Designing lightweight deep learning models for echocardiography view classification.
Proceedings of SPIE(2019)
摘要
Transthoracic echocardiography (echo) is the most common imaging modality for diagnosis of cardiac conditions. Echo is acquired from a multitude of views, each of which distinctly highlights specific regions of the heart anatomy. In this paper, we present an approach based on knowledge distillation to obtain a highly accurate lightweight deep learning model for classification of 12 standard echocardiography views. The knowledge of several deep learning architectures based on the three common state-of-the-art architectures, VGG-16, DenseNet, and Resnet, are distilled to train a set of lightweight models. Networks were developed and evaluated using a dataset of 16,612 echo cines obtained from 3,151 unique patients across several ultrasound imaging machines. The best accuracy of 89.0% is achieved by an ensemble of the three very deep models while we show an ensemble of lightweight models has a comparable accuracy of 88.1%. The lightweight models have approximately 1% of the very deep model parameters and are six times faster in run-time. Such lightweight view classification models could be used to build fast mobile applications for real-time point-of-care ultrasound diagnosis.
更多查看译文
关键词
Echocardiography,View classification,View detection,Convolutional neural networks,Deep learning,Knowledge distillation
AI 理解论文
溯源树
样例
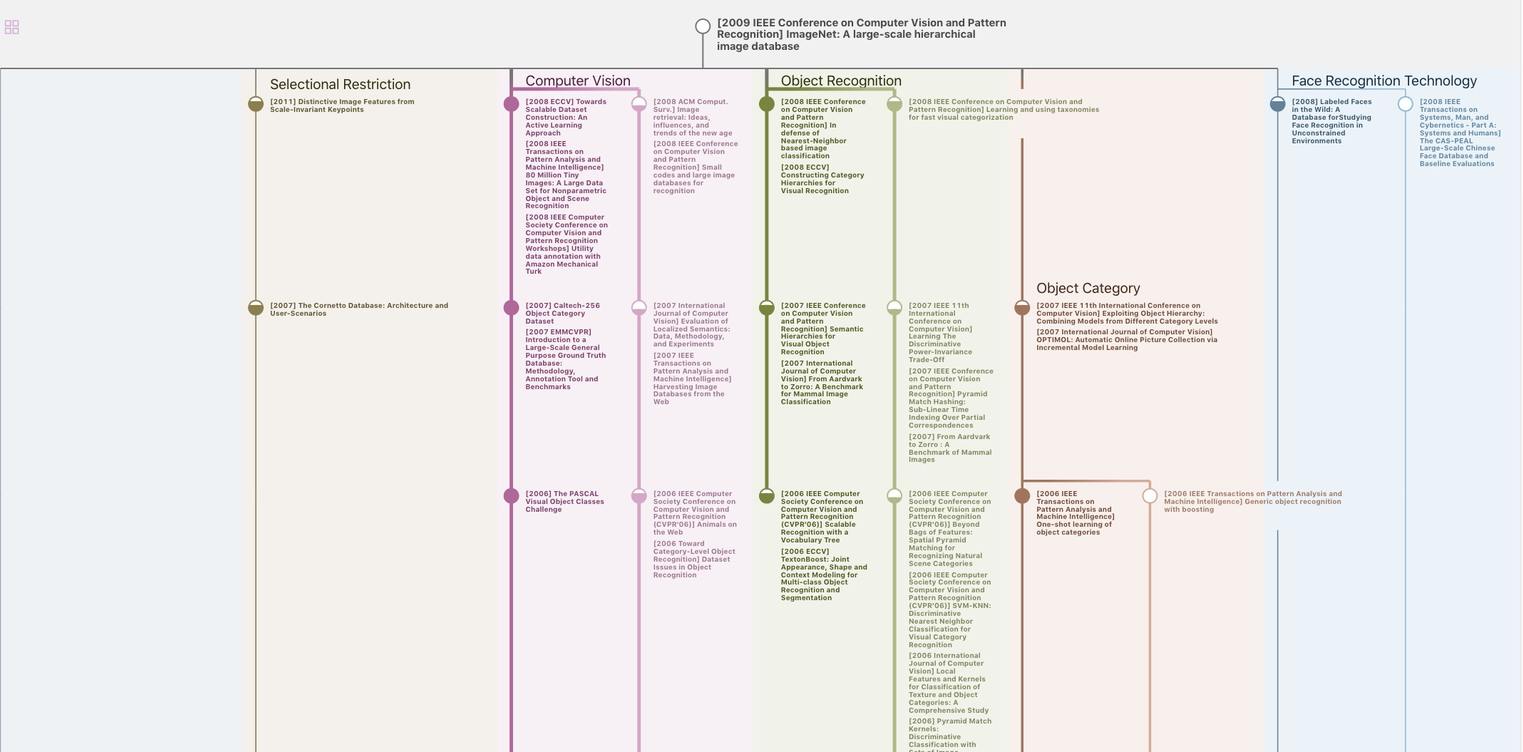
生成溯源树,研究论文发展脉络
Chat Paper
正在生成论文摘要