Adaptive Sequential Machine Learning
SEQUENTIAL ANALYSIS-DESIGN METHODS AND APPLICATIONS(2019)
摘要
A framework previously introduced in Wilson et al. (2018) for solving a sequence of stochastic optimization problems with bounded changes in the minimizers is extended and applied to machine learning problems such as regression and classification. The stochastic optimization problems arising in these machine learning problems are solved using algorithms such as stochastic gradient descent (SGD). A method based on estimates of the change in the minimizers and properties of the optimization algorithm is introduced for adaptively selecting the number of samples at each time step to ensure that the excess risk-that is, the expected gap between the loss achieved by the approximate minimizer produced by the optimization algorithm and the exact minimizer-does not exceed a target level. A bound is developed to show that the estimate of the change in the minimizers is non trivial provided that the excess risk is small enough. Extensions relevant to the machine learning setting are considered, including a cost-based approach to select the number of samples with a cost budget over a fixed horizon, and an approach to applying cross-validation for model selection. Finally, experiments with synthetic and real data are used to validate the algorithms.
更多查看译文
关键词
Adaptive learning,cross-validation,excess risk,sequential learning,stochastic gradient descent
AI 理解论文
溯源树
样例
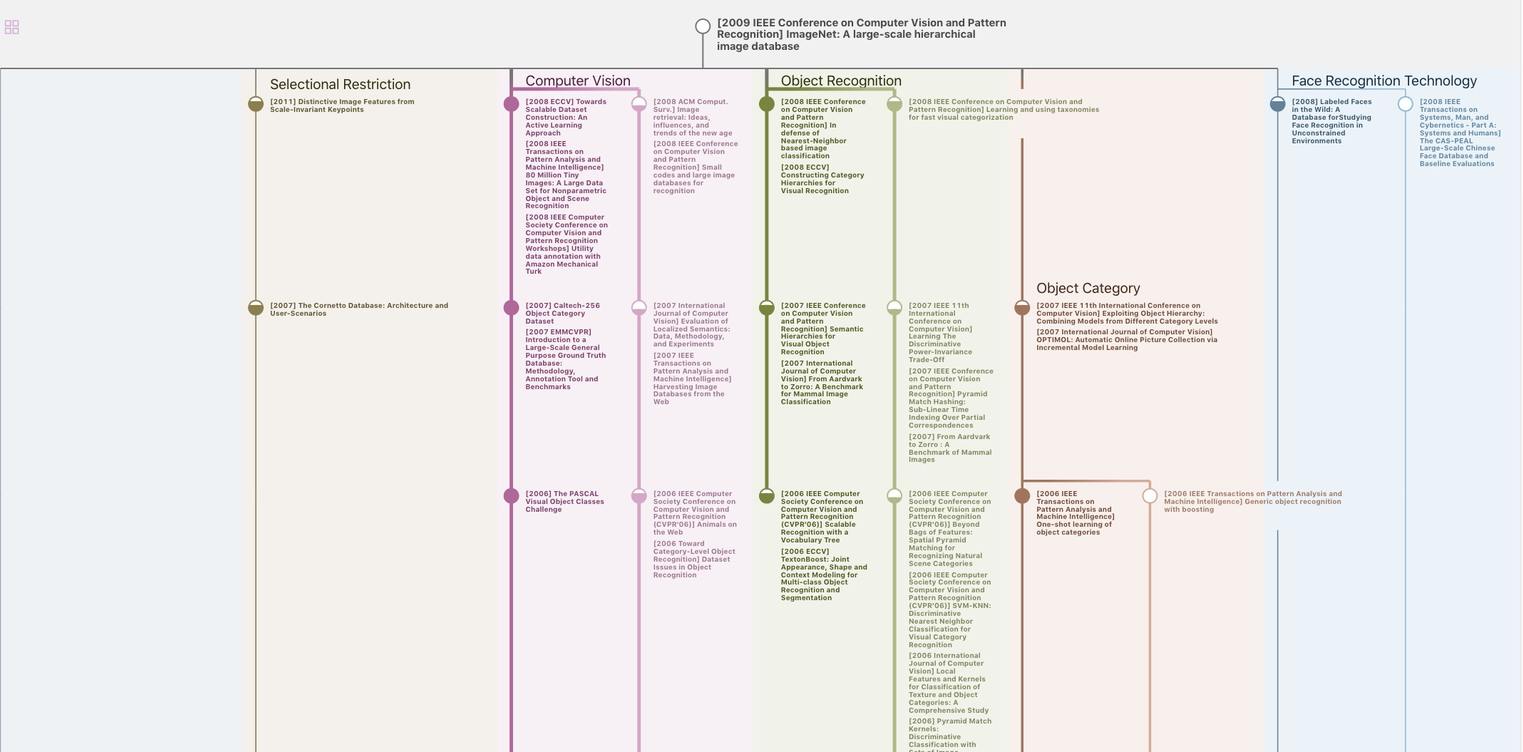
生成溯源树,研究论文发展脉络
Chat Paper
正在生成论文摘要