Disagreement-Based Active Learning in Online Settings
IEEE Transactions on Signal Processing(2022)
摘要
We study online active learning for classifying streaming instances within the framework of statistical learning theory. At each time, the learner either queries the label of the current instance or predicts the label based on past seen examples. The objective is to minimize the number of queries while constraining the number of prediction errors over a horizon of length T. We develop a disagreement-based online learning algorithm for a general hypothesis space and under the Tsybakov noise and establish its label complexity under a constraint of bounded regret in terms of classification errors. We further establish a matching (up to a poly-logarithmic factor) lower bound, demonstrating the order optimality of the proposed algorithm. We address the tradeoff between label complexity and regret and show that the algorithm can be modified to operate at a different point on the tradeoff curve.
更多查看译文
关键词
Active learning, label complexity, online learning, regret, statistical learning theory
AI 理解论文
溯源树
样例
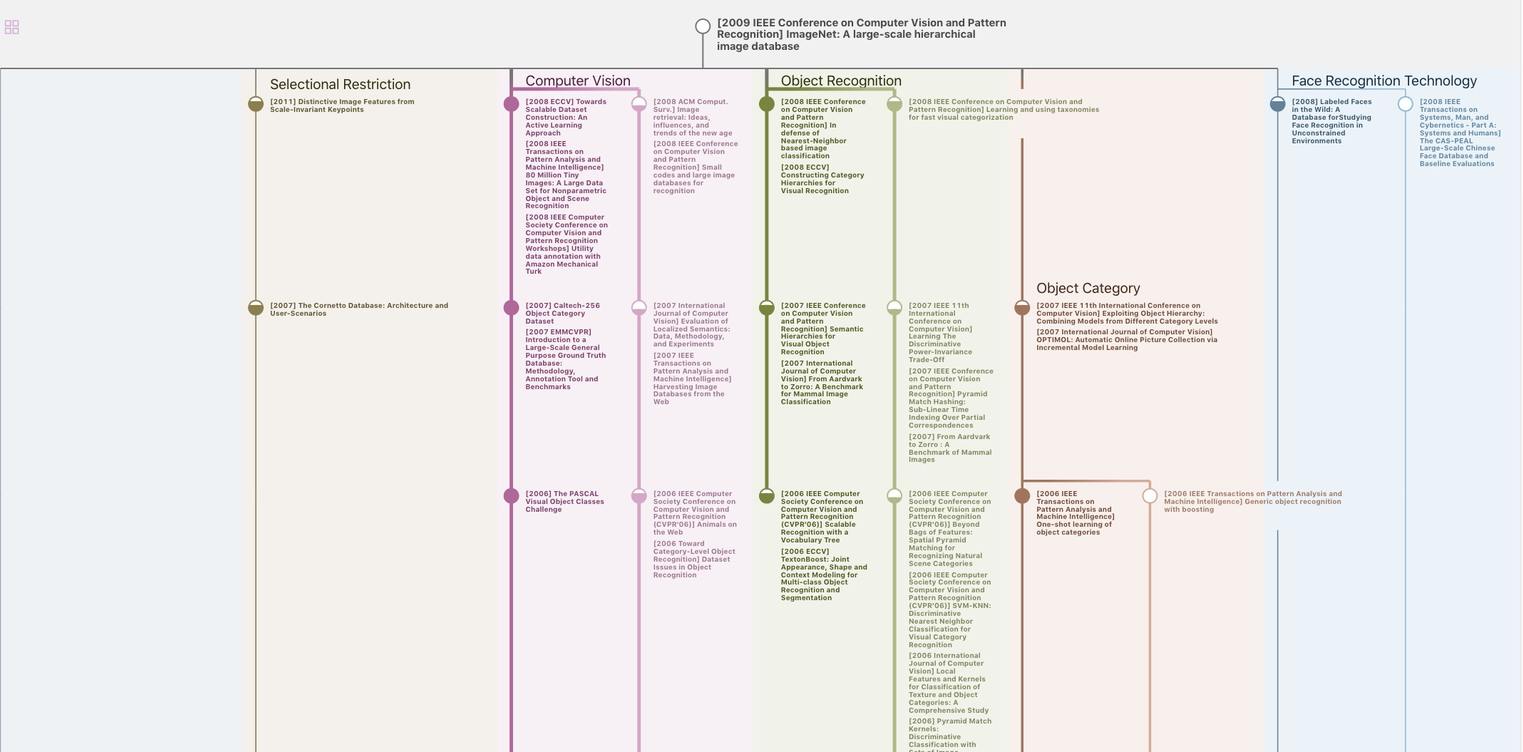
生成溯源树,研究论文发展脉络
Chat Paper
正在生成论文摘要