Environment-Aware Multi-Target Tracking Of Pedestrians
IEEE ROBOTICS AND AUTOMATION LETTERS(2019)
摘要
When navigating mobile robotic systems in dynamic environments, the ability to predict where pedestrians will move in the next few seconds is crucial. To tackle this problem, many solutions have been developed which take the environment's influence on human navigation behavior into account. However, few do this in a way which seamlessly generalizes to new environments where no prior observations of pedestrians are available. In this letter, we propose a novel method that uses convolutional neural networks (CNNs) to predict local statistics about the direction of likely human motion. Specifically, the network takes crops of a floor plan as an input and computes a cell-wise probability distributions over directions conditioned on the direction in the last time step. These environment-aware motion models can be used to improve the performance of existing tracking algorithms. We evaluated our approach with two different existing trackers, a discrete, grid-based filter as well as a particle filter and show that using our proposed method as motion model considerably improves prediction quality in previously unseen test environments. Even though we train the CNN entirely in simulation, our experiments suggest that the learned models generalize to real pedestrian data.
更多查看译文
关键词
Deep Learning in Robotics and Automation, Semantic Scene Understanding, Social Human-Robot Interaction
AI 理解论文
溯源树
样例
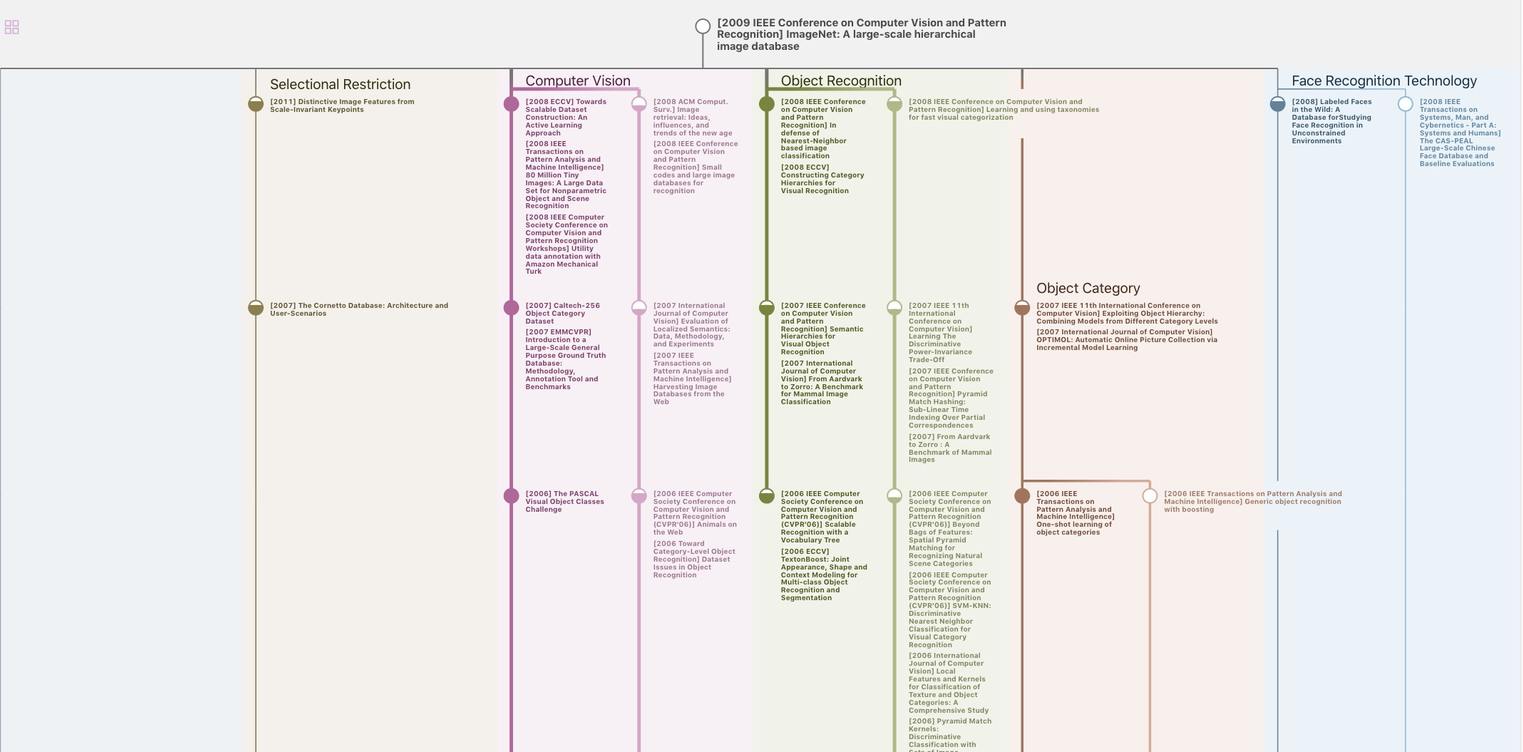
生成溯源树,研究论文发展脉络
Chat Paper
正在生成论文摘要