A Unified Neural Architecture for Instrumental Audio Tasks
ICASSP 2019 - 2019 IEEE International Conference on Acoustics, Speech and Signal Processing (ICASSP)(2019)
摘要
Within Music Information Retrieval (MIR), prominent tasks — including pitch-tracking, source-separation, super-resolution, and synthesis — typically call for specialised methods, despite their similarities. Conditional Generative Adversarial Networks (cGANs) have been shown to be highly versatile in learning general image-to-image translations, but have not yet been adapted across MIR. In this work, we present an end-to-end supervisable architecture to perform all aforementioned audio tasks, consisting of a WaveNet synthesiser conditioned on the output of a jointly-trained cGAN spectrogram translator. In doing so, we demonstrate the potential of such flexible techniques to unify MIR tasks, promote efficient transfer learning, and converge research to the improvement of powerful, general methods. Finally, to the best of our knowledge, we present the first application of GANs to guided instrument synthesis.
更多查看译文
关键词
Task analysis,Spectrogram,Instruments,Computer architecture,Image resolution,Generative adversarial networks,Harmonic analysis
AI 理解论文
溯源树
样例
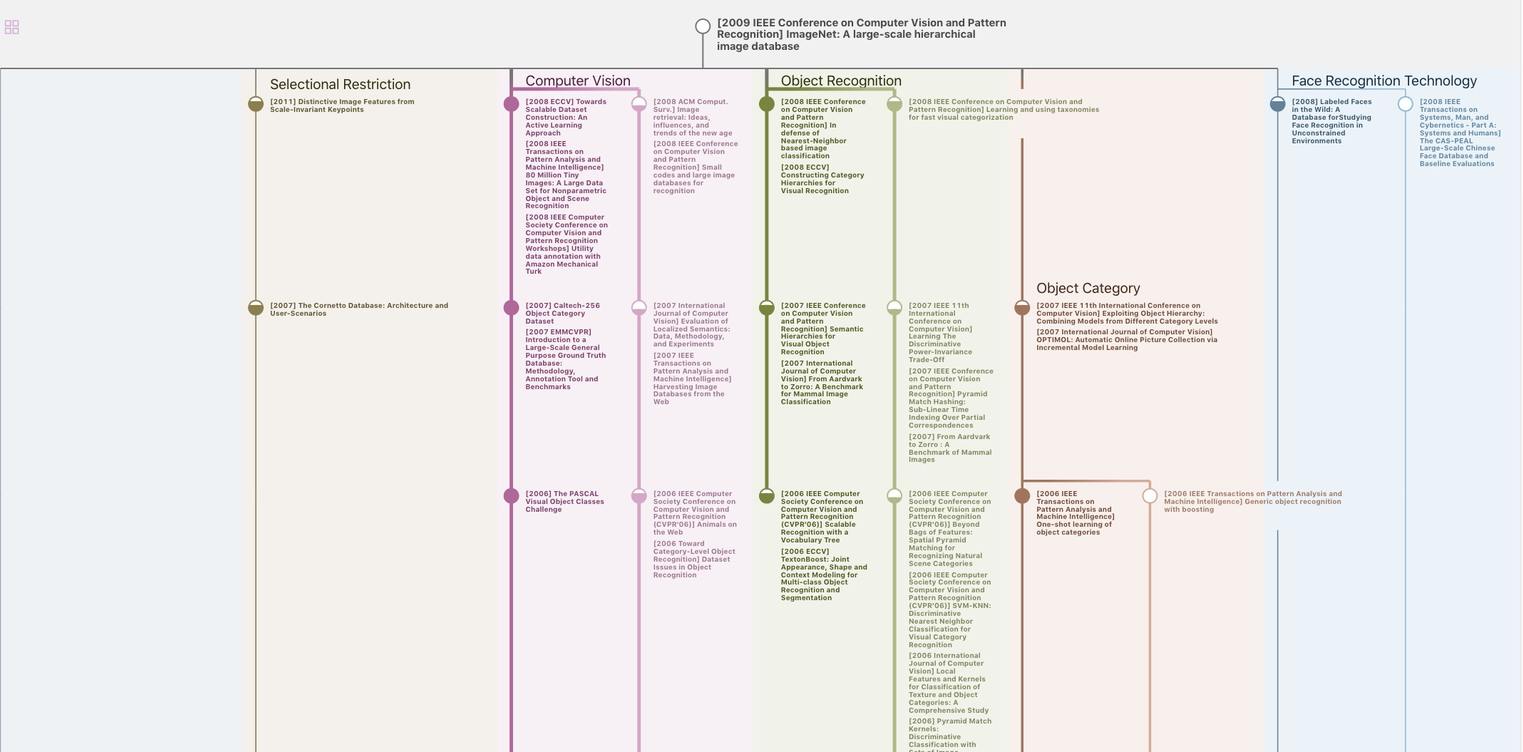
生成溯源树,研究论文发展脉络
Chat Paper
正在生成论文摘要