HIGAN: Cosmic Neutral Hydrogen with Generative Adversarial Networks.
CoRR(2019)
摘要
One of the most promising ways to observe the Universe is by detecting the 21cm emission from cosmic neutral hydrogen (HI) through radio-telescopes. Those observations can shed light on fundamental astrophysical questions only if accurate theoretical predictions are available. In order to maximize the scientific return of these surveys, those predictions need to include different observables and be precise on non-linear scales. Currently, one of the best ways to achieve this is via cosmological hydrodynamic simulations; however, the computational cost of these simulations is high -- tens of millions of CPU hours. In this work, we use Wasserstein Generative Adversarial Networks (WGANs) to generate new high-resolution ($35~h^{-1}{rm kpc}$) 3D realizations of cosmic HI at $z=5$. We do so by sampling from a 100-dimension manifold, learned by the generator, that characterizes the fully non-linear abundance and clustering of cosmic HI from the state-of-the-art simulation IllustrisTNG. We show that different statistical properties of the produced samples -- 1D PDF, power spectrum, bispectrum, and void size function -- match very well those of IllustrisTNG, and outperform state-of-the-art models such as Halo Occupation Distributions (HODs). Our WGAN samples reproduce the abundance of HI across 9 orders of magnitude, from the Ly$alpha$ forest to Damped Lyman Absorbers. WGAN can produce new samples orders of magnitude faster than hydrodynamic simulations.
更多查看译文
AI 理解论文
溯源树
样例
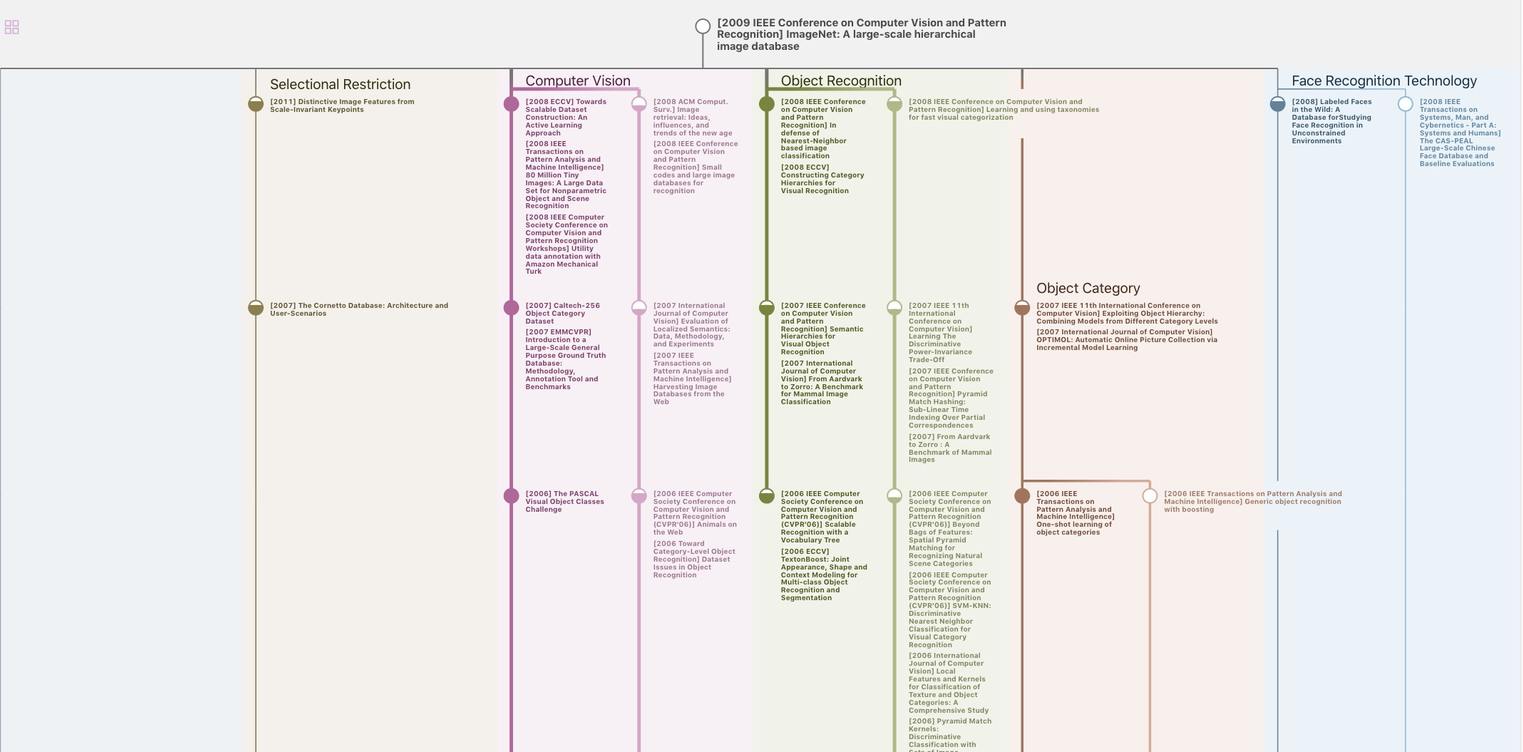
生成溯源树,研究论文发展脉络
Chat Paper
正在生成论文摘要