Rnn-Based Counterfactual Prediction, With An Application To Homestead Policy And Public Schooling
JOURNAL OF THE ROYAL STATISTICAL SOCIETY SERIES C-APPLIED STATISTICS(2021)
Abstract
This paper proposes a method for estimating the effect of a policy intervention on an outcome over time. We train recurrent neural networks (RNNs) on the history of control unit outcomes to learn a useful representation for predicting future outcomes. The learned representation of control units is then applied to the treated units for predicting counterfactual outcomes. RNNs are specifically structured to exploit temporal dependencies in panel data and are able to learn negative and non-linear interactions between control unit outcomes. We apply the method to the problem of estimating the long-run impact of US homestead policy on public school spending.
MoreTranslated text
Key words
counterfactual prediction, panel data, political economy, recurrent neural networks, synthetic controls
AI Read Science
Must-Reading Tree
Example
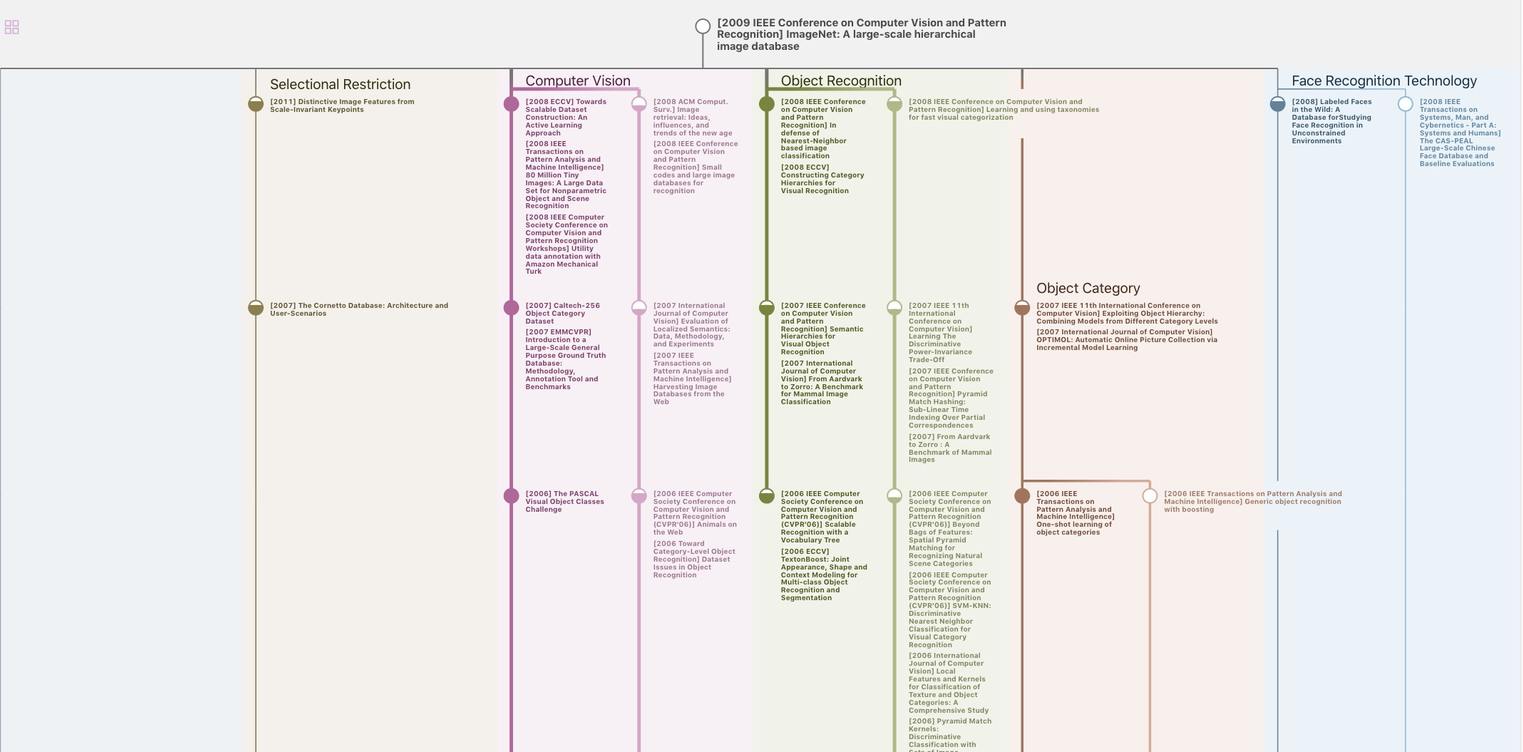
Generate MRT to find the research sequence of this paper
Chat Paper
Summary is being generated by the instructions you defined