Automatic generation of dense non-rigid optical flow
Computer Vision and Image Understanding(2021)
摘要
There hardly exists any large-scale datasets with dense optical flow of non-rigid motion from real-world imagery as of today. The reason lies mainly in the required setup to derive ground truth optical flows: a series of images with known camera poses along its trajectory, and an accurate 3D model from a textured scene. Human annotation is not only too tedious for large databases, it can simply hardly contribute to accurate optical flow. To circumvent the need for manual annotation, we propose a framework to automatically generate optical flow from real-world videos. The method extracts and matches objects from video frames to compute initial constraints, and applies a deformation over the objects of interest to obtain dense optical flow fields. We propose several ways to augment the optical flow variations. Extensive experimental results show that training on our automatically generated optical flow outperforms methods that are trained on rigid synthetic data using FlowNet-S, LiteFlowNet, PWC-Net, and RAFT. Datasets and implementation of our optical flow generation framework are released at https://github.com/lhoangan/arap_flow.
更多查看译文
关键词
non-rigi,optical flow,dataset,generation,as-rigid-as-possible
AI 理解论文
溯源树
样例
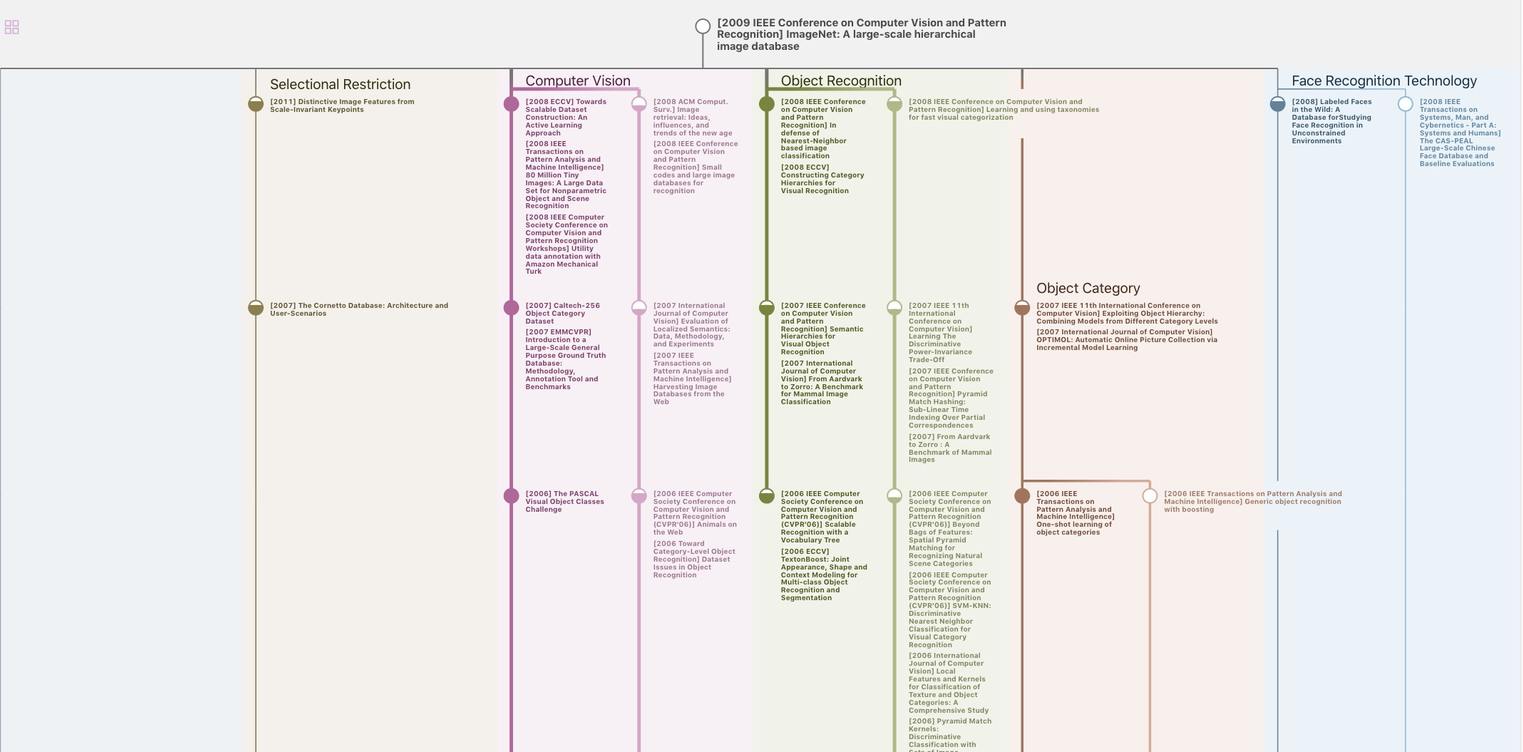
生成溯源树,研究论文发展脉络
Chat Paper
正在生成论文摘要