Comparative Study of Back Propagation Artificial Neural Networks and Logistic Regression Model in Predicting Poor Prognosis after Acute Ischemic Stroke.
OPEN MEDICINE(2019)
摘要
Objective: To investigate the predictive value of clinical variables on the poor prognosis at 90-day follow-up from acute stroke onset, and compare the diagnostic performance between back propagation artificial neural networks (BP ANNs) and Logistic regression (LR) models in predicting the prognosis. Methods: We studied the association between clinical variables and the functional recovery of 435 acute ischemic stroke patients. The patients were divided into 2 groups according to modified Rankin Scale scores evaluated on the 90th day after stroke onset. Both BP ANNs and LR models were established for predicting the poor outcome and their diagnostic performance were compared by receiver operating curve. Results: Age, free fatty acid, homocysteine and alkaline phosphatase were closely related with the poor outcome in acute ischemic stroke patients and finally enrolled in models. The accuracy, sensitivity and specificity of BP ANNs were 80.15%, 75.64% and 82.07% respectively. For the LR model, the accuracy, sensitivity and specificity was 70.61%, 88.46% and 63.04% respectively. The area under the ROC curve of the BP ANNs and LR model was 0.881and 0.809. Conclusions: Both BP ANNs and LR model were promising for the prediction of poor outcome by combining age, free fatty acid, homocysteine and alkaline phosphatase. However, BP ANNs model showed better performance than LR model in predicting the prognosis.
更多查看译文
关键词
Back propagation artificial neural networks,Logistic regression model,Acute ischemic stroke,Poor outcome,Clinical variables
AI 理解论文
溯源树
样例
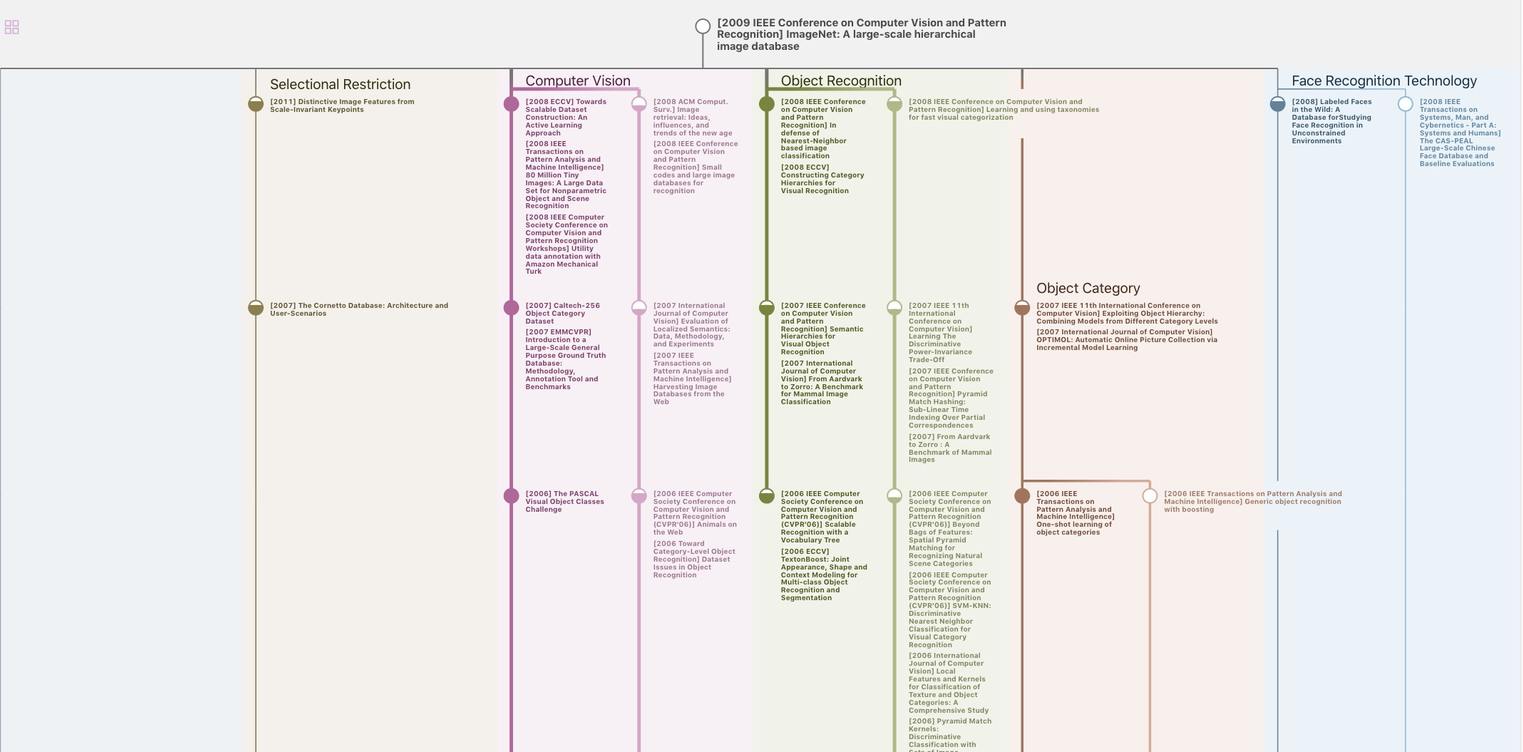
生成溯源树,研究论文发展脉络
Chat Paper
正在生成论文摘要