Recognizing Basal Cell Carcinoma on Smartphone-Captured Digital Histopathology Images with Deep Neural Network.
BRITISH JOURNAL OF DERMATOLOGY(2020)
摘要
Background Pioneering effort has been made to facilitate the recognition of pathology in malignancies based on whole-slide images (WSIs) through deep learning approaches. It remains unclear whether we can accurately detect and locate basal cell carcinoma (BCC) using smartphone-captured images. Objectives To develop deep neural network frameworks for accurate BCC recognition and segmentation based on smartphone-captured microscopic ocular images (MOIs). Methods We collected a total of 8046 MOIs, 6610 of which had binary classification labels and the other 1436 had pixelwise annotations. Meanwhile, 128 WSIs were collected for comparison. Two deep learning frameworks were created. The 'cascade' framework had a classification model for identifying hard cases (images with low prediction confidence) and a segmentation model for further in-depth analysis of the hard cases. The 'segmentation' framework directly segmented and classified all images. Sensitivity, specificity and area under the curve (AUC) were used to evaluate the overall performance of BCC recognition. Results The MOI- and WSI-based models achieved comparable AUCs around 0 center dot 95. The 'cascade' framework achieved 0 center dot 93 sensitivity and 0 center dot 91 specificity. The 'segmentation' framework was more accurate but required more computational resources, achieving 0 center dot 97 sensitivity, 0 center dot 94 specificity and 0 center dot 987 AUC. The runtime of the 'segmentation' framework was 15 center dot 3 +/- 3 center dot 9 s per image, whereas the 'cascade' framework took 4 center dot 1 +/- 1 center dot 4 s. Additionally, the 'segmentation' framework achieved 0 center dot 863 mean intersection over union. Conclusions Based on the accessible MOIs via smartphone photography, we developed two deep learning frameworks for recognizing BCC pathology with high sensitivity and specificity. This work opens a new avenue for automatic BCC diagnosis in different clinical scenarios. What's already known about this topic? The diagnosis of basal cell carcinoma (BCC) is labour intensive due to the large number of images to be examined, especially when consecutive slide reading is needed in Mohs surgery. Deep learning approaches have demonstrated promising results on pathological image-related diagnostic tasks. Previous studies have focused on whole-slide images (WSIs) and leveraged classification on image patches for detecting and localizing breast cancer metastases. What does this study add? Instead of WSIs, microscopic ocular images (MOIs) photographed from microscope eyepieces using smartphone cameras were used to develop neural network models for recognizing BCC automatically. The MOI- and WSI-based models achieved comparable areas under the curve around 0 center dot 95. Two deep learning frameworks for recognizing BCC pathology were developed with high sensitivity and specificity. Recognizing BCC through a smartphone could be considered a future clinical choice.
更多查看译文
关键词
basal cell carcinoma,deep convolutional neural network,digital histopathology images,disease diagnosis and localization
AI 理解论文
溯源树
样例
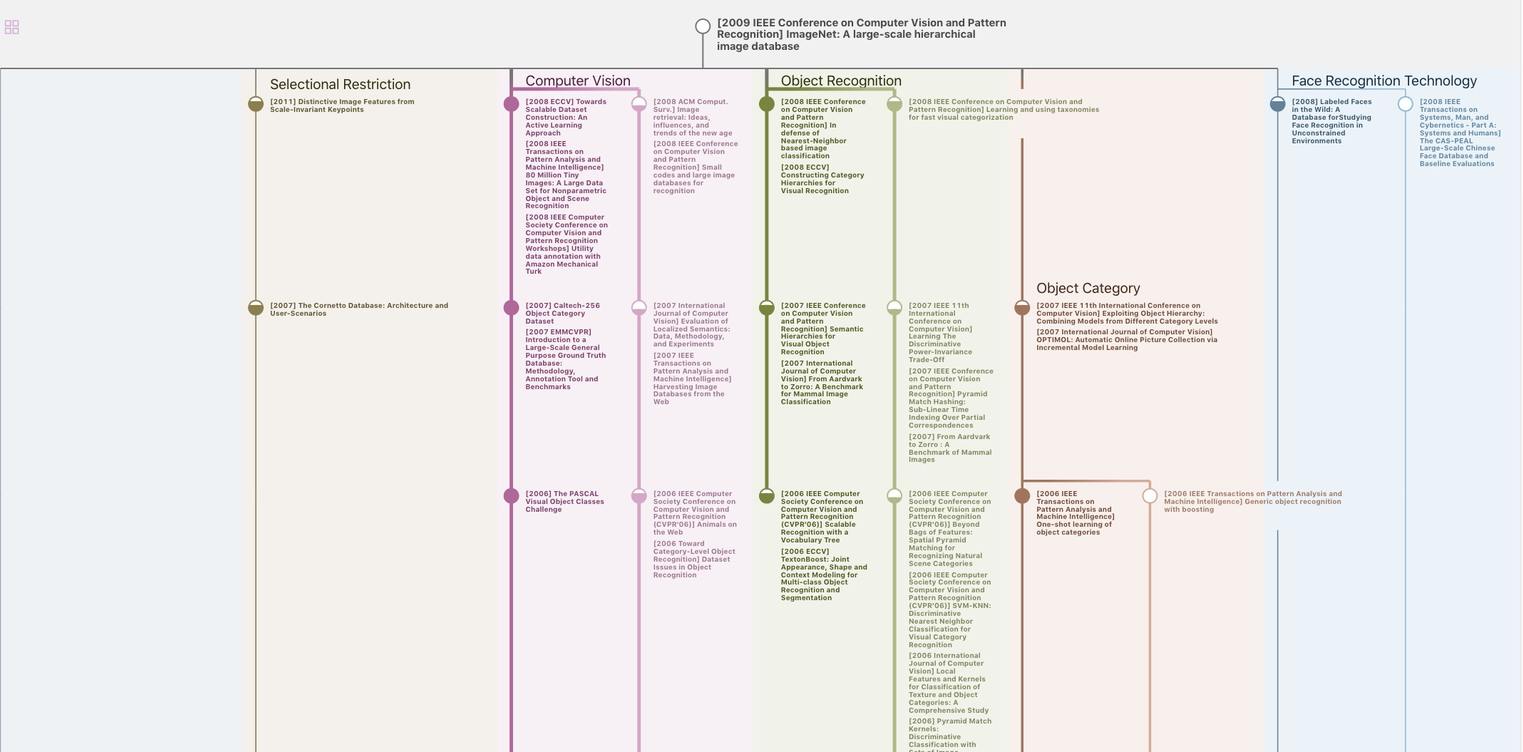
生成溯源树,研究论文发展脉络
Chat Paper
正在生成论文摘要