QoS-Aware Power Management with Deep Learning.
Immunotechnology(2019)
Key words
Deep learning,power management,feedforward neural networks,QoS-aware,wireless communications
AI Read Science
Must-Reading Tree
Example
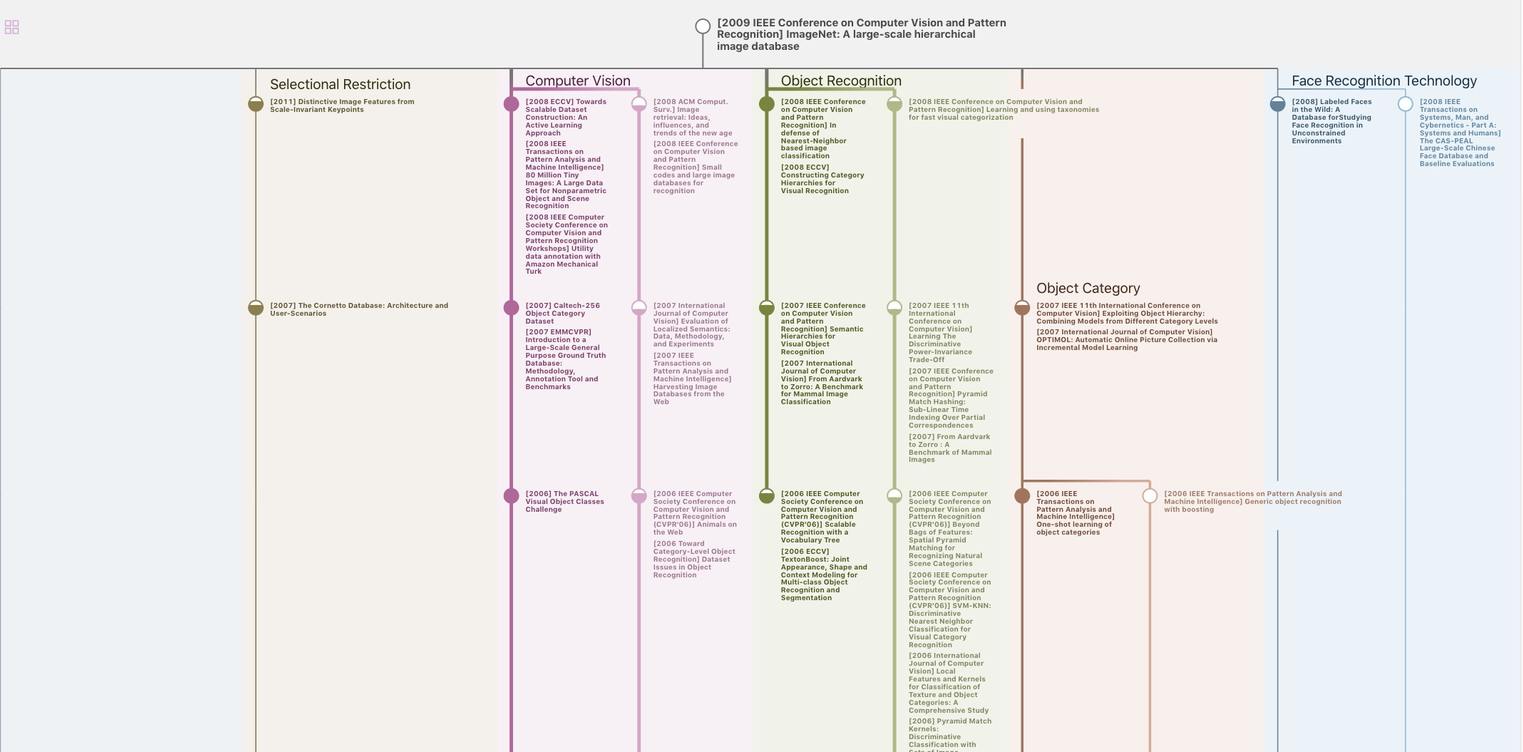
Generate MRT to find the research sequence of this paper
Chat Paper
Summary is being generated by the instructions you defined