Accurate MapReduce Algorithms for $k$-median and $k$-means in General Metric Spaces
CoRR(2019)
摘要
Center-based clustering is a fundamental primitive for data analysis and becomes very challenging for large datasets. In this paper, we focus on the popular $k$-median and $k$-means variants which, given a set $P$ of points from a metric space and a parameter $ku003c|P|$, require to identify a set $S$ of $k$ centers minimizing respectively the sum of the distances and of the squared distances of all points in $P$ from their closest centers. Our specific focus is on general metric spaces for which it is reasonable to require that the centers belong to the input set (i.e., $S subseteq P$). We present coreset-based 2-round distributed approximation algorithms for the above problems using the MapReduce computational model. The algorithms are rather simple and obliviously adapt to the intrinsic complexity of the dataset, captured by the doubling dimension $D$ of the metric space. Remarkably, the algorithms attain approximation ratios that can be made arbitrarily close to those achievable by the best known polynomial-time sequential approximations, and they are very space efficient for small $D$, requiring local memories sizes substantially sublinear in the input size. To the best of our knowledge, no previous distributed approaches were able to attain similar quality-performance guarantees in general metric spaces.
更多查看译文
AI 理解论文
溯源树
样例
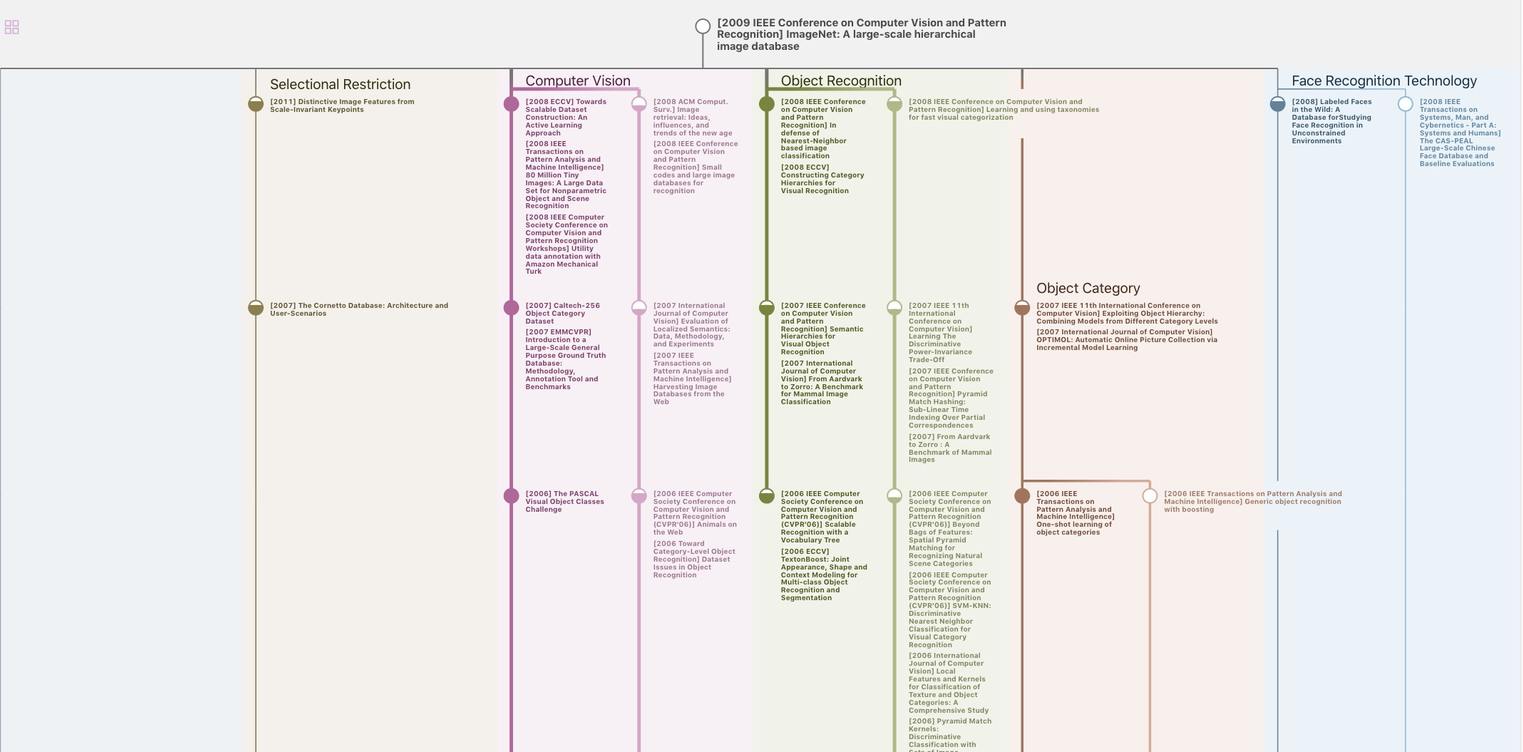
生成溯源树,研究论文发展脉络
Chat Paper
正在生成论文摘要