Bayesian variable selection for multioutcome models through shared shrinkage
SCANDINAVIAN JOURNAL OF STATISTICS(2021)
摘要
Variable selection over a potentially large set of covariates in a linear model is quite popular. In the Bayesian context, common prior choices can lead to a posterior expectation of the regression coefficients that is a sparse (or nearly sparse) vector with a few nonzero components, those covariates that are most important. This article extends the "global-local" shrinkage idea to a scenario where one wishes to model multiple response variables simultaneously. Here, we have developed a variable selection method for a K-outcome model (multivariate regression) that identifies the most important covariates across all outcomes. The prior for all regression coefficients is a mean zero normal with coefficient-specific variance term that consists of a predictor-specific factor (shared local shrinkage parameter) and a model-specific factor (global shrinkage term) that differs in each model. The performance of our modeling approach is evaluated through simulation studies and a data example.
更多查看译文
关键词
global-local shrinkage prior,multioutcome model,multivariate regression,shrinkage,variable selection
AI 理解论文
溯源树
样例
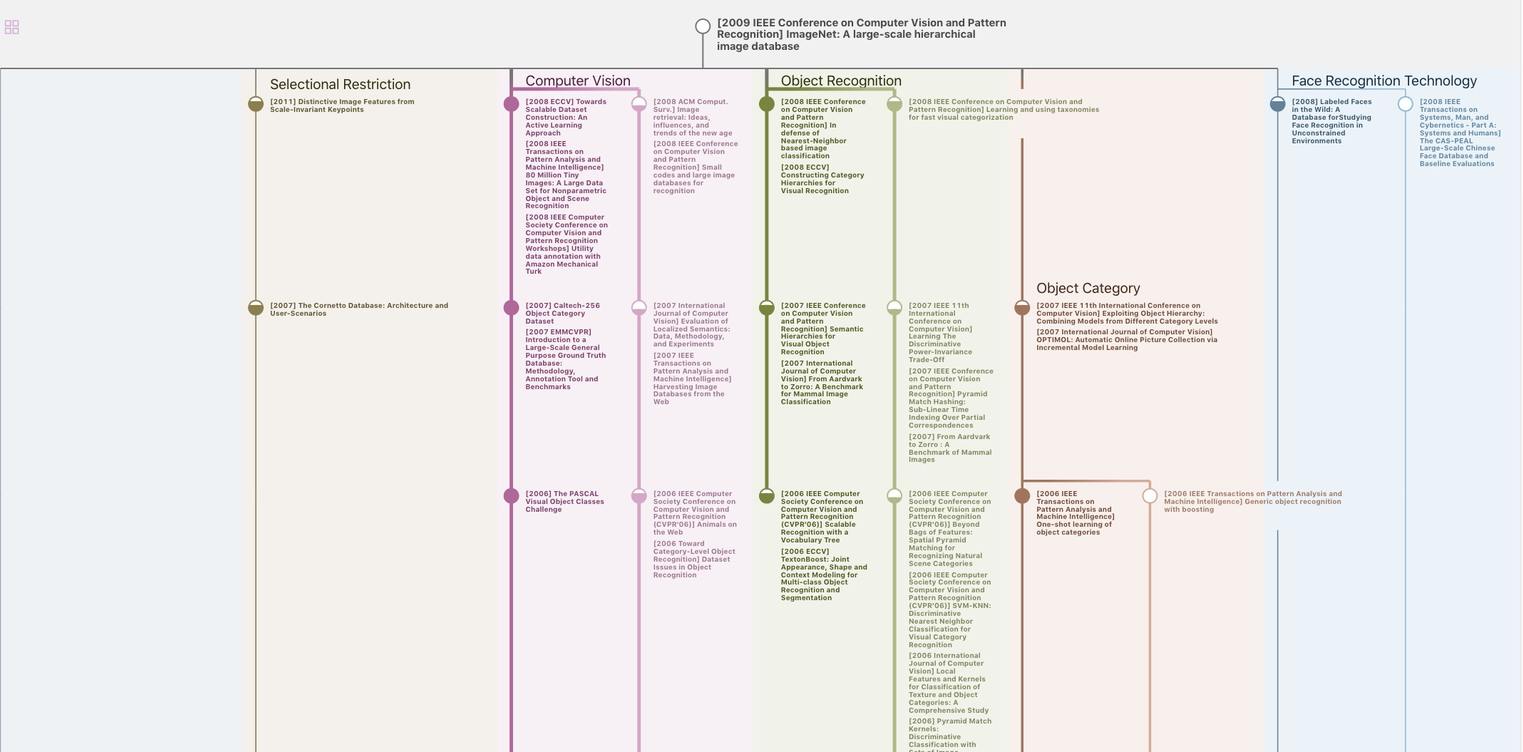
生成溯源树,研究论文发展脉络
Chat Paper
正在生成论文摘要