Conditional Dilated Convolution Attention Tracking Model
2019 Third IEEE International Conference on Robotic Computing (IRC)(2019)
摘要
Current commercial tracking systems do not process fast enough to perform in real-time. State-of-the-art (SOTA) methods use entire scenes to locate objects frame by frame and are commonly slowed by large convolutions. Alternatively, attention mechanisms track more efficiently by mimicking human optical cognitive interaction to only process small portions of an image. Thus, we took an attention approach to create a single model that learns to compare features along a sequence using dilated convolutions. Using popular data sets like Modified National Institute of Standards and Technology (MNIST) handwritten digits, we tested our work against previous attention based networks like Deep Recurrent Attentive Writer (DRAW) and Recurrent Attention Tracking Model (RATM) to compare tracking abilities. Here we present a novel Conditional Dilated Convolution Attention Network that builds on previous attention principles to achieve generic, efficient, and recurrent-less object tracking.
更多查看译文
关键词
Convolution,Target tracking,Data models,Mathematical model,Computational modeling,Kernel
AI 理解论文
溯源树
样例
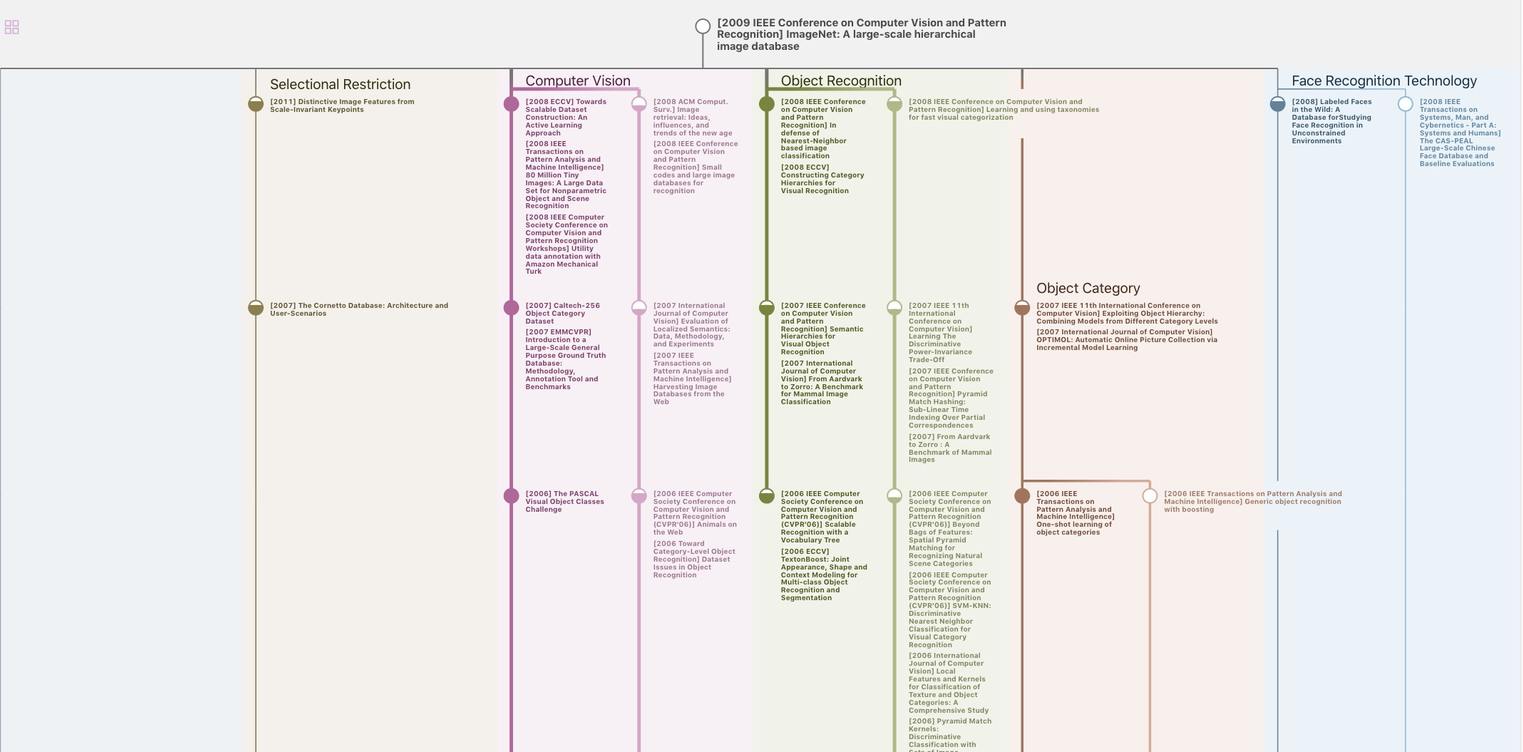
生成溯源树,研究论文发展脉络
Chat Paper
正在生成论文摘要