Progressive Ensemble Networks For Zero-Shot Recognition
2019 IEEE/CVF CONFERENCE ON COMPUTER VISION AND PATTERN RECOGNITION (CVPR 2019)(2019)
Abstract
Despite the advancement of supervised image recognition algorithms, their dependence on the availability of labeled data and the rapid expansion of image categories raise the significant challenge of zero-shot learning. Zero-shot learning (ZSL) aims to transfer knowledge from labeled classes into unlabeled classes to reduce human labeling effort. In this paper, we propose a novel progressive ensemble network model with multiple projected label embeddings to address zero-shot image recognition. The ensemble network is built by learning multiple image classification functions with a shared feature extraction network but different label embedding representations, which enhance the diversity of the classifiers and facilitate information transfer to unlabeled classes. A progressive training framework is then deployed to gradually label the most confident images in each unlabeled class with predicted pseudo-labels and update the ensemble network with the training data augmented by the pseudo-labels. The proposed model performs training on both labeled and unlabeled data. It can naturally bridge the domain shift problem in visual appearances and be extended to the generalized zero-shot learning scenario. We conduct experiments on multiple ZSL datasets and the empirical results demonstrate the efficacy of the proposed model.
MoreTranslated text
Key words
Statistical Learning,Recognition: Detection,Categorization,Retrieval
AI Read Science
Must-Reading Tree
Example
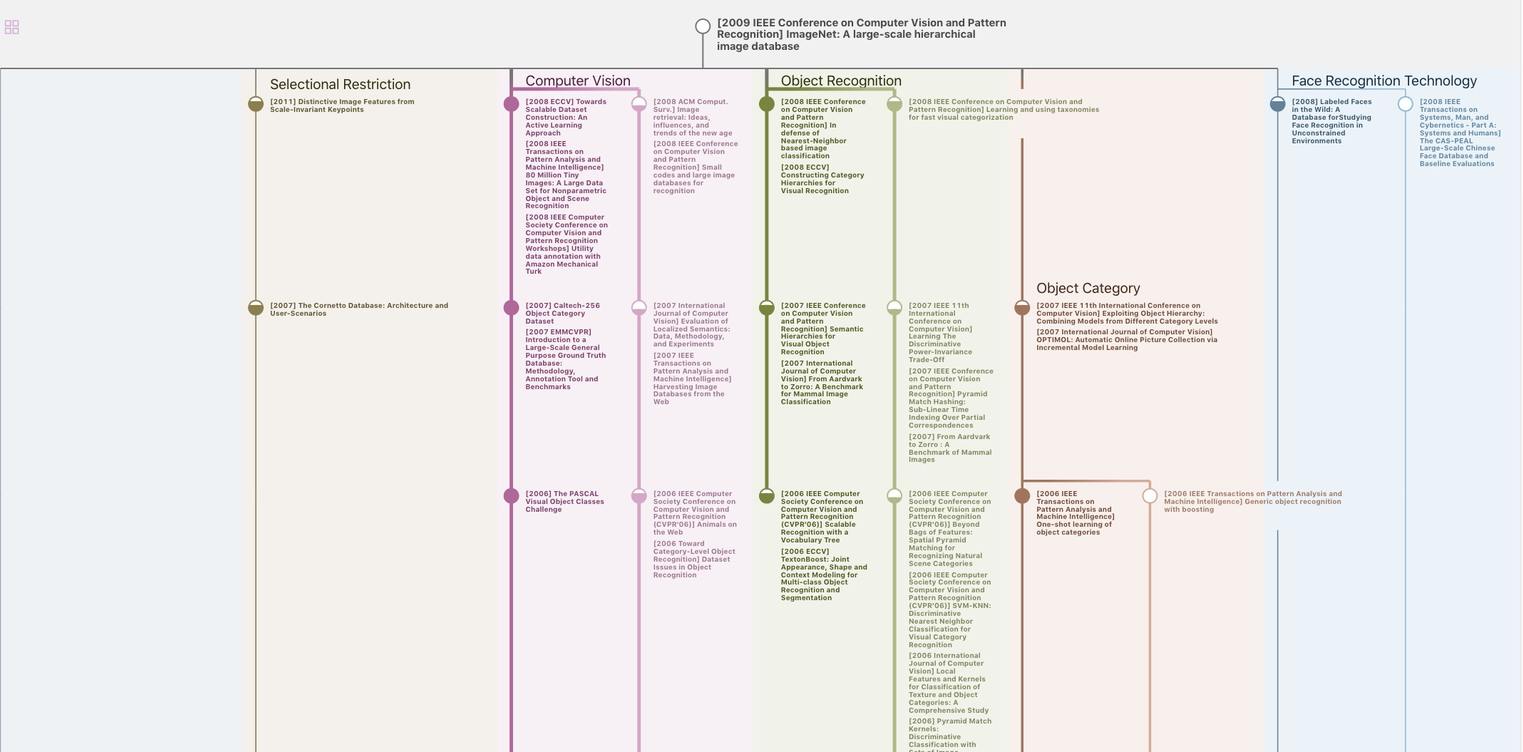
Generate MRT to find the research sequence of this paper
Chat Paper
Summary is being generated by the instructions you defined