Synthesis and texture manipulation of screening mammograms using conditional generative adversarial network.
Proceedings of SPIE(2019)
摘要
Annotated data availability has always been a major limiting f actor for the development of algorithms in the field of computer aided diagnosis. The purpose of this study is to investigate the feasibility of using a conditional generative adversarial network (GAN) to synthesize high resolution mammography images with semantic control. We feed a binary mammographic texture map to the generator to synthesize a full-field digital-mammogram (FFDM). Our results show the generator quickly learned to grow anatomical details around the edges within the texture mask. However, we found the training unstable and the quality of generated images unsatisfactory due to the inherent limitation of latent space and sample space mapping by the pix2pix framework. In order to synthesize high resolution mammography images with semantic control, we identified the critical challenge is to build the efficient mappings of binary textures with a great variety of pattern realizations with the image domain.
更多查看译文
关键词
conditional generative adversarial network,data augmentation,deep learning
AI 理解论文
溯源树
样例
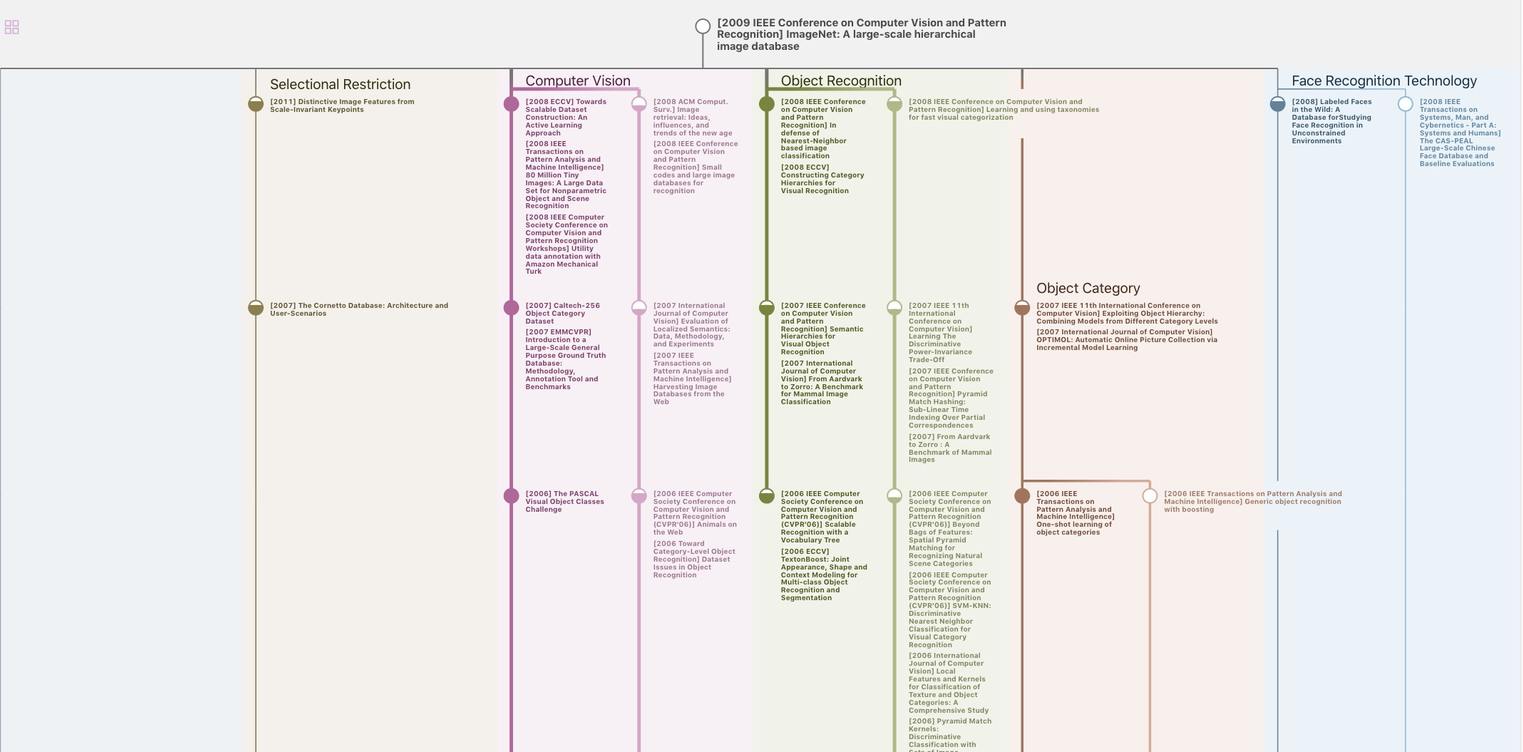
生成溯源树,研究论文发展脉络
Chat Paper
正在生成论文摘要