Using Surgeon Hand Motions to Predict Surgical Maneuvers.
HUMAN FACTORS(2019)
摘要
Objective: This study explores how common machine learning techniques can predict surgical maneuvers from a continuous video record of surgical benchtop simulations. Background: Automatic computer vision recognition of surgical maneuvers (suturing, tying, and transition) could expedite video review and objective assessment of surgeries. Method: We recorded hand movements of 37 clinicians performing simple and running subcuticular suturing benchtop simulations, and applied three machine learning techniques (decision trees, random forests, and hidden Markov models) to classify surgical maneuvers every 2 s (60 frames) of video. Results: Random forest predictions of surgical video correctly classified 74% of all video segments into suturing, tying, and transition states for a randomly selected test set. Hidden Markov model adjustments improved the random forest predictions to 79% for simple interrupted suturing on a subset of randomly selected participants. Conclusion: Random forest predictions aided by hidden Markov modeling provided the best prediction of surgical maneuvers. Training of models across all users improved prediction accuracy by 10% compared with a random selection of participants. Application: Marker-less video hand tracking can predict surgical maneuvers from a continuous video record with similar accuracy as robot-assisted surgical platforms, and may enable more efficient video review of surgical procedures for training and coaching.
更多查看译文
关键词
computer vision,marker-less hand tracking,machine learning,open surgeries,surgical skills
AI 理解论文
溯源树
样例
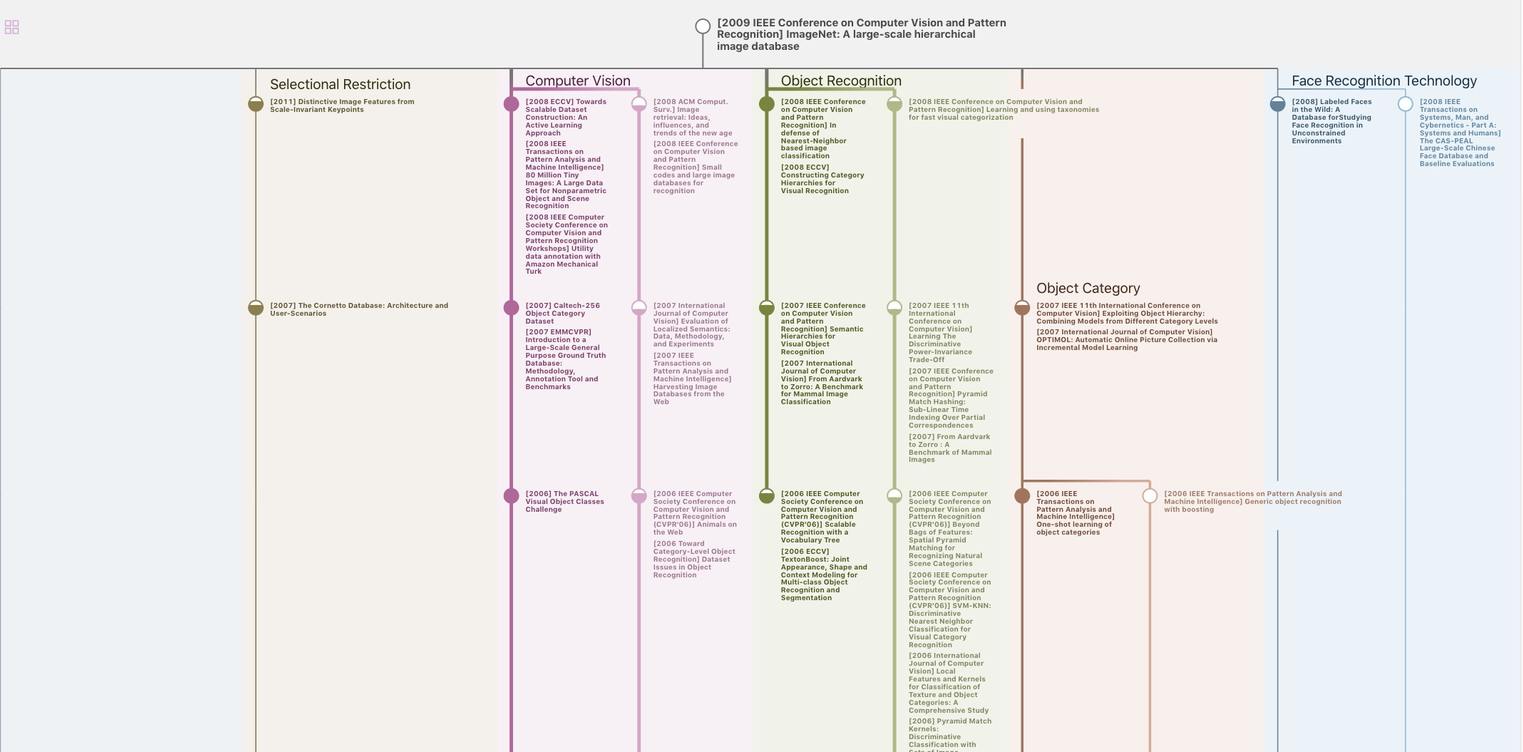
生成溯源树,研究论文发展脉络
Chat Paper
正在生成论文摘要