AutoSF: Searching Scoring Functions for Knowledge Graph Embedding
2020 IEEE 36th International Conference on Data Engineering (ICDE)(2020)
摘要
Scoring functions (SFs), which measure the plausibility of triplets in knowledge graph (KG), have become the crux of KG embedding. Lots of SFs, which target at capturing different kinds of relations in KGs, have been designed by humans in recent years. However, as relations can exhibit complex patterns that are hard to infer before training, none of them can consistently perform better than others on existing benchmark data sets. In this paper, inspired by the recent success of automated machine learning (AutoML), we propose to automatically design SFs (AutoSF) for distinct KGs by the AutoML techniques. However, it is non-trivial to explore domain-specific information here to make AutoSF efficient and effective. We firstly identify a unified representation over popularly used SFs, which helps to set up a search space for AutoSF. Then, we propose a greedy algorithm to search in such a space efficiently. The algorithm is further sped up by a filter and a predictor, which can avoid repeatedly training SFs with same expressive ability and help removing bad candidates during the search before model training. Finally, we perform extensive experiments on benchmark data sets. Results on link prediction and triplets classification show that the searched SFs by AutoSF, are KG dependent, new to the literature, and outperform the state-of- the-art SFs designed by humans.
更多查看译文
关键词
triplets classification,searched SFs,link prediction,model training,search space,domain-specific information,AutoML techniques,distinct KGs,automated machine learning,benchmark data sets,complex patterns,KG embedding,knowledge graph,searching scoring functions
AI 理解论文
溯源树
样例
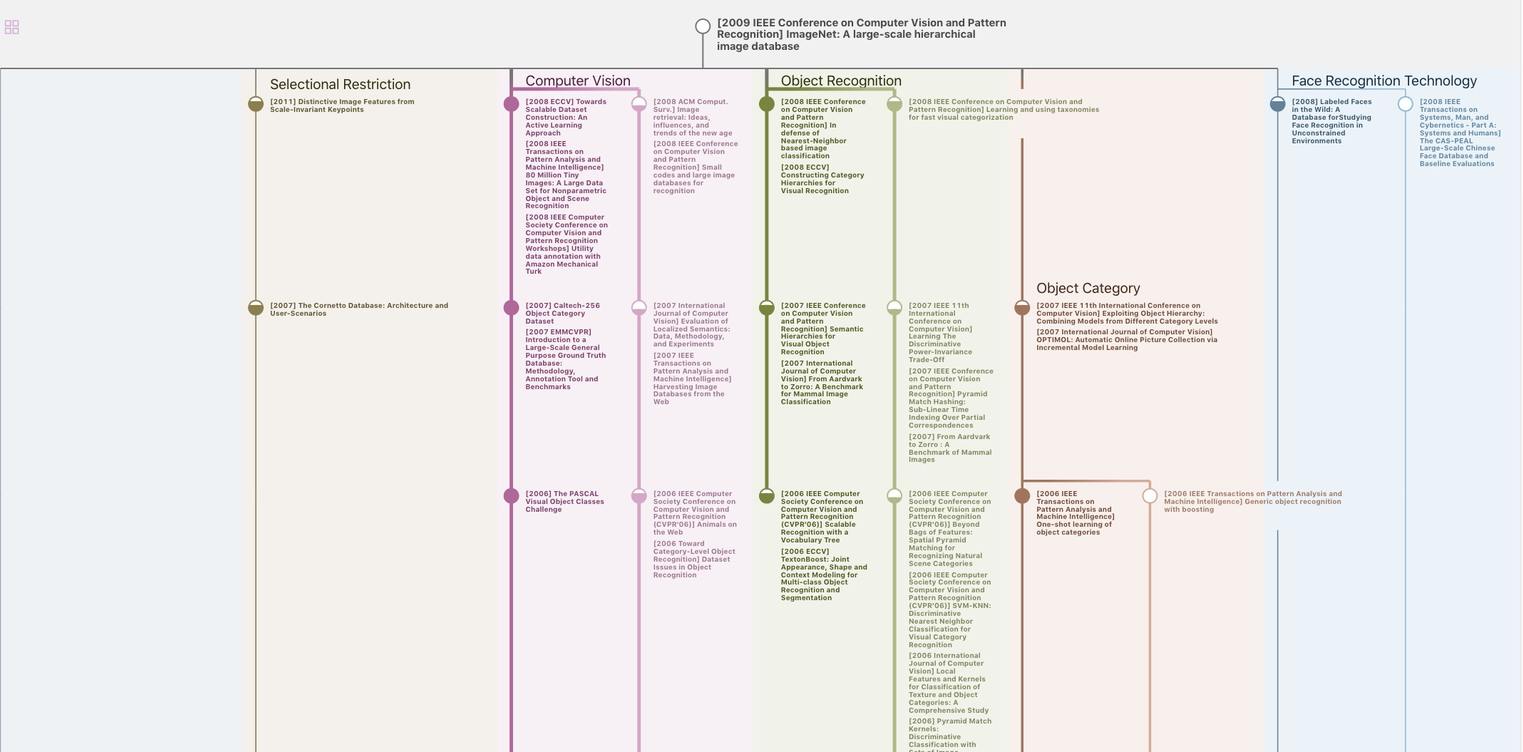
生成溯源树,研究论文发展脉络
Chat Paper
正在生成论文摘要