Generalized Transfer Matrix States From Artificial Neural Networks
PHYSICAL REVIEW B(2019)
Abstract
Identifying variational wave functions that efficiently parametrize the physically relevant states in the exponentially large Hilbert space is one of the key tasks towards solving the quantum many-body problem. Powerful tools in this context such as tensor network states have recently been complemented by states derived from artificial neural networks (ANNs). Here, we propose and investigate a new family of quantum states, coined generalized transfer matrix states (GTMS), which bridges between the two mentioned approaches in the framework of deep ANNs. In particular, we show by means of a constructive embedding that the class of GTMS contains generic matrix product states while at the same time being capable of capturing more long-ranged quantum correlations that go beyond the area-law entanglement properties of tensor networks. While the state amplitude of generic deep ANNs cannot be exactly evaluated, that of a GTMS network can be analytically computed using transfer matrix methods. With numerical simulations, we demonstrate how the GTMS network learns random matrix product states in a supervised learning scheme, and how augmenting the network by long-ranged couplings leads to the onset of volume-law entanglement scaling. By means of an explicit example using variational Monte Carlo, we also show that GTMS can parametrize critical quantum many-body ground states to a good accuracy. Our findings suggest that GTMS are a promising candidate for the study of critical and dynamical quantum many-body systems.
MoreTranslated text
Key words
Matrix Product States
AI Read Science
Must-Reading Tree
Example
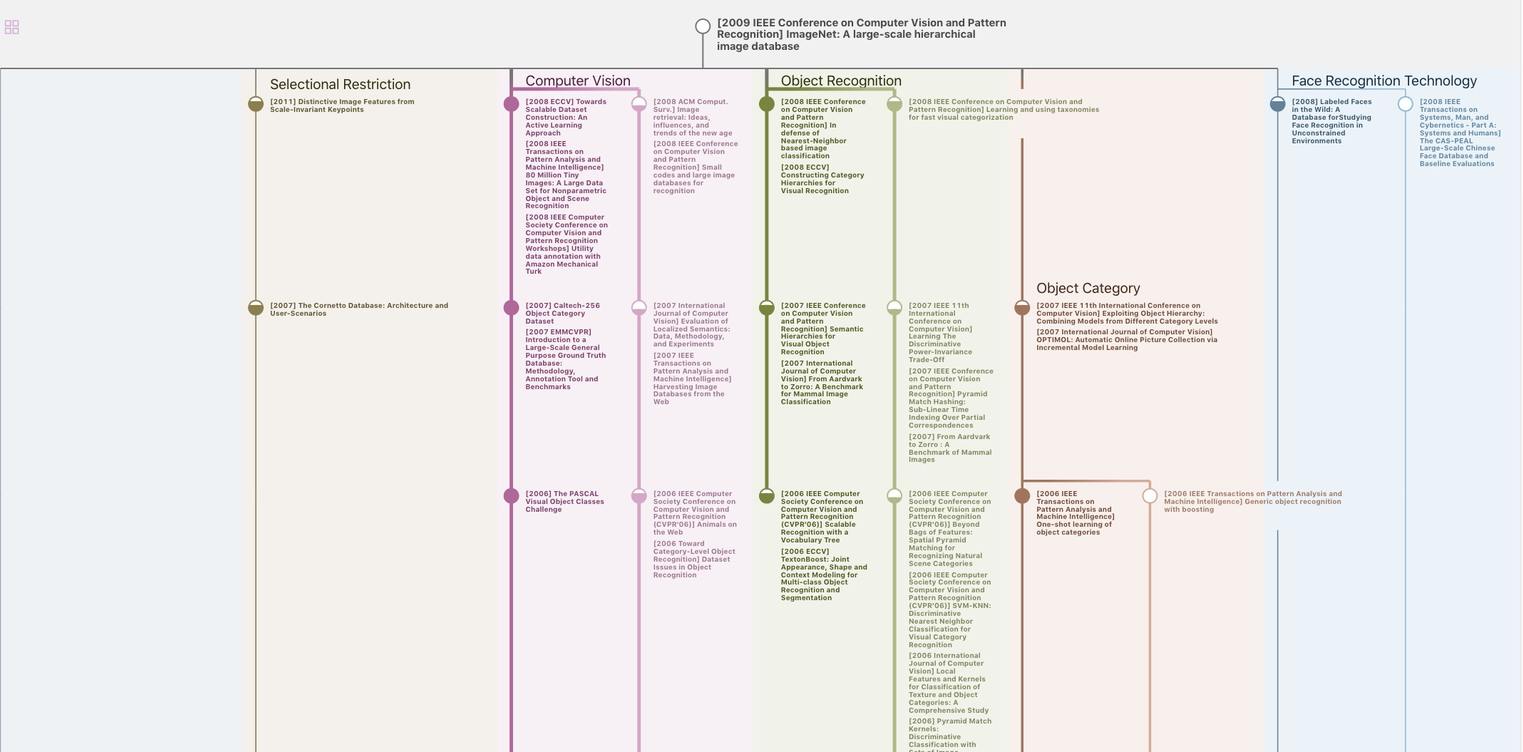
Generate MRT to find the research sequence of this paper
Chat Paper
Summary is being generated by the instructions you defined