Transformable Bottleneck Networks
2019 IEEE/CVF INTERNATIONAL CONFERENCE ON COMPUTER VISION (ICCV 2019)(2019)
摘要
We propose a novel approach to performing fine-grained 3D manipulation of image content via a convolutional neural network, which we call the Transformable Bottleneck Network (TBN). It applies given spatial transformations directly to a volumetric bottleneck within our encoder-bottleneck-decoder architecture. Multi-view supervision encourages the network to learn to spatially disentangle the feature space within the bottleneck. The resulting spatial structure can be manipulated with arbitrary spatial transformations. We demonstrate the efficacy of TBNs for novel view synthesis, achieving state-of-the-art results on a challenging benchmark. We demonstrate that the bottlenecks produced by networks trained for this task contain meaningful spatial structure that allows us to intuitively perform a variety of image manipulations in 3D, well beyond the rigid transformations seen during training. These manipulations include non-uniform scaling, non-rigid warping, and combining content from different images. Finally, we extract explicit 3D structure from the bottleneck, performing impressive 3D reconstruction from a single input image.(1)
更多查看译文
关键词
spatial structure,arbitrary spatial transformations,image manipulations,explicit 3D structure,fine-grained 3D manipulation,image content,convolutional neural network,volumetric bottleneck,encoder-bottleneck-decoder architecture,multiview supervision,transformable bottleneck network
AI 理解论文
溯源树
样例
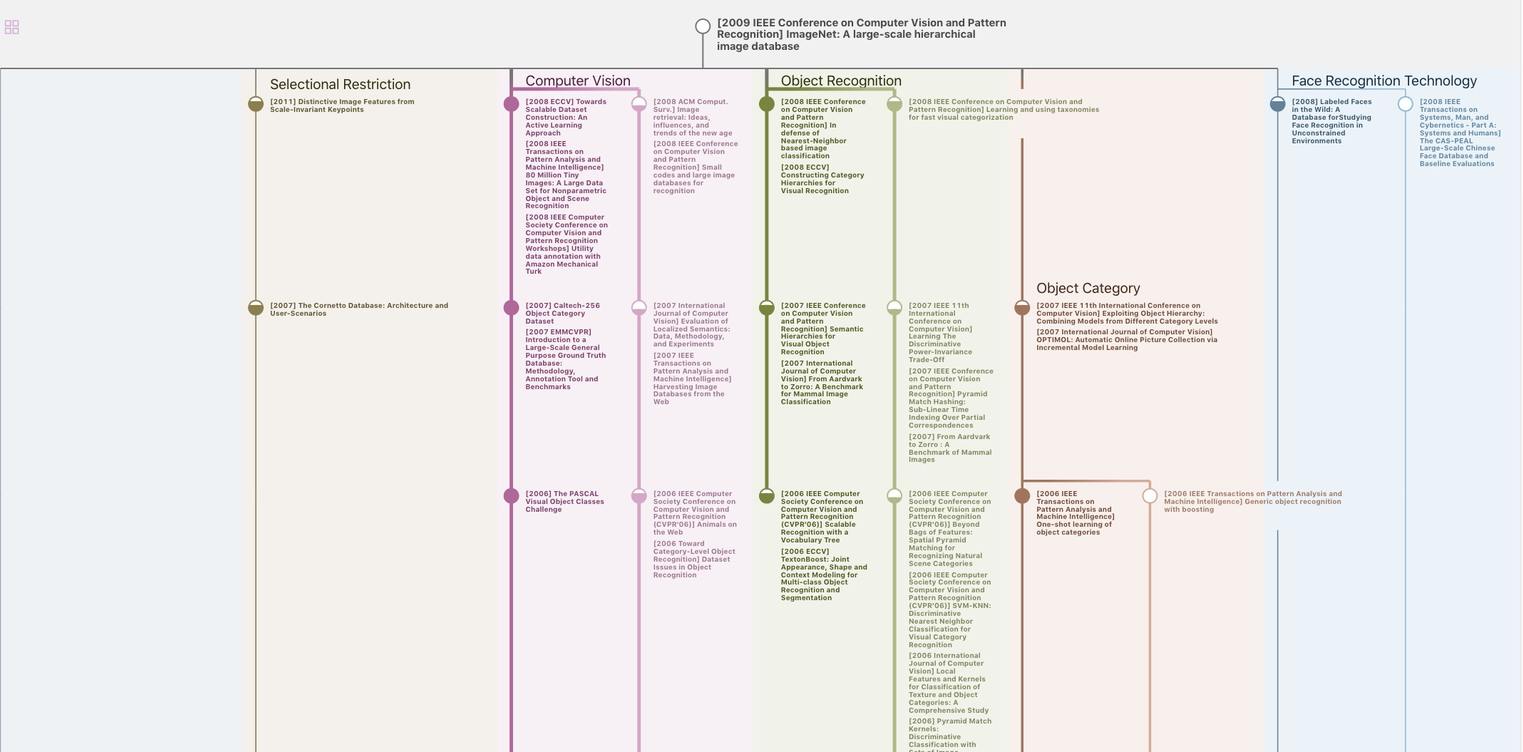
生成溯源树,研究论文发展脉络
Chat Paper
正在生成论文摘要