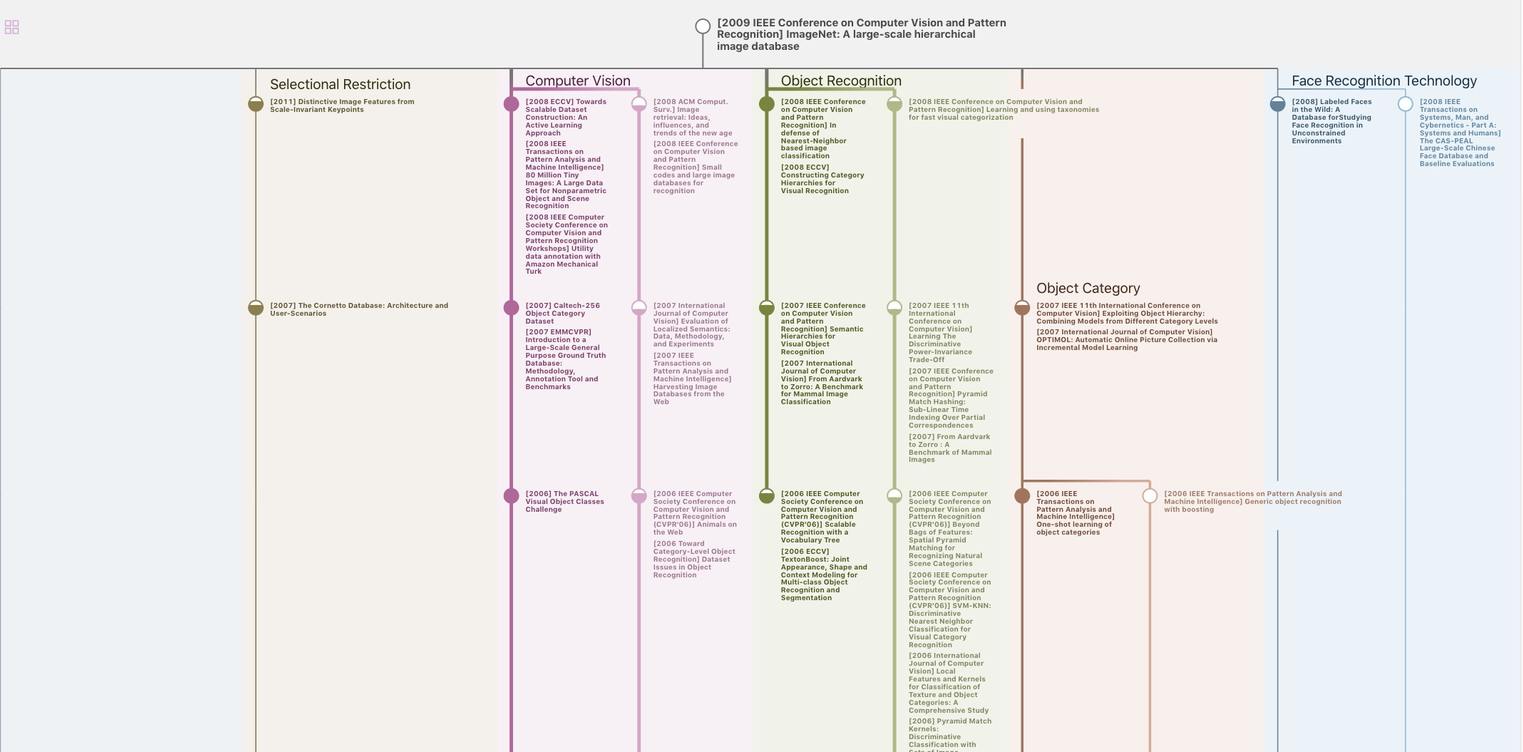
生成溯源树,研究论文发展脉络
Chat Paper
正在生成论文摘要