Learning Affective Correspondence Between Music And Image
2019 IEEE INTERNATIONAL CONFERENCE ON ACOUSTICS, SPEECH AND SIGNAL PROCESSING (ICASSP)(2019)
Abstract
We introduce the problem of learning affective correspondence between audio (music) and visual data (images). For this task, a music clip and an image are considered similar (having true correspondence) if they have similar emotion content. in order to estimate this crossmodal, emotion-centric similarity, we propose a deep neural network architecture that learns to project the data from the two modalities to a common representation space, and performs a binary classification task of predicting the affective correspondence (true or false). To facilitate the current study, we construct a large scale database containing more than 3,500 music clips and 85, 000 images with three emotion classes (positive, neutral, negative). The proposed approach achieves 61.67% accuracy for the affective correspondence prediction task on this database, outperforming two relevant and competitive baselines. We also demonstrate that our network learns modality-specific representations of emotion (without explicitly being trained with emotion labels), which are useful for emotion recognition in individual modalities.
MoreTranslated text
Key words
correspondence learning,crossmodal,deep learning,emotion recognition
AI Read Science
Must-Reading Tree
Example
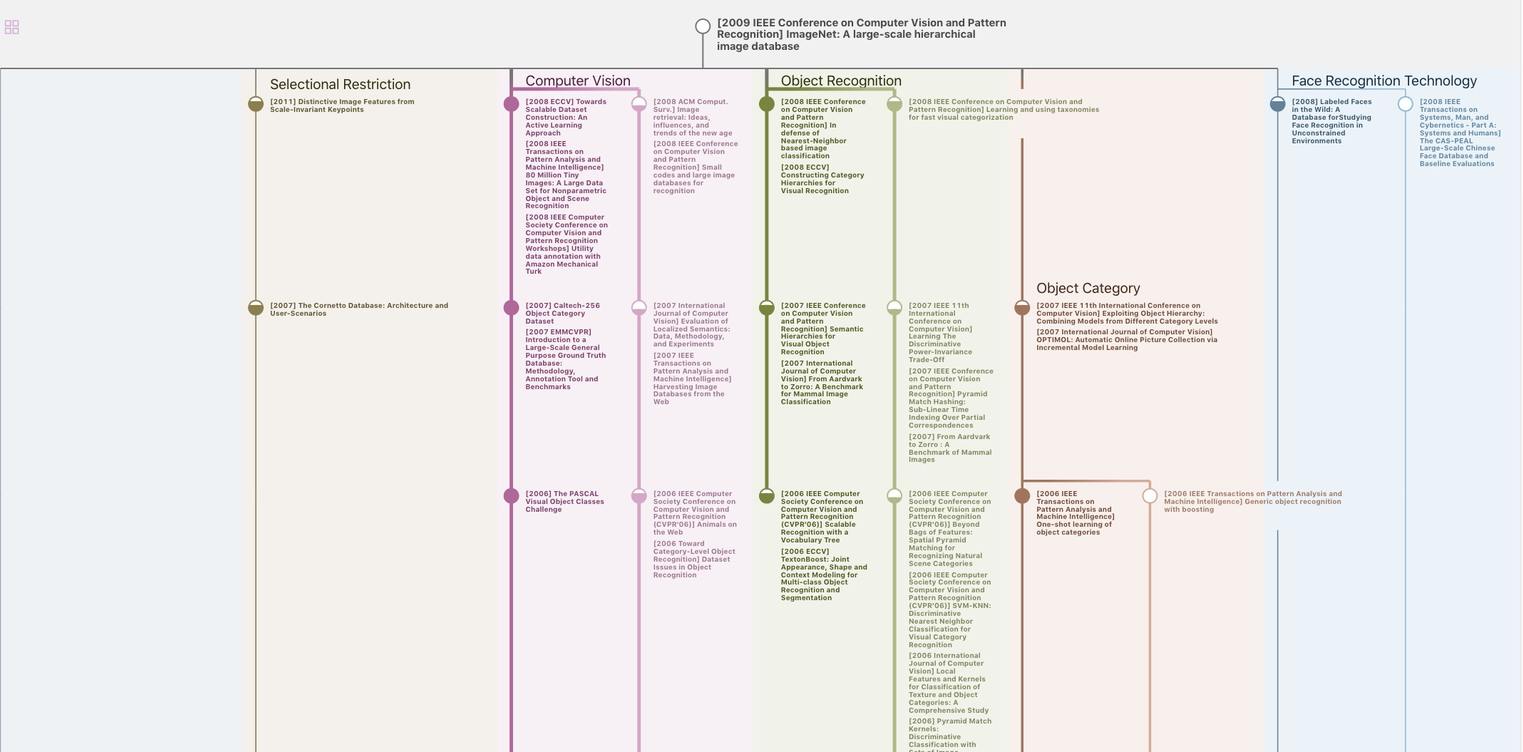
Generate MRT to find the research sequence of this paper
Chat Paper
Summary is being generated by the instructions you defined