Approximate Spectral Gaps For Markov Chain Mixing Times In High Dimensions
SIAM Journal on Mathematics of Data Science(2021)
摘要
This paper introduces a concept of approximate spectral gap to analyze the mixing time of reversible Markov chain Monte Carlo (MCMC) algorithms for which the usual spectral gap is degenerate or almost degenerate. We use the idea to analyze an MCMC algorithm to sample from mixtures of densities. As an application we study the mixing time of a Gibbs sampler for variable selection in linear regression models. We show that, properly tuned, the algorithm has a mixing time that grows at most polynomially with the dimension. Our results also suggest that the mixing time improves when the posterior distribution contracts towards the true model and the initial distribution is well chosen.
更多查看译文
关键词
Markov chain Monte Carlo algorithms, Markov chain mixing times, spectral gap, MCMC for mixtures of densities, high-dimensional linear regression models
AI 理解论文
溯源树
样例
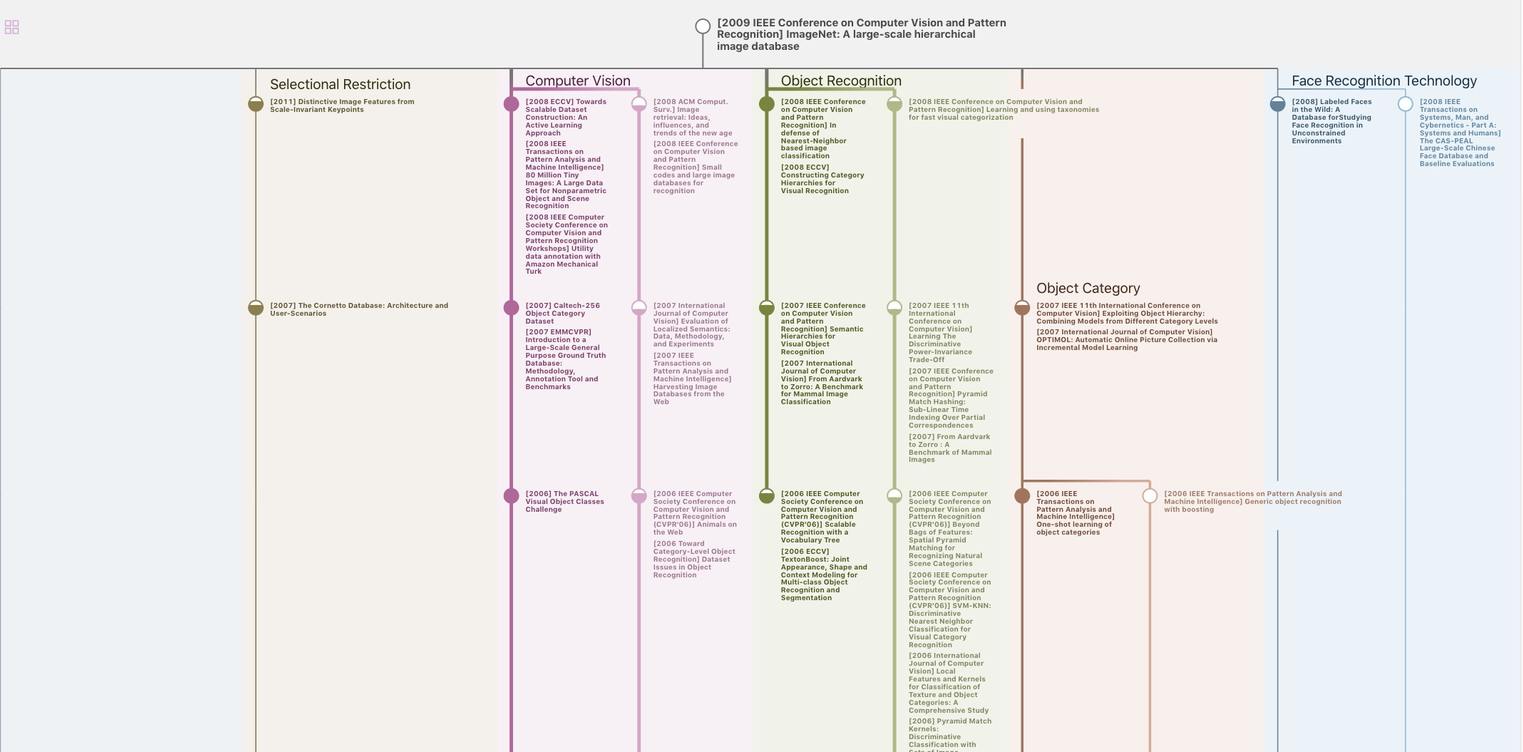
生成溯源树,研究论文发展脉络
Chat Paper
正在生成论文摘要