Moment Matching for Multi-Source Domain Adaptation
2019 IEEE/CVF INTERNATIONAL CONFERENCE ON COMPUTER VISION (ICCV 2019)(2019)
摘要
Conventional unsupervised domain adaptation (UDA) assumes that training data are sampled from a single domain. This neglects the more practical scenario where training data are collected from multiple sources, requiring multi-source domain adaptation. We make three major contributions towards addressing this problem. First, we collect and annotate by far the largest UDA dataset, called DomainNet, which contains six domains and about 0.6 million images distributed among 345 categories, addressing the gap in data availability for multi-source UDA research. Second, we propose a new deep learning approach, Moment Matching for Multi-Source Domain Adaptation (M3SDA), which aims to transfer knowledge learned from multiple labeled source domains to an unlabeled target domain by dynamically aligning moments of their feature distributions. Third, we provide new theoretical insights specifically for moment matching approaches in both single and multiple source domain adaptation. Extensive experiments are conducted to demonstrate the power of our new dataset in benchmarking state-of-the-art multi-source domain adaptation methods, as well as the advantage of our proposed model. Dataset and Code are available at http://ai.bu.edu/M3SDA/.
更多查看译文
关键词
multisource UDA,moment matching,multiple labeled source domains,unlabeled target domain,single source domain adaptation,multiple source domain adaptation,unsupervised domain adaptation,multisource domain adaptation methods,DomainNet,data availability,deep learning approach
AI 理解论文
溯源树
样例
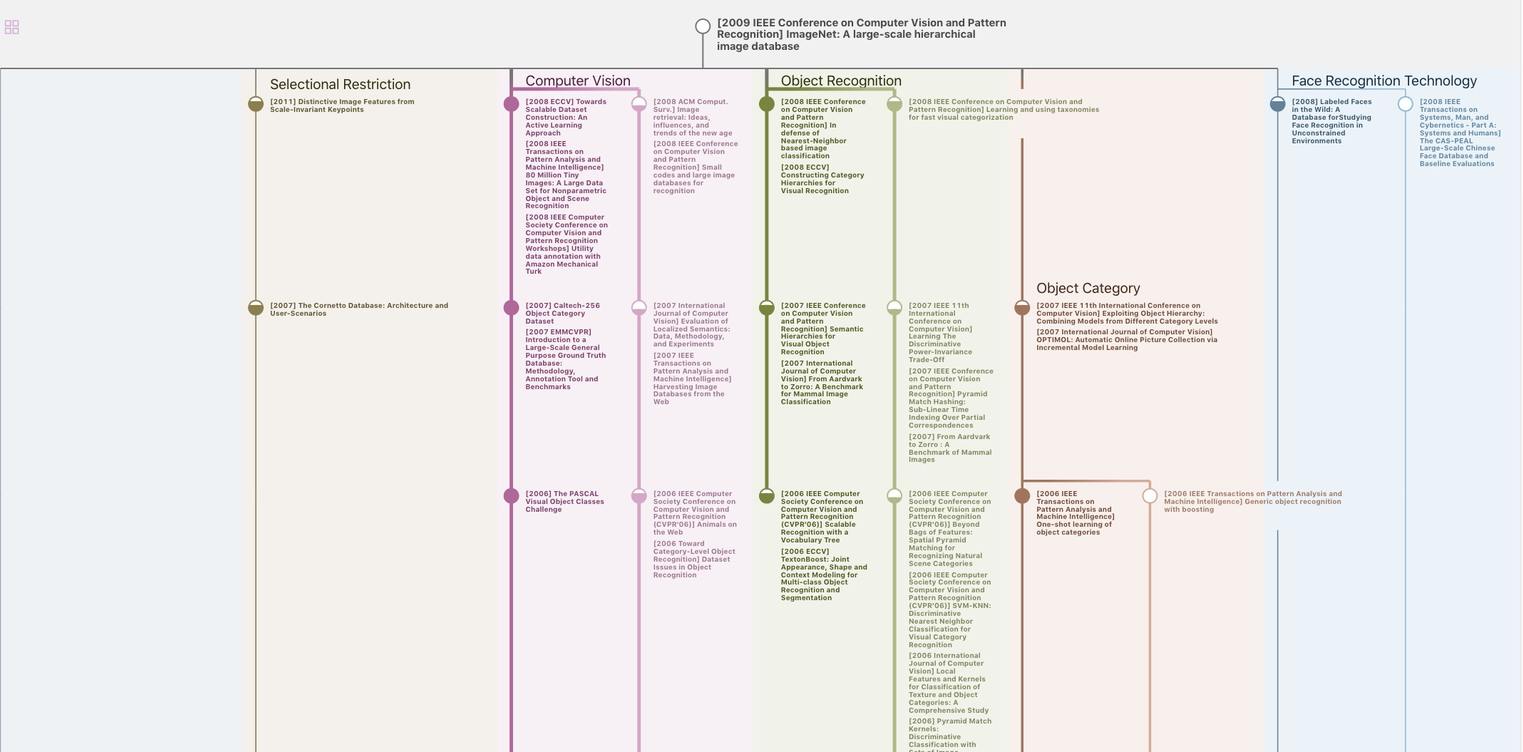
生成溯源树,研究论文发展脉络
Chat Paper
正在生成论文摘要