Natural Language Processing And Recurrent Network Models For Identifying Genomic Mutation-Associated Cancer Treatment Change From Patient Progress Notes
JAMIA OPEN(2019)
摘要
Objectives: Natural language processing (NLP) and machine learning approaches were used to build classifiers to identify genomic-related treatment changes in the free-text visit progress notes of cancer patients.Methods: We obtained 5889 deidentified progress reports (2439 words on average) for 755 cancer patients who have undergone a clinical next generation sequencing (NGS) testing in Wake Forest Baptist Comprehensive Cancer Center for our data analyses. An NLP system was implemented to process the free-text data and extract NGS-related information. Three types of recurrent neural network (RNN) namely, gated recurrent unit, long short-term memory (LSTM), and bidirectional LSTM (LSTM_Bi) were applied to classify documents to the treatment-change and no-treatment-change groups. Further, we compared the performances of RNNs to 5 machine learning algorithms including Naive Bayes, K-nearest Neighbor, Support Vector Machine for classification, Random forest, and Logistic Regression.Results: Our results suggested that, overall, RNNs outperformed traditional machine learning algorithms, and LSTM_Bi showed the best performance among the RNNs in terms of accuracy, precision, recall, and F1 score. In addition, pre-trained word embedding can improve the accuracy of LSTM by 3.4% and reduce the training time by more than 60%.Discussion and Conclusion: NLP and RNN-based text mining solutions have demonstrated advantages in information retrieval and document classification tasks for unstructured clinical progress notes.
更多查看译文
关键词
machine learning, natural language processing, electronic health records, cancer, genomics
AI 理解论文
溯源树
样例
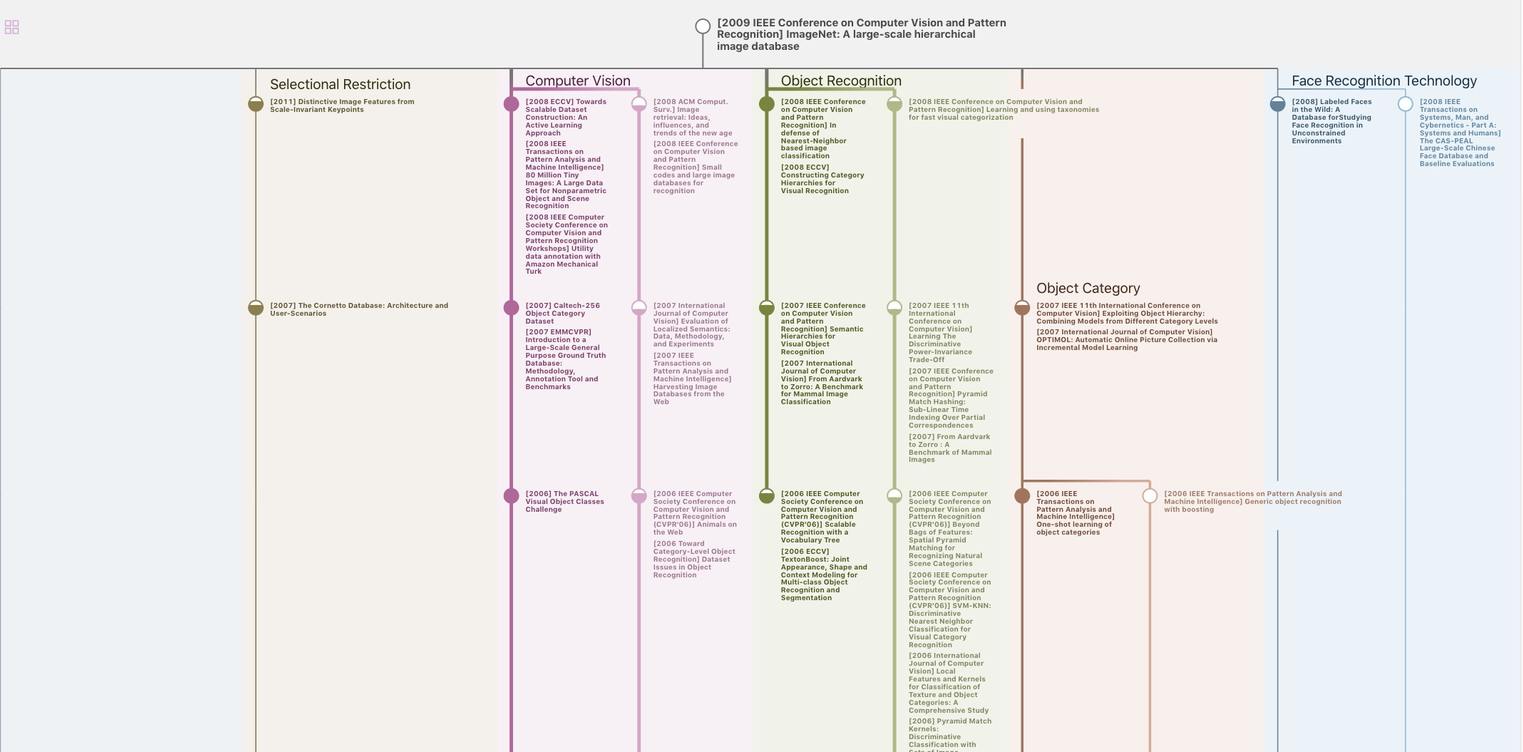
生成溯源树,研究论文发展脉络
Chat Paper
正在生成论文摘要