Data Disclosure Under Perfect Sample Privacy
IEEE TRANSACTIONS ON INFORMATION FORENSICS AND SECURITY(2020)
摘要
Perfect data privacy seems to be in fundamental opposition to the economical and scientific opportunities related to extensive data exchange. This paper defies this intuition by developing the principle of synergistic disclosure, in which collective properties of datasets are revealed without compromising the privacy of individual data samples. We study the properties of optimal strategies/mappings on finite as well as asymptotically large datasets, and discuss its fundamental limits defined as the synergistic disclosure capacity. Furthermore, we present explicit analytical expressions for the synergistic disclosure capacity of large datasets in various scenarios, and present cases in which our approach can disclose most of the information of interest. We finally discuss suboptimal schemes to provide sample privacy guarantees to large datasets at a reduced computational cost.
更多查看译文
关键词
Data disclosure, inference attacks, data privacy, latent features, perfect privacy
AI 理解论文
溯源树
样例
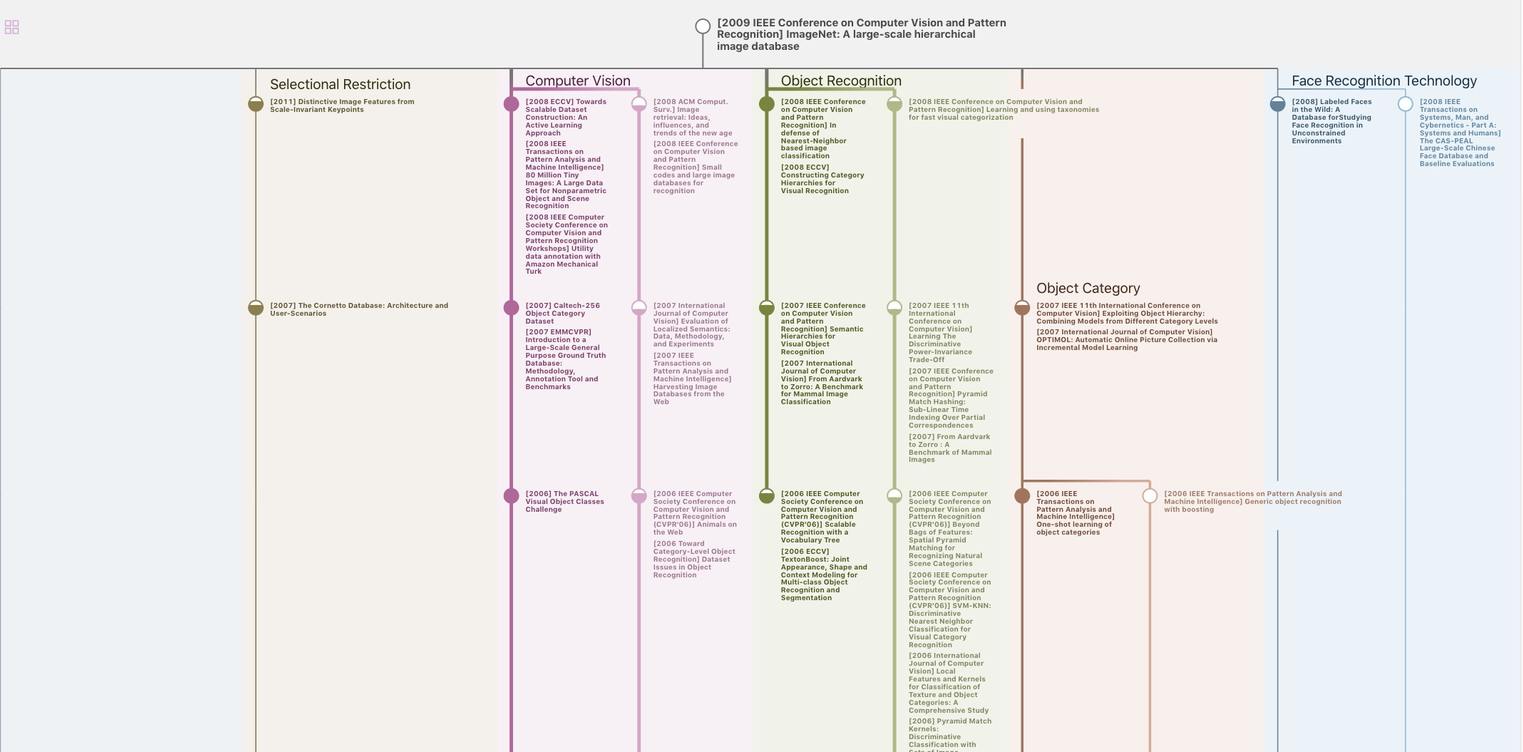
生成溯源树,研究论文发展脉络
Chat Paper
正在生成论文摘要